A hybrid meta-heuristic for the generation of feasible large-scale course timetables using instance decomposition
arXiv (Cornell University)(2023)
摘要
This study introduces a hybrid meta-heuristic for generating feasible course timetables in large-scale scenarios. We conducted tests using our university's instances. The current commercial software often struggles to meet constraints and takes hours to find satisfactory solutions. Our methodology combines adaptive large neighbourhood search, guided local search, variable neighbourhood search, and an innovative instance decomposition technique. Constraint violations from various groups are treated as objective functions to minimize. The search focuses on time slots with the most violations, and if no improvements are observed after a certain number of iterations, the most challenging constraint groups receive new weights to guide the search towards non-dominated solutions, even if the total sum of violations increases. In cases where this approach fails, a shaking phase is employed. The decomposition mechanism works by iteratively introducing curricula to the problem and finding new feasible solutions while considering an expanding set of lectures. Assignments from each iteration can be adjusted in subsequent iterations. Our methodology is tested on real-world instances from our university and random subdivisions. For subdivisions with 400 curricula timetables, decomposition reduced solution times by up to 27%. In real-world instances with 1,288 curricula timetables, the reduction was 18%. Clustering curricula with more common lectures and professors during increments improved solution times by 18% compared to random increments. Using our methodology, viable solutions for real-world instances are found in an average of 21 minutes, whereas the commercial software takes several hours.
更多查看译文
关键词
course timetables,instance decomposition,meta-heuristic,large-scale
AI 理解论文
溯源树
样例
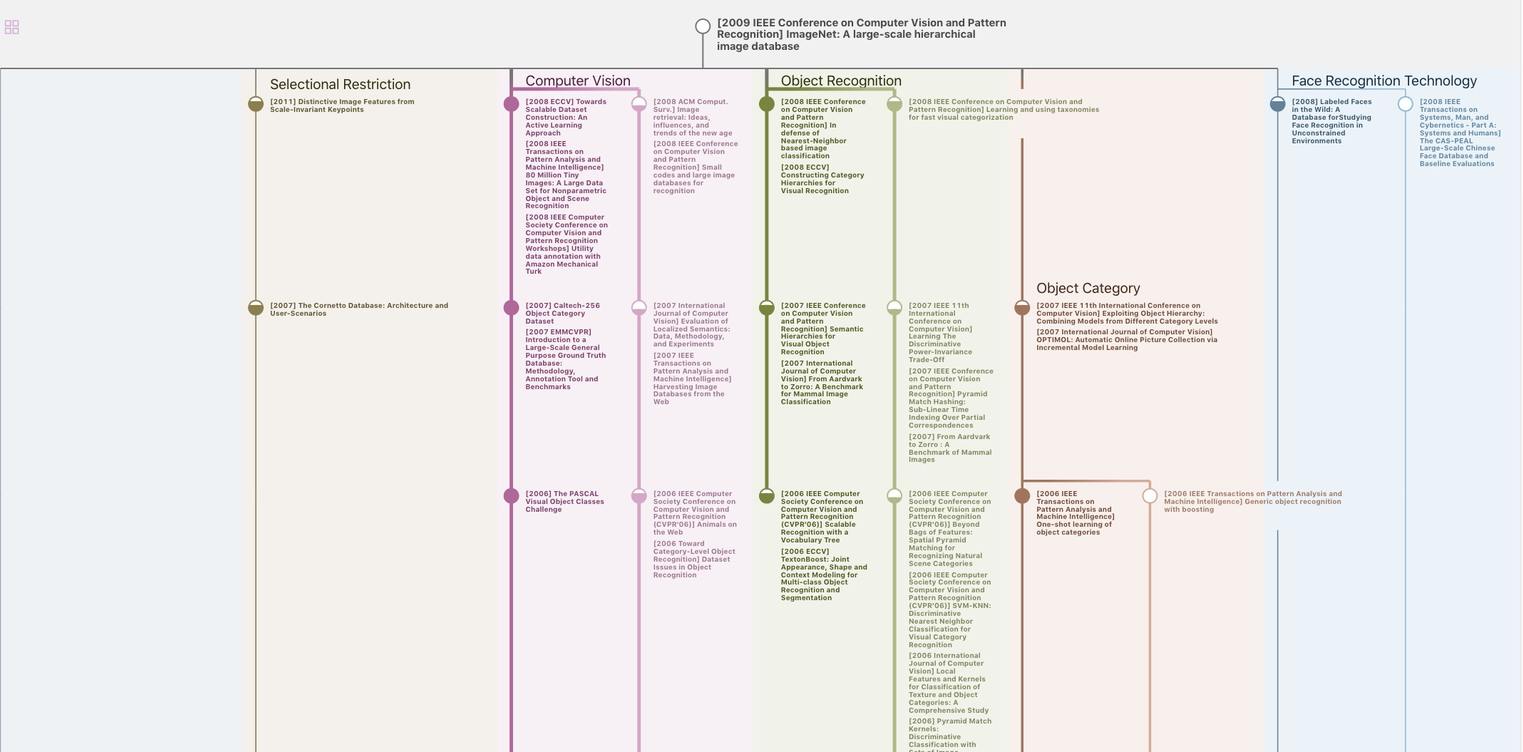
生成溯源树,研究论文发展脉络
Chat Paper
正在生成论文摘要