Legate Sparse: Distributed Sparse Computing in Python
SC '23: Proceedings of the International Conference for High Performance Computing, Networking, Storage and Analysis(2023)
摘要
The sparse module of the popular SciPy Python library is widely used across applications in scientific computing, data analysis and machine learning. The standard implementation of SciPy is restricted to a single CPU and cannot take advantage of modern distributed and accelerated computing resources. We introduce Legate Sparse, a system that transparently distributes and accelerates unmodified sparse matrix-based SciPy programs across clusters of CPUs and GPUs, and composes with cuNumeric, a distributed NumPy library. Legate Sparse uses a combination of static and dynamic techniques to efficiently compose independently written sparse and dense array programming libraries, providing a unified Python interface for distributed sparse and dense array computations. We show that Legate Sparse is competitive with single-GPU libraries like CuPy and achieves 65% of the performance of PETSc on up to 1280 CPU cores and 192 GPUs of the Summit supercomputer, while offering the productivity benefits of idiomatic SciPy and NumPy.
更多查看译文
关键词
Machine Learning,Sparsity,Computer Science,Dense Array,Single CPU,Parallelization,Sparse Data,Sparse Matrix,Load Data,Regional Mapping,Single Node,Data Partitioning,Communication Patterns,Nonzero Entries,Linear Algebra,Sparse Structure,Single GPU,Standard Representation,Constraint Satisfaction Problem,Quantum Simulation,External Libraries,Sparse Format,All-to-all Communication,Destination Regions,Domain-specific Languages,Multigrid Method,Data Structure,Partitioning Scheme,Mapping Strategy,Memory Usage
AI 理解论文
溯源树
样例
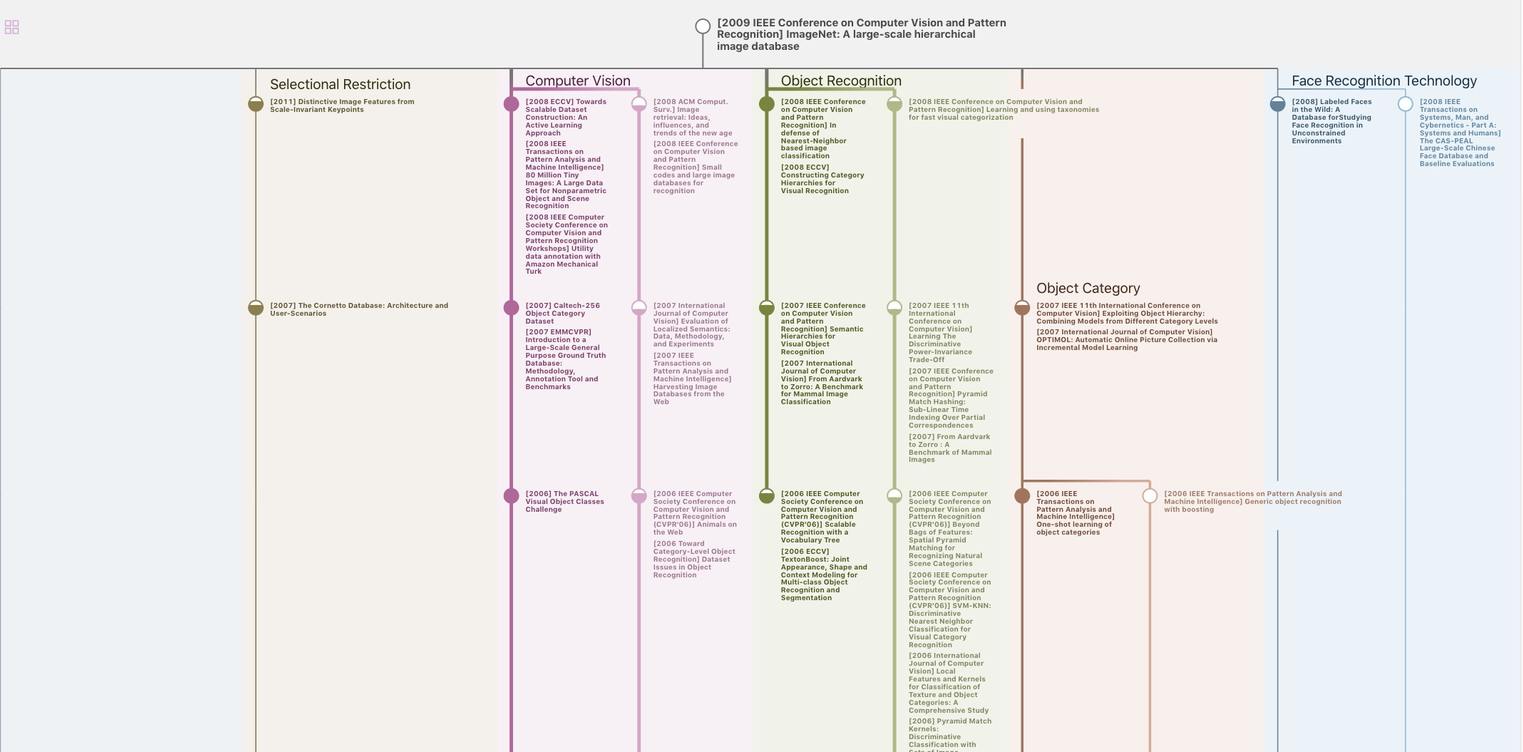
生成溯源树,研究论文发展脉络
Chat Paper
正在生成论文摘要