MetaReVision: Meta-Learning with Retrieval for Visually Grounded Compositional Concept Acquisition.
CoRR(2023)
Abstract
Humans have the ability to learn novel compositional concepts by recalling and generalizing primitive concepts acquired from past experiences. Inspired by this observation, in this paper, we propose MetaReVision, a retrieval-enhanced meta-learning model to address the visually grounded compositional concept learning problem. The proposed MetaReVision consists of a retrieval module and a meta-learning module which are designed to incorporate retrieved primitive concepts as a supporting set to meta-train vision-anguage models for grounded compositional concept recognition. Through meta-learning from episodes constructed by the retriever, MetaReVision learns a generic compositional representation that can be fast updated to recognize novel compositional concepts. We create CompCOCO and CompFlickr to benchmark the grounded compositional concept learning. Our experimental results show that MetaReVision outperforms other competitive baselines and the retrieval module plays an important role in this compositional learning process.
MoreTranslated text
Key words
metarevision meta-learning,retrieval,concept
AI Read Science
Must-Reading Tree
Example
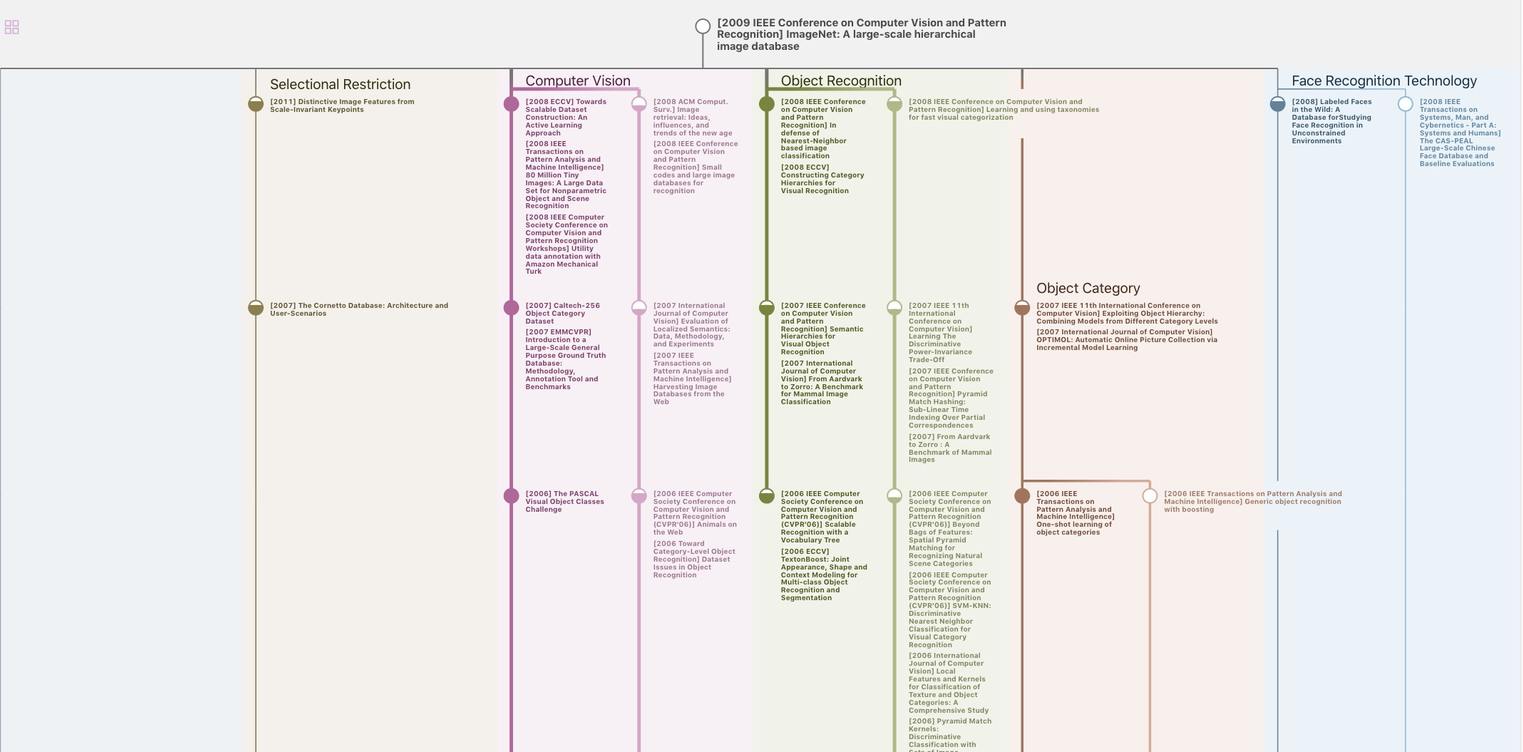
Generate MRT to find the research sequence of this paper
Chat Paper
Summary is being generated by the instructions you defined