AutoMixer for Improved Multivariate Time-Series Forecasting on BizITOps Data
CoRR(2023)
摘要
The efficiency of business processes relies on business key performance indicators (Biz-KPIs), that can be negatively impacted by IT failures. BizITOps data fuses both Biz-KPIs and IT event channels together as multivariate time series data. Forecasting Biz-KPIs in advance can enhance efficiency and revenue through proactive corrective measures. However, BizITOps data generally exhibit both useful and noisy inter-channel interactions between Biz-KPIs and IT events that need to be effectively decoupled. This leads to suboptimal forecasting performance when existing multivariate forecasting models are employed. To address this, we introduce AutoMixer, a time-series Foundation Model (FM) approach, grounded on the novel technique of channel-compressed pretrain and finetune workflows. AutoMixer leverages an AutoEncoder for channel-compressed pretraining and integrates it with the advanced TSMixer model for multivariate time series forecasting. This fusion greatly enhances the potency of TSMixer for accurate forecasts and also generalizes well across several downstream tasks. Through detailed experiments and dashboard analytics, we show AutoMixer's capability to consistently improve the Biz-KPI's forecasting accuracy (by 11-15%) which directly translates to actionable business insights.
更多查看译文
AI 理解论文
溯源树
样例
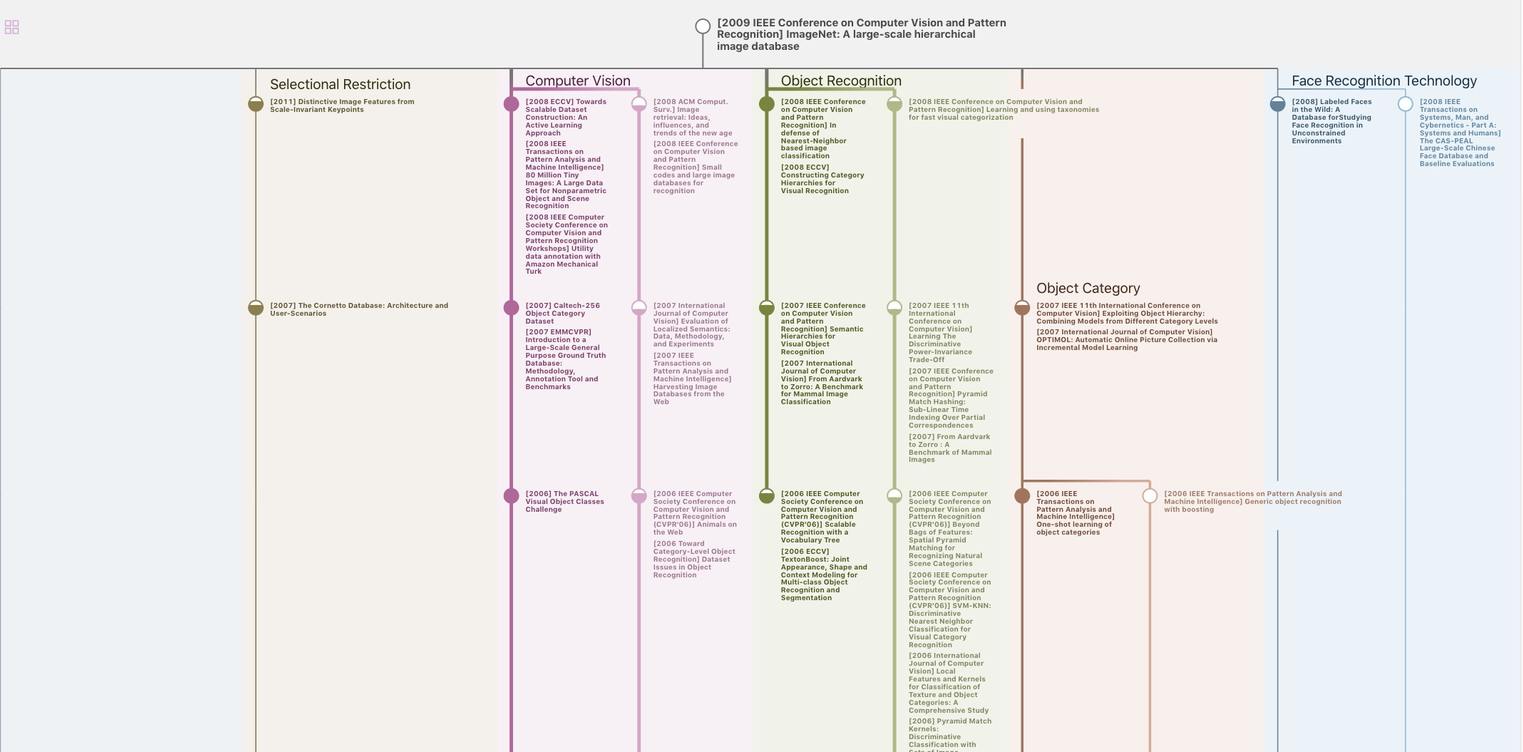
生成溯源树,研究论文发展脉络
Chat Paper
正在生成论文摘要