Deep reinforcement learning based voltage control revisited
IET Generation, Transmission & Distribution(2023)
摘要
Abstract Deep Reinforcement Learning (DRL) has shown promise for voltage control in power systems due to its speed and model‐free nature. However, learning optimal control policies through trial and error on a real grid is infeasible due to the mission‐critical nature of power systems. Instead, DRL agents are typically trained on a simulator, which may not accurately represent the real grid. This discrepancy can lead to suboptimal control policies and raises concerns for power system operators. In this paper, we revisit the problem of RL‐based voltage control and investigate how model inaccuracies affect the performance of the DRL agent. Extensive numerical experiments are conducted to quantify the impact of model inaccuracies on learning outcomes. Specifically, techniques that enable the DRL agent are focused on learning robust policies that can still perform well in the presence of model errors. Furthermore, the impact of the agent's decisions on the overall system loss are analyzed to provide additional insight into the control problem. This work aims to address the concerns of power system operators and make DRL‐based voltage control more practical and reliable.
更多查看译文
关键词
learning (artificial intelligence), power system control, power system security, voltage control
AI 理解论文
溯源树
样例
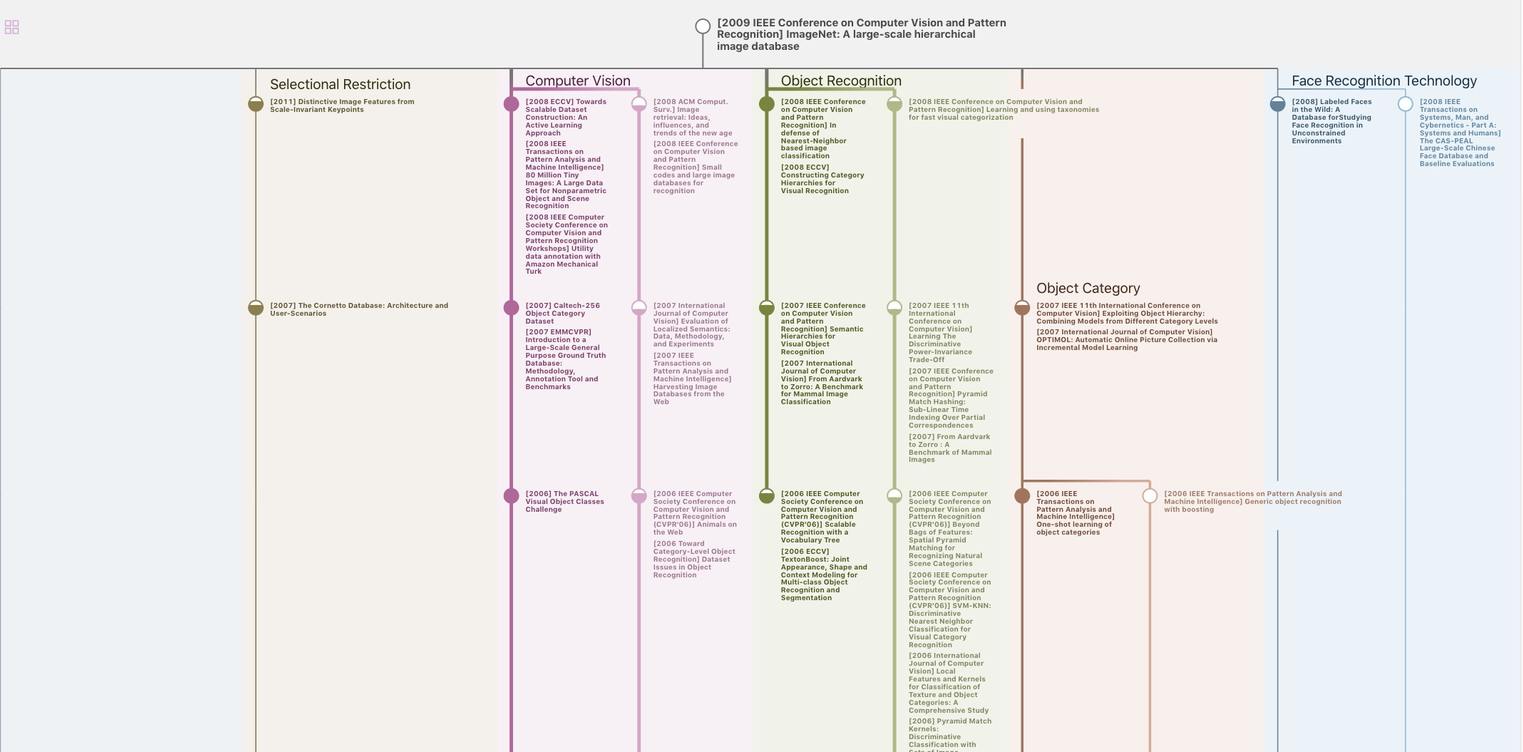
生成溯源树,研究论文发展脉络
Chat Paper
正在生成论文摘要