Explored seeds generation for weakly supervised semantic segmentation
Neural Computing and Applications(2024)
摘要
Weakly supervised semantic segmentation with only image-level labels is an essential application since it reduces the considerable human effort to fully annotate image. Most state-of-the-art methods take into account discriminative regions or prior knowledge (called seeds) by applying the recent Classification Activation Maps (CAM) methods. As seeds used in the CAM methods could be considered as segmentation knowledge, increasing seeds will naturally improve the performance of the CAM methods. We treat such task as a seed exploration with clustering learning, which is different from the current methods in which region mining or seeds learning are performed in an end-to-end learning manner. In this paper, we propose a progressive framework containing autoencoders to explore numerous and accurate seeds to further improve the performance of CAM methods. Our explored seeds can replace the initial seeds, and thus, they can be integrated with current weakly supervised semantic segmentation methods for performance improvement. We show experimentally that our provided seeds lead to better model training. As a result, we obtain performance improvement from corresponding counterparts to reach 68.1 and 69.2% mIoU on PASCAL VOC 2012 validation and test dataset, respectively.
更多查看译文
关键词
Weakly supervised learning,Semantic segmentation,Clustering learning,Autoencoder
AI 理解论文
溯源树
样例
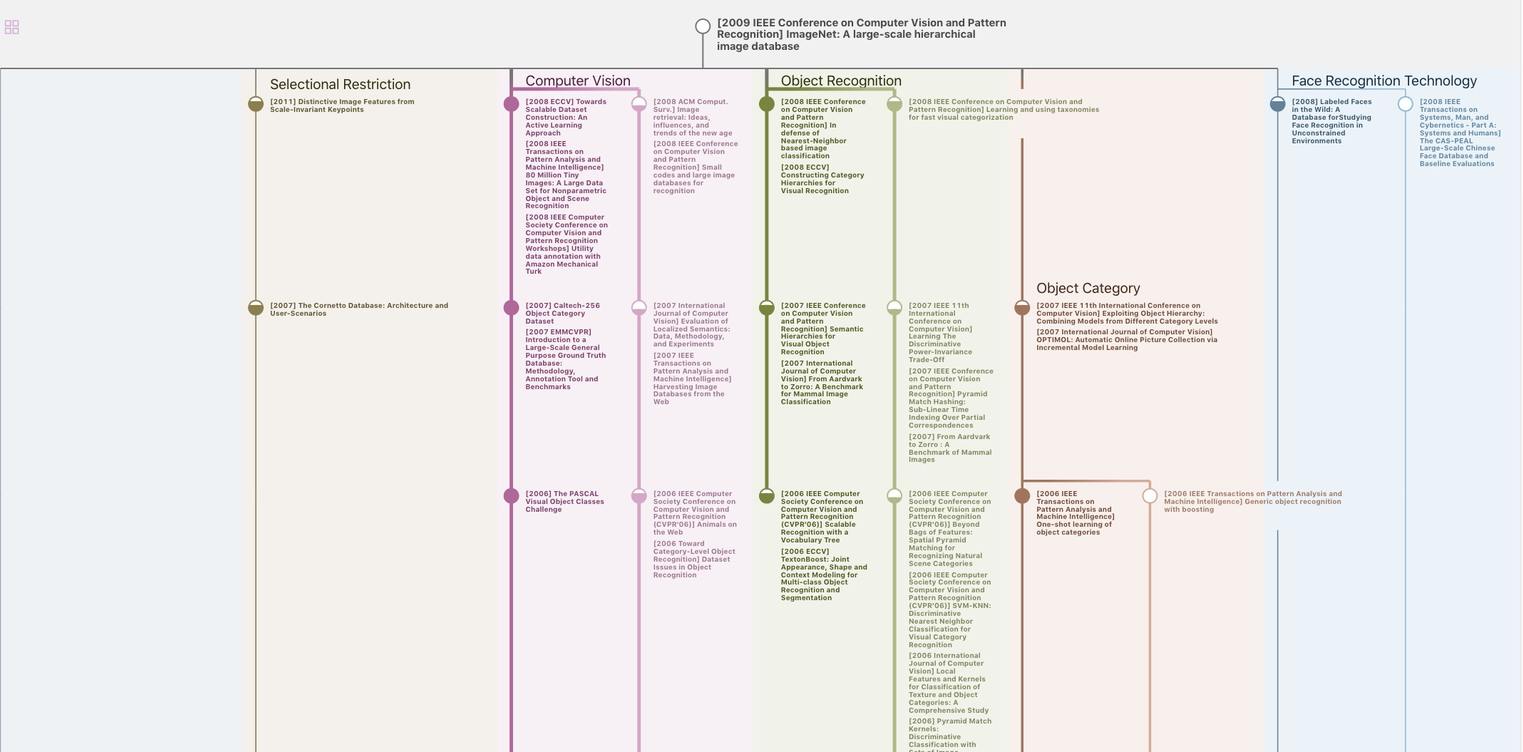
生成溯源树,研究论文发展脉络
Chat Paper
正在生成论文摘要