Comparative Analysis of Surrogate Models for High-Dimensional Expensive Problems
2023 5th International Conference on Data-driven Optimization of Complex Systems (DOCS)(2023)
摘要
To resolve the challenges posed by evaluating approximated fitness values in high-dimensional expensive optimization problems. This work primary focus on exploring the theoretical and structural aspects of four distinct surrogate models. The models under comparison are radial basis function, Broad learning system, random vector functional link neural networks, and extreme learning machines. The primary focus of the investigation encompassed the comprehensive examination of six distinct test functions, applied to both 100-D and 50-D benchmark problems. Throughout the comparison, meticulous attention was directed towards two pivotal facets: accuracy and computational cost. On the other hand, the combination of the approximated fitness values and the approximated uncertainty is regarded as two distinct objectives.The study's results revealed notable differences in performance among the examined models. Radial basis function and random vector functional link neural networks exhibited superior accuracy and scalability compared to the Broad learning system and extreme learning machines. Among these, the random vector functional link neural networks show-case the best performance across all six test functions in the 50-D benchmark problems. The implications of these findings are valuable for selecting appropriate fitness estimation models in the context of High-dimensional Expensive optimization problems.
更多查看译文
关键词
radial basis function,broad Learning System,random vector functional link neural networks,Expensive optimization
AI 理解论文
溯源树
样例
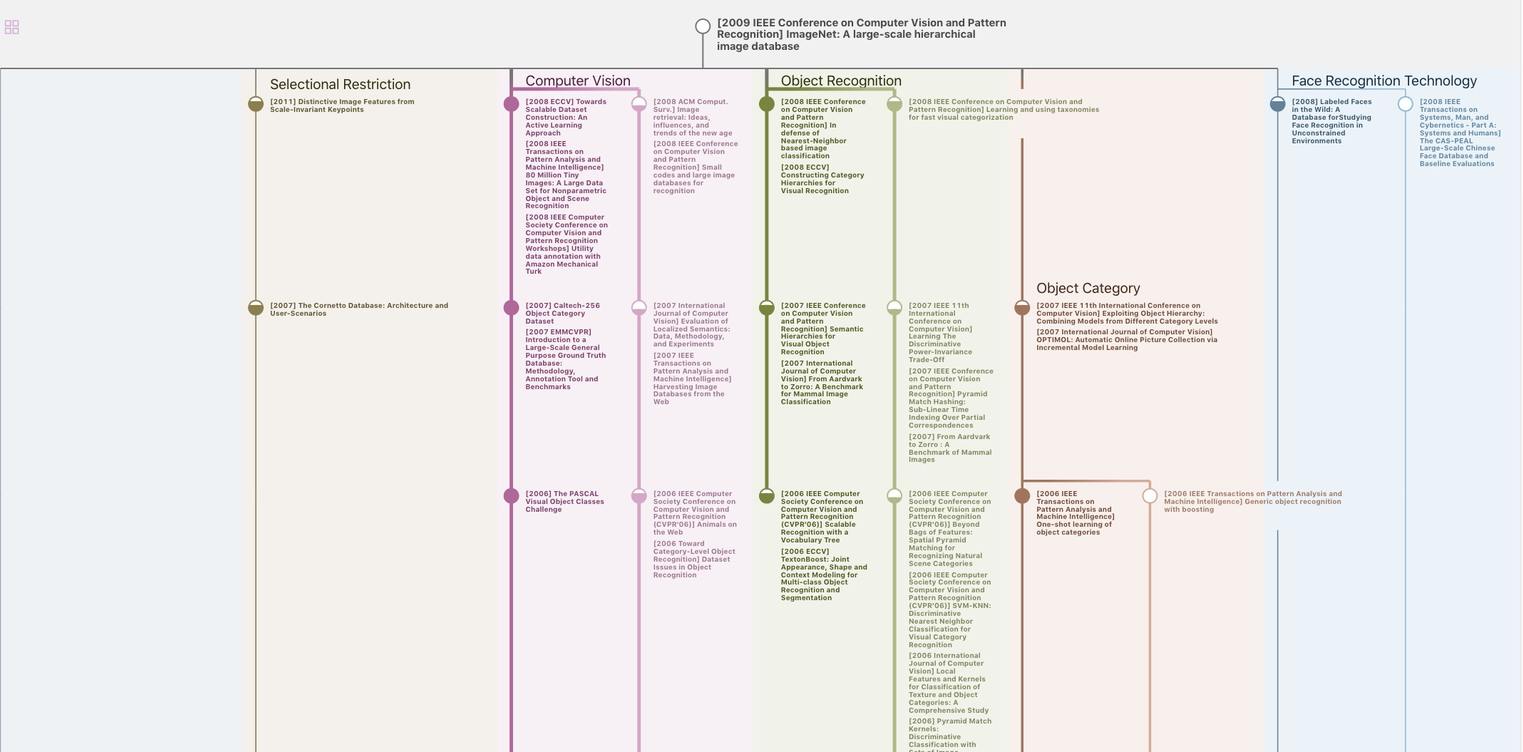
生成溯源树,研究论文发展脉络
Chat Paper
正在生成论文摘要