Resource and Bandwidth-Aware Video Analytics with Adaptive Offloading
2023 IEEE 20th International Conference on Mobile Ad Hoc and Smart Systems (MASS)(2023)
摘要
To enable computation-intensive video analytics, streaming video data and offloading computation from the source to the inference server running deep neural networks has now become a mainstream approach. Existing works adapt to either computing or network resources, and fail to consider the adaptation for both computation and network resources, which is a practical issue in real-world scenarios. To this end, we propose a video analytics pipeline that jointly considers the computing resource on both sides as well as the available bandwidth to achieve high inference accuracy with guaranteed inference latency and alleviate server-side workloads. We adopt model partitioning to allocate the computation on both sides and a hybrid compression method to adjust the data transmission. We further propose an offloading adaptation algorithm to make proper decisions on the partitioning point and the compression ratio to achieve good performance under limited source-side capabilities and varying bandwidth. Evaluation results show that our resource and bandwidth-aware adaptive offloading approach can maintain almost lossless inference accuracy with low latency while reducing server-side resource usage by up to 30% compared to the baseline approaches.
更多查看译文
关键词
video analytics,deep neural networks,model partitioning,adaptive offloading
AI 理解论文
溯源树
样例
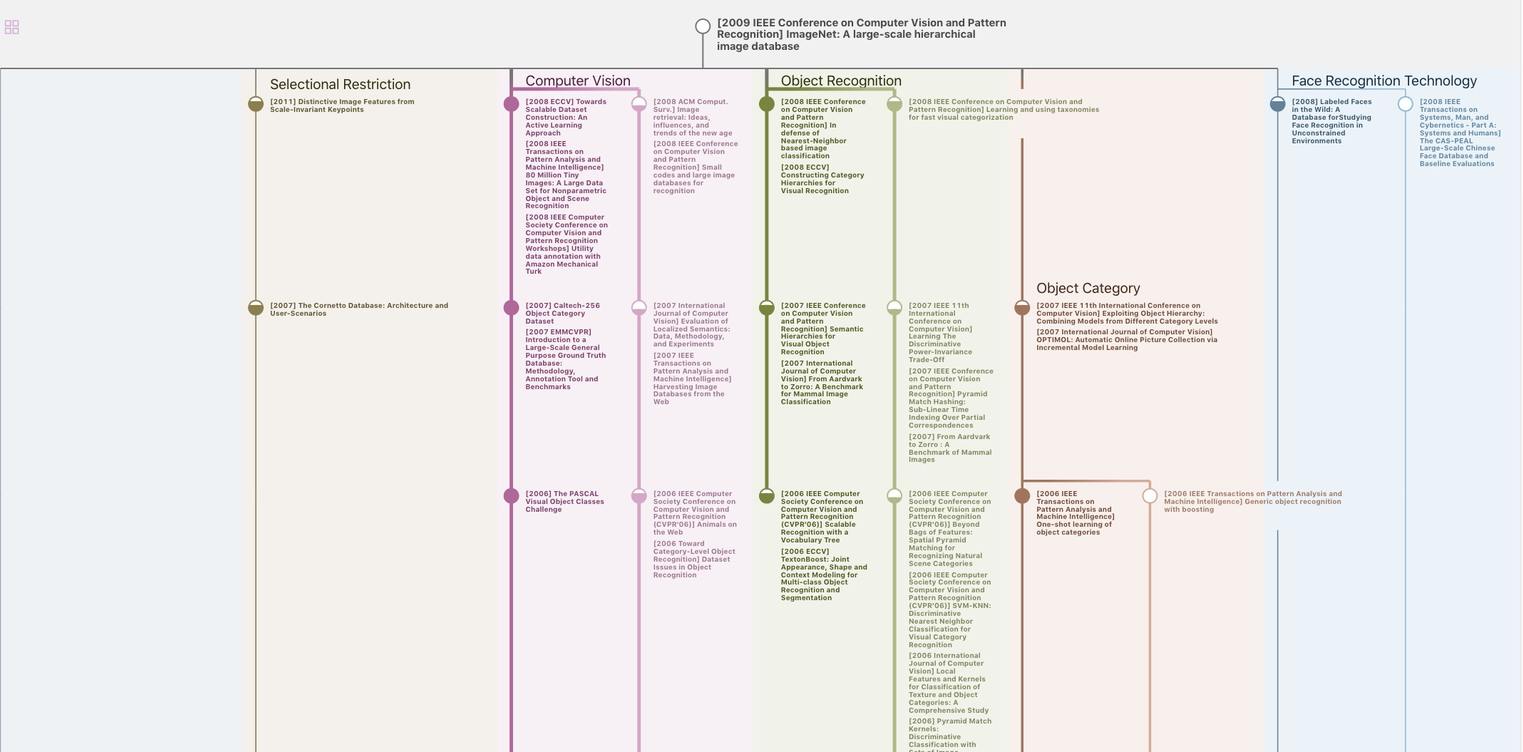
生成溯源树,研究论文发展脉络
Chat Paper
正在生成论文摘要