FedCE: Personalized Federated Learning Method based on Clustering Ensembles
MM '23: Proceedings of the 31st ACM International Conference on Multimedia(2023)
摘要
Federated learning (FL) is a privacy-aware computing framework that enables multiple clients to collaborate in solving machine learning problems. In real scenarios, non-IID data held by different edge devices will degrade the performance of global FL models. To address this issue, most FL methods utilize cluster algorithms to group clients with similar distributions. However, these methods do not fully utilize the distribution features of client data, resulting in a lack of generalization in the cluster model. In order to make the cluster more suitable for the distribution features of user data, we propose a clustering-ensemble based federated learning method (FedCE) that sets each client associated with multiple clusters. We extract the features of client distributions to quantify the relationship between clients and clusters, and optimize the local model of clients through the historical performance of the cluster model. Furthermore, we dynamically estimate the number of clusters each client belongs to through the diversity of client performance. We conduct experiments on scenarios with mixture two-distributions, three-distributions and Dirichlet-distributions. The results show that the FedCE algorithm has better performance than the state-of-the-art clustered FL methods in both cluster and client models under different data distributions.
更多查看译文
AI 理解论文
溯源树
样例
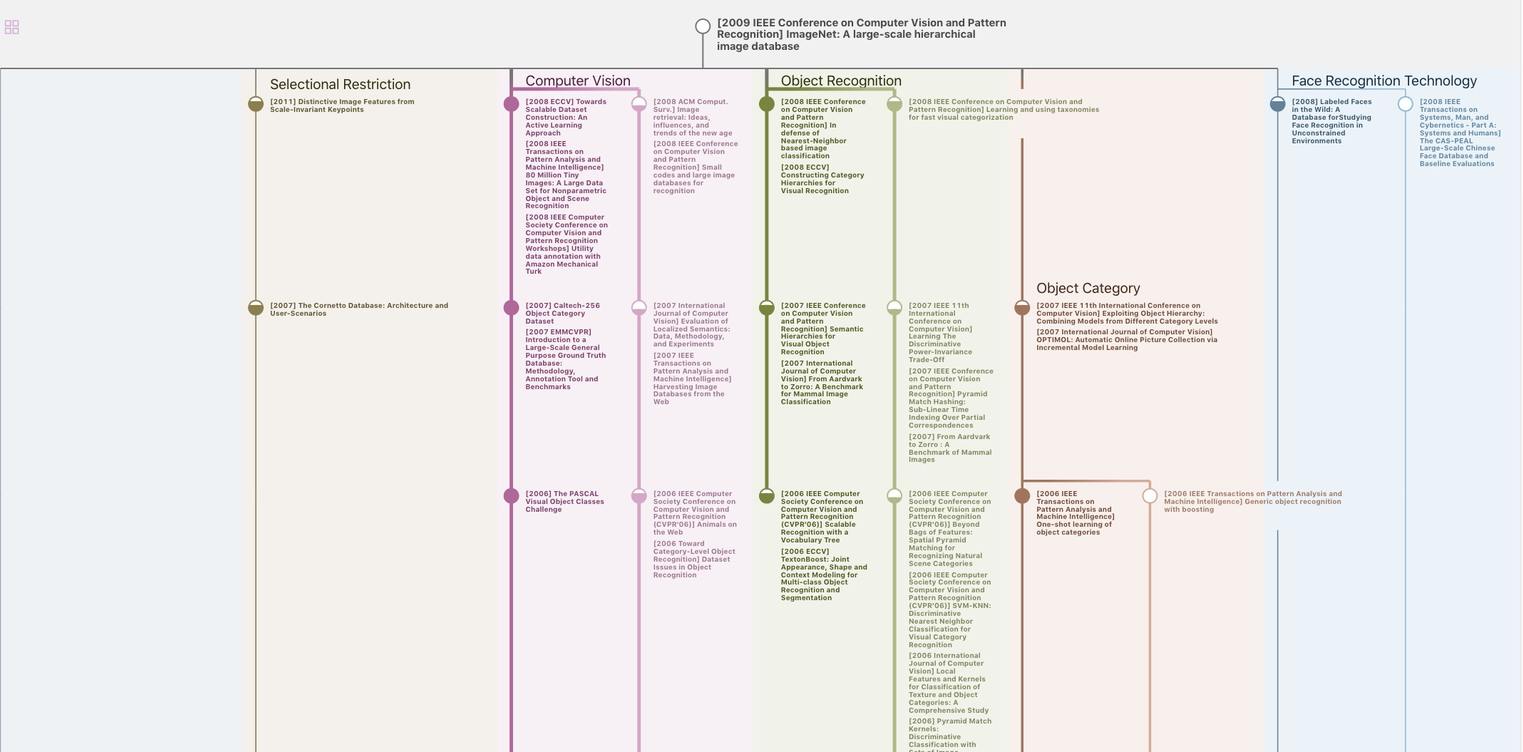
生成溯源树,研究论文发展脉络
Chat Paper
正在生成论文摘要