MIEP: Channel Pruning with Multi-granular Importance Estimation for Object Detection
MM '23: Proceedings of the 31st ACM International Conference on Multimedia(2023)
摘要
This paper investigates compressing a pre-trained deep object detector to a lightweight one by channel pruning, which has proved effective and flexible in promoting efficiency. However, the majority of existing works trim channels based on a monotonous criterion for general purposes, i.e., the importance to the task-specific loss. They are prone to overly prune intermediate layers and simultaneously leave large intra-layer redundancy, severely deteriorating the detection accuracy. To address the issues above, we propose a novel channel pruning approach with multi-granular importance estimation (MIEP), consisting of the Feature-level Object-sensitive Importance (FOI) and the Intra-layer Redundancy-aware Importance (IRI). The former puts large weights on channels that are critical for object representation through the guidance of object features from the pre-trained model, and mitigates over-pruning when combined with the task-specific loss. The latter groups highly correlated channels based on clustering, which are subsequently pruned with priority to decrease redundancy. Extensive experiments on the COCO and VOC benchmarks demonstrate that MIEP remarkably outperforms the state-of-the-art channel pruning approaches, achieves a better balance between accuracy and efficiency compared to lightweight object detectors, and generalizes well to various detection frameworks (e.g., Faster-RCNN and FSAF) and tasks (e.g., classification).
更多查看译文
AI 理解论文
溯源树
样例
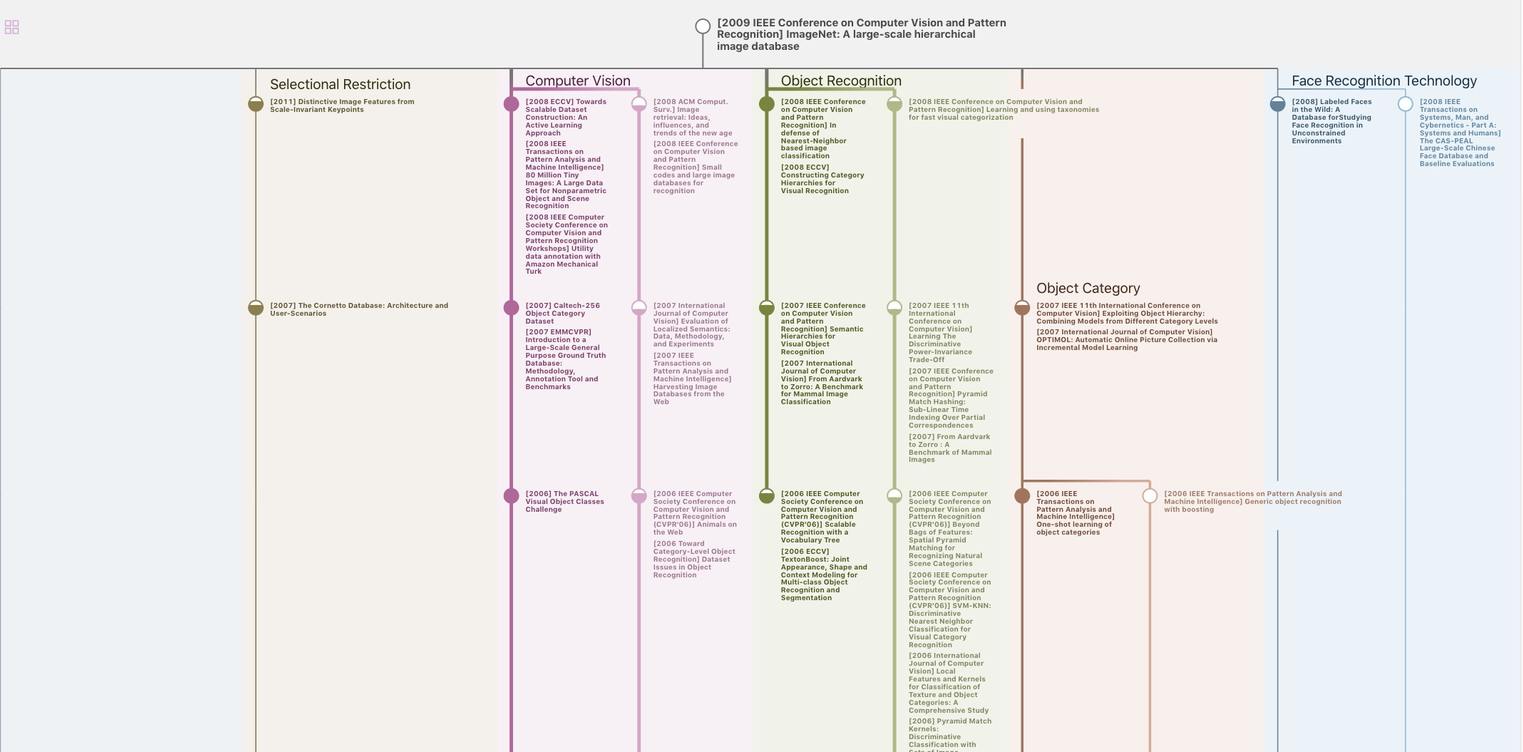
生成溯源树,研究论文发展脉络
Chat Paper
正在生成论文摘要