Hierarchical Category-Enhanced Prototype Learning for Imbalanced Temporal Recommendation
MM '23: Proceedings of the 31st ACM International Conference on Multimedia(2023)
摘要
Temporal recommendation systems aim to suggest items to users at the optimal time. However, the significant imbalance of items in the training data poses a major challenge to predictive accuracy. Existing approaches attempt to alleviate this issue by modifying the loss function or utilizing resampling techniques, but such approaches may inadvertently amplify the specificity of certain behaviors. To address this problem, we propose a novel temporal recommendation algorithm called HCRec. The algorithm adopts a transformer-like architecture, focuses on decoupling variant and invariant information according to hierarchical categories, and then uses invariant prototypes to mitigate the impact of special behaviors. Although category-enhanced recommendation is not a new concept, current approaches have considerable limitations. They usually incorporate the category information into a black-box model as another input sequence, which can easily lead to overfitting. In contrast, HCRec creates a hierarchical relationship tree of items and categories, and establishes mapping rules to classify nodes as variant or invariant. Then, an attention model is used to extract potential variant node features from historical behaviors and enhance them with external knowledge. The invariant node features are fused based on the relationship tree, and finally used for recommendation. On top of this, two time kernel functions are designed to simulate short-term and long-period time effects. Experiments on real-world datasets show that hierarchical category-enhanced prototype learning helps alleviate the imbalance problem, enabling HCRec to outperform all baseline methods.
更多查看译文
AI 理解论文
溯源树
样例
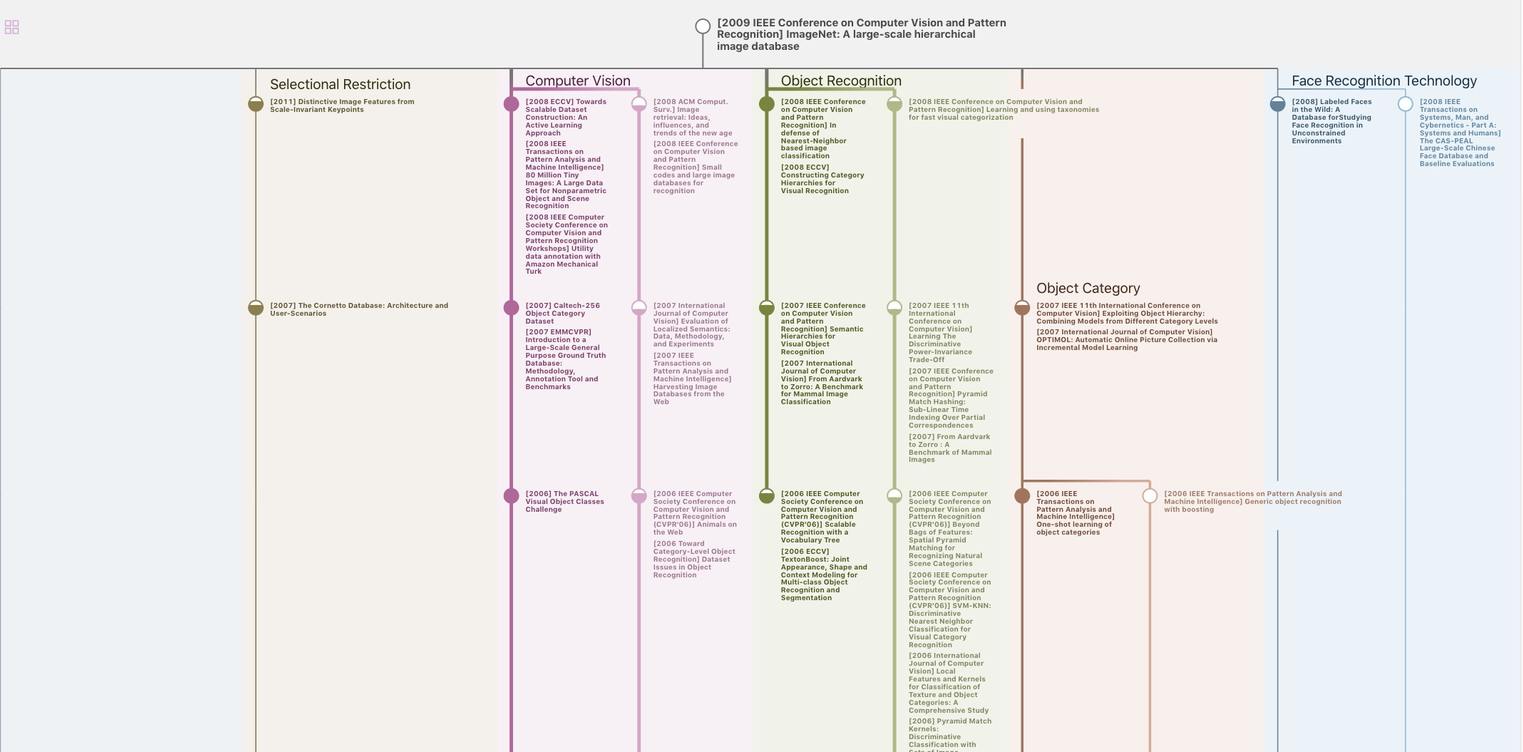
生成溯源树,研究论文发展脉络
Chat Paper
正在生成论文摘要