Semi-Supervised Convolutional Vision Transformer with Bi-Level Uncertainty Estimation for Medical Image Segmentation
MM '23: Proceedings of the 31st ACM International Conference on Multimedia(2023)
摘要
Semi-supervised learning (SSL) has attracted much attention in the field of medical image segmentation, which enables to alleviate the heavy burden of labelling pixel-wise annotation by extracting knowledge from unlabeled data. The existing methods basically benefit from the success of convolutional neural networks (CNNs) by keeping consistency of the predictions under small perturbations imposed on the networks or inputs. Two main concerns arise when learning such a paradigm: (1) CNNs tend to retain discriminative local features, neglecting global dependency and thus leading to inaccurate localization; (2) CNNs omit reliable feature-level and pixel-level information, resulting in sketchy pseudo-labels, especially around the confusing boundary. In this paper, we revisit the model of semi-supervised learning and develop a novel CNN-Transformer learning framework that allows for effective segmentation of medical images by producing complementary and reliable features and pseudo-label with bi-level uncertainty. Motivated by the uncertainty estimation to gain insight on feature discrimination, we explore the statistical and geometrical properties of features on network optimization and thus launching an alignment method in a more accurate and stable way. We attach equal significance to pixel-level uncertainty estimation for alleviating the influence of unreliable pseudo-labels in the training progress and advocating the reliability of predictions. Experimental results show that our method significantly surpasses existing semi-supervised approaches on two public medical image segmentation datasets.
更多查看译文
AI 理解论文
溯源树
样例
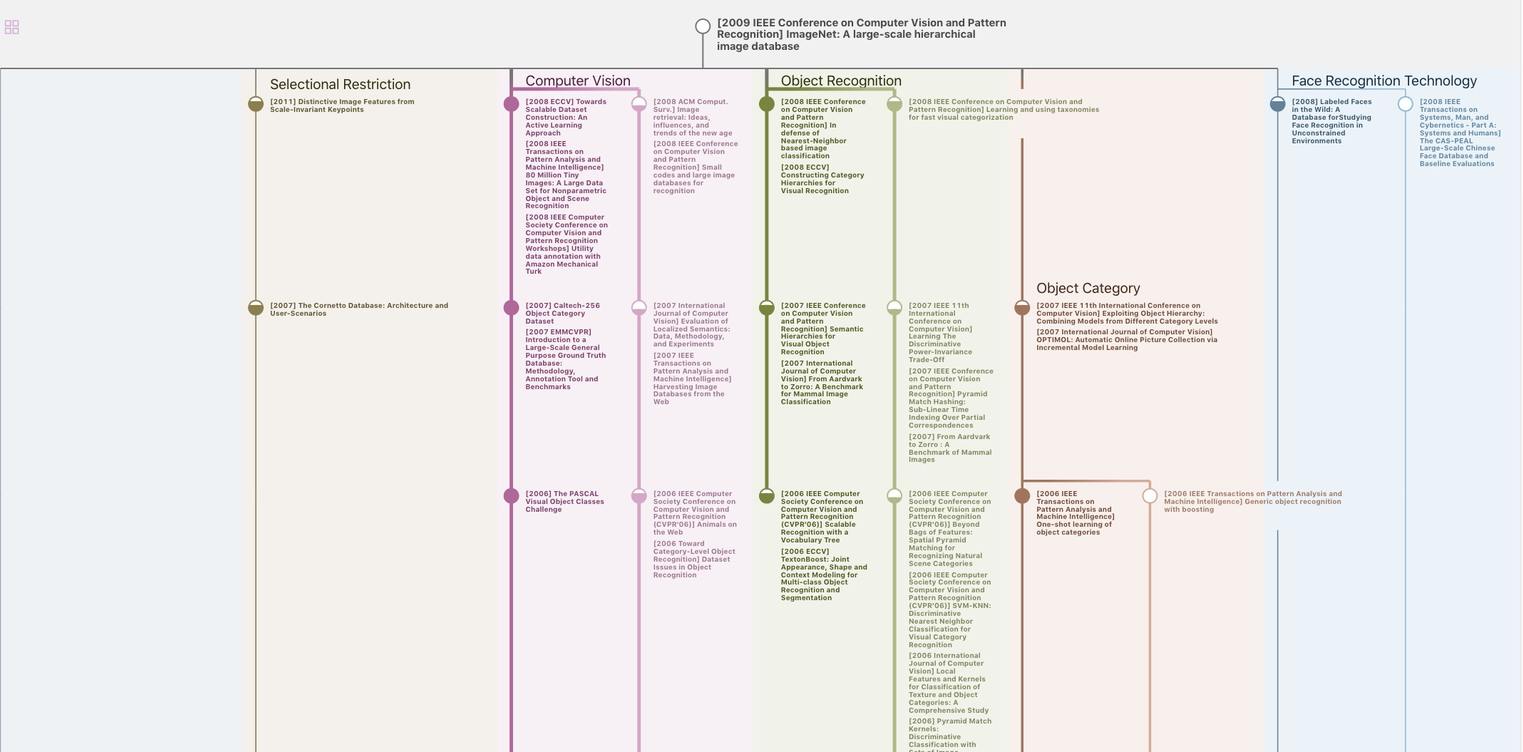
生成溯源树,研究论文发展脉络
Chat Paper
正在生成论文摘要