Factorized Omnidirectional Representation based Vision GNN for Anisotropic 3D Multimodal MR Image Segmentation
MM '23: Proceedings of the 31st ACM International Conference on Multimedia(2023)
摘要
Anisotropy arises due to the influence of scanning equipment and parameters, resulting in a distance between slices that is often much greater than the actual distance represented by a single pixel within each slice. This can lead to inefficiency or ineffectiveness in 3D convolution. To address the anisotropy issue, we propose FOrViG, an asymmetric vision graph neural network (GNN) framework that captures the correlation between different slices by constructing a graph for multi-slice images and aggregating information from adjacent nodes. This allows FOrViG to efficiently extract 3D spatial scale information, and effectively identify feature nodes associated with small lesions efficiently, thereby improving the accuracy of lesion segmentation on anisotropic 3D multimodal MR images. As far as we know, this is the first study that adopts GNN to address anisotropy issues. Additionally, we also design a factorized omnidirectional representation method and a supervised multi-perspective contrastive learning strategy to enhance the capability of FOrViG in learning multi-scale omnidirectional presentation information, graphics construction, and distinguishing foreground from background. Extensive experiments on the PI-CAI dataset demonstrate that FOrViG significantly outperforms several state-of-the-art 3D segmentation algorithms.
更多查看译文
AI 理解论文
溯源树
样例
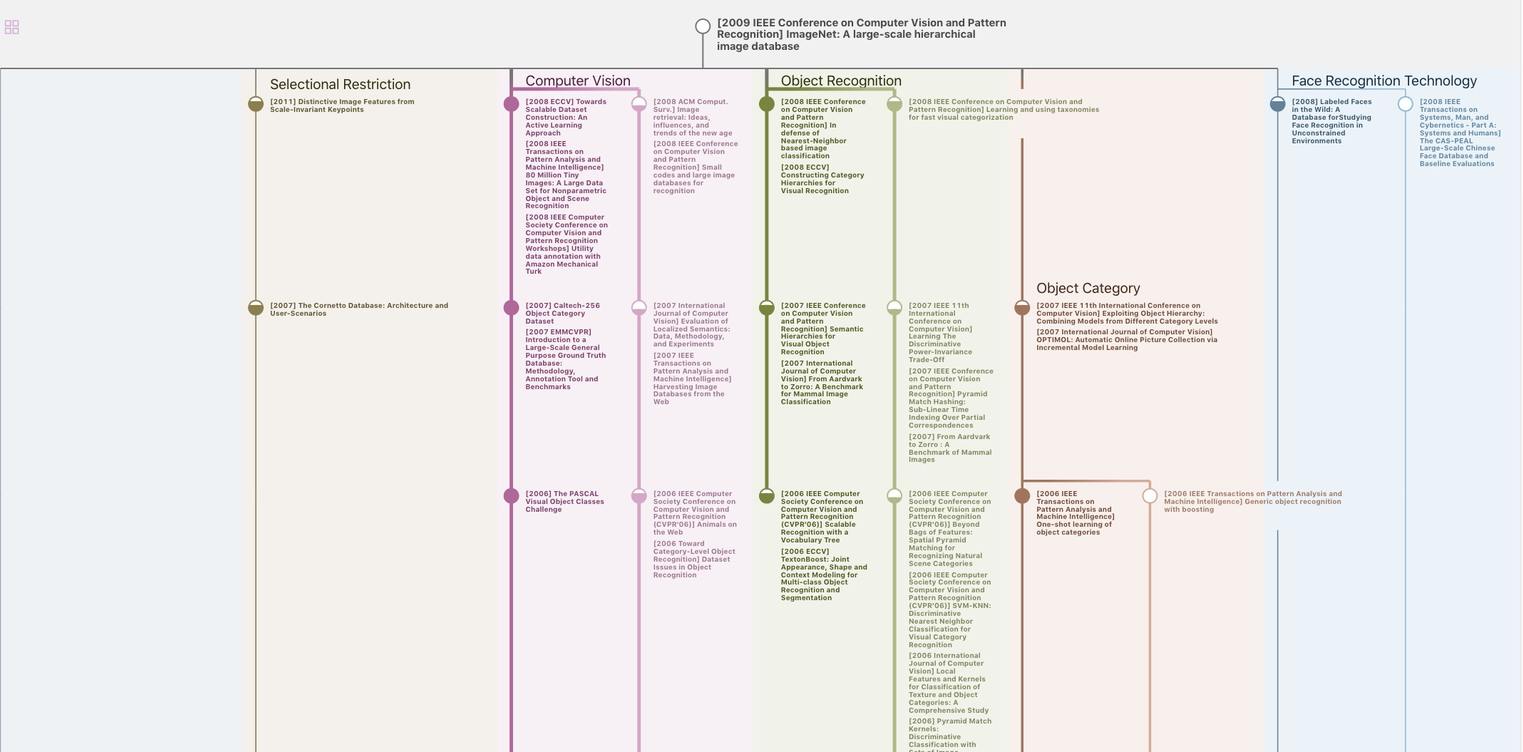
生成溯源树,研究论文发展脉络
Chat Paper
正在生成论文摘要