BERT Lost Patience Won't Be Robust to Adversarial Slowdown
NeurIPS(2023)
摘要
In this paper, we systematically evaluate the robustness of multi-exit language models against adversarial slowdown. To audit their robustness, we design a slowdown attack that generates natural adversarial text bypassing early-exit points. We use the resulting WAFFLE attack as a vehicle to conduct a comprehensive evaluation of three multi-exit mechanisms with the GLUE benchmark against adversarial slowdown. We then show our attack significantly reduces the computational savings provided by the three methods in both white-box and black-box settings. The more complex a mechanism is, the more vulnerable it is to adversarial slowdown. We also perform a linguistic analysis of the perturbed text inputs, identifying common perturbation patterns that our attack generates, and comparing them with standard adversarial text attacks. Moreover, we show that adversarial training is ineffective in defeating our slowdown attack, but input sanitization with a conversational model, e.g., ChatGPT, can remove perturbations effectively. This result suggests that future work is needed for developing efficient yet robust multi-exit models. Our code is available at: https://github.com/ztcoalson/WAFFLE
更多查看译文
关键词
adversarial slowdown,bert,patience
AI 理解论文
溯源树
样例
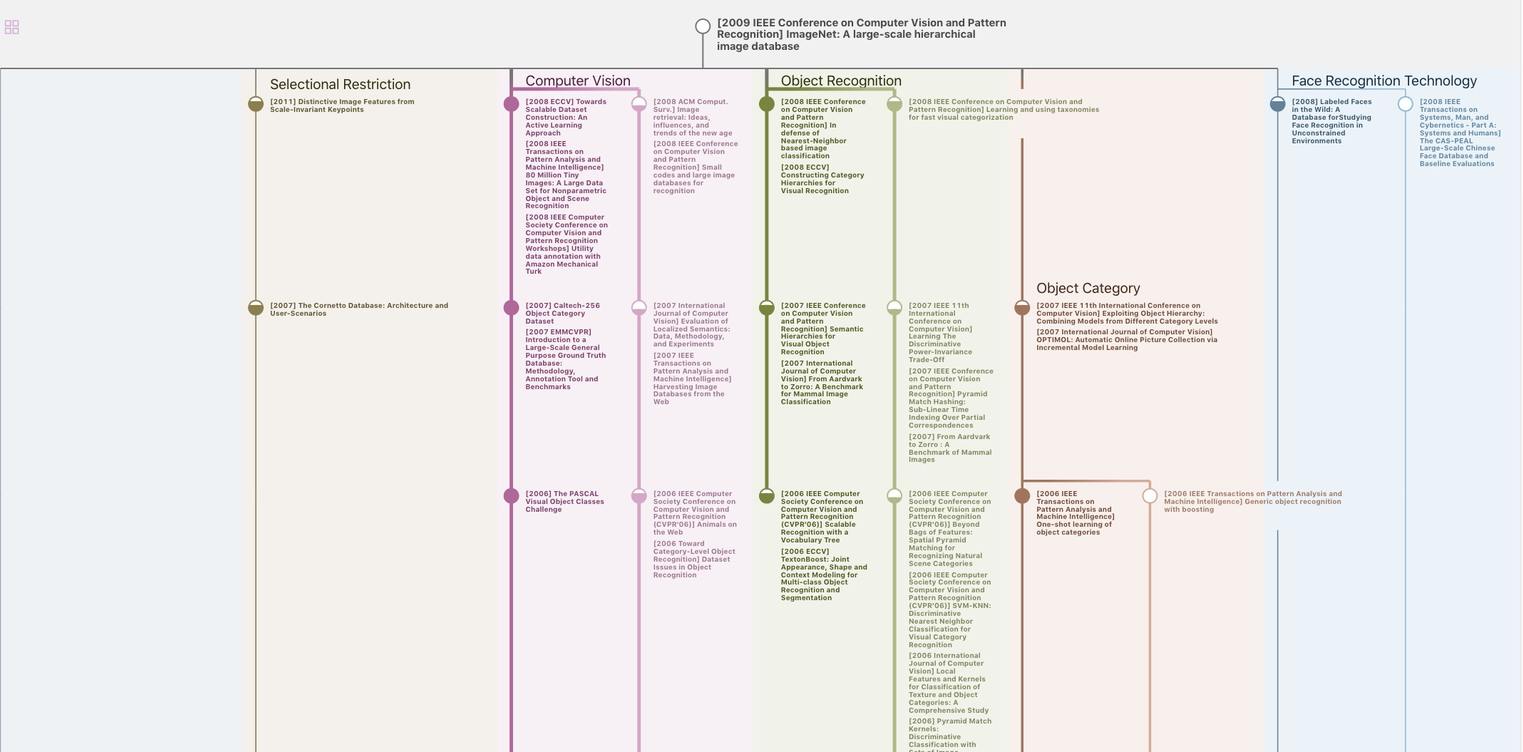
生成溯源树,研究论文发展脉络
Chat Paper
正在生成论文摘要