Learning to complement: Relation complementation network for few-shot class-incremental learning
Knowledge-Based Systems(2023)
摘要
Real-world industrial scenarios pose a challenging task known as few-shot class-incremental learning (FSCIL), which aims to recognize new classes using a few samples while not forgetting the old classes. Despite the recent advance of FSCIL, most existing methods rely on a single metric for making incremental relation predictions, which is unilateral and lacks stability. In this paper, we remedy this issue from two aspects. Specifically, to make convincing relation predictions, we first propose a relation complementation strategy that aggregates different metric models to investigate the comprehensive relation of classifier weights and test features. Then, to make the proposed strategy well fit the incremental scenarios, we design a pseudo incremental relation complementation learning scheme that constructs the learning tasks by mimicking the data setting in real incremental sessions. Taken together, our proposed method dubbed Relation Complementation Network (RCN) achieves the state-of-the-art performance on miniImageNet, CIFAR100 and CUB200. Our code is available at https://github.com/YeZiLaiXi/KT-RCN.git.
更多查看译文
关键词
Lifelong learning,Class-incremental learning,Few-shot class-incremental learning,Image recognition
AI 理解论文
溯源树
样例
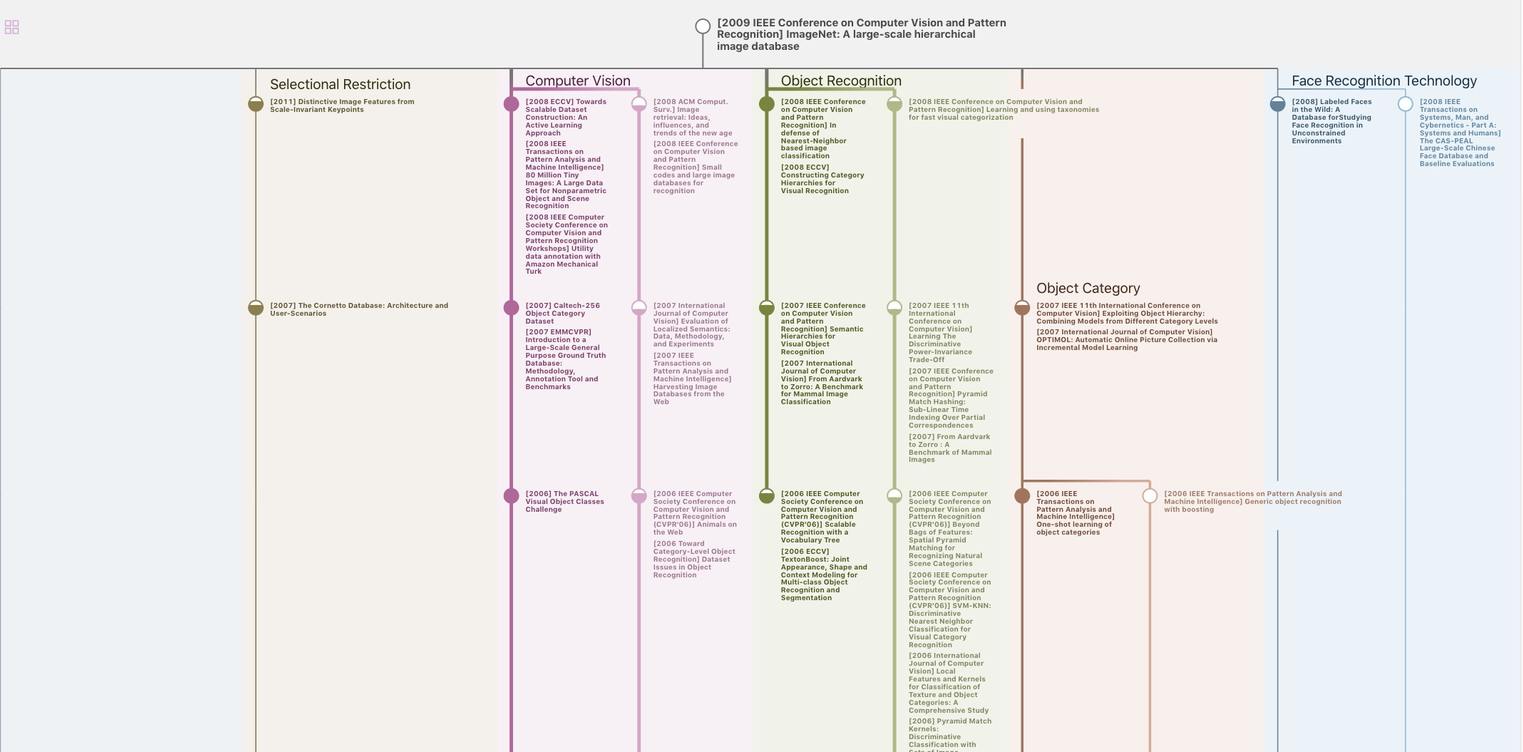
生成溯源树,研究论文发展脉络
Chat Paper
正在生成论文摘要