Spatial-angular Quality-aware Representation Learning for Blind Light Field Image Quality Assessment
PROCEEDINGS OF THE 31ST ACM INTERNATIONAL CONFERENCE ON MULTIMEDIA, MM 2023(2023)
关键词
Blind light field image quality assessment,self-supervised learning,spatial-angular quality-aware representations,FSFNet
AI 理解论文
溯源树
样例
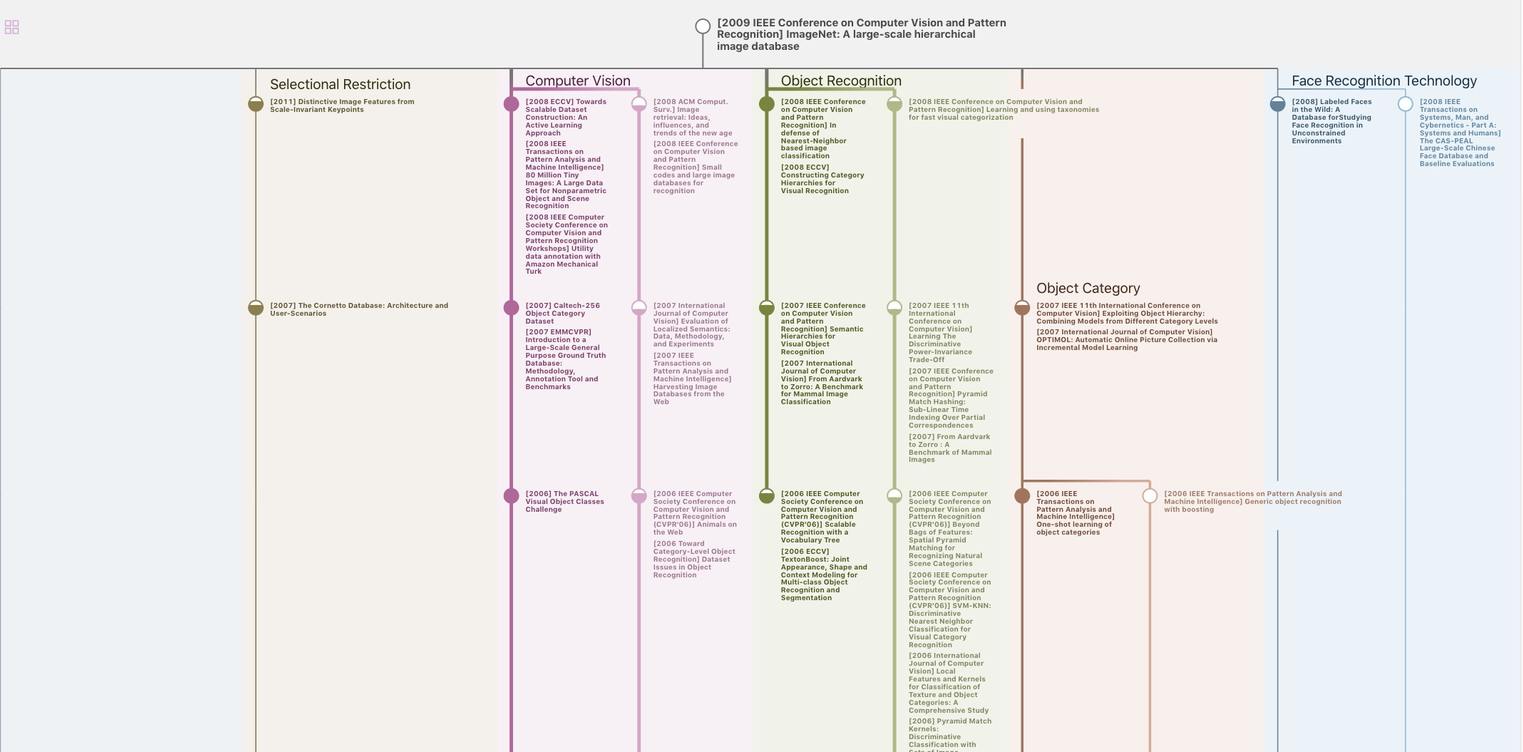
生成溯源树,研究论文发展脉络
Chat Paper
正在生成论文摘要