Not all fake news is semantically similar: Contextual semantic representation learning for multimodal fake news detection
INFORMATION PROCESSING & MANAGEMENT(2024)
摘要
Multimodal fake news detection, which aims to detect fake news across vast amounts of multimodal data in social networks, greatly contributes to identifying potential risks on the Internet. Although numerous fake news detection methods have been proposed and achieved some progress in recent years, almost all existing methods rely solely on global semantic features to detect fake news while ignoring that fake news is not consistently semantically similar. To fill the gap between news semantic feature space and fake news decision space, we propose a novel method, i.e., Contextual Semantic representation learning for multimodal Fake News Detection (CSFND), by introducing the context information into the representation learning process. Specifically, CSFND implements an unsupervised context learning stage to acquire the local context features of news, which are then fused with the global semantic features to learn the contextual semantic representation of news. In our proposed representation space, semantically dissimilar fake news is explicitly isolated and distinguished from real news separately. Moreover, CSFND devises a contextual testing strategy aimed at distinguishing between fake and real news within the data having similar semantics, wherein the learned decision boundaries are impervious to the semantic characteristics. Extensive experiments conducted on two real-world multimodal datasets demonstrate that CSFND significantly outperforms ten state-of-the-art competitors in detecting fake news and outperforms the best baselines on two datasets by 2.5% on average in terms of Accuracy.
更多查看译文
关键词
Fake news detection,Multimodal learning,Social network,Representation learning,Deep learning
AI 理解论文
溯源树
样例
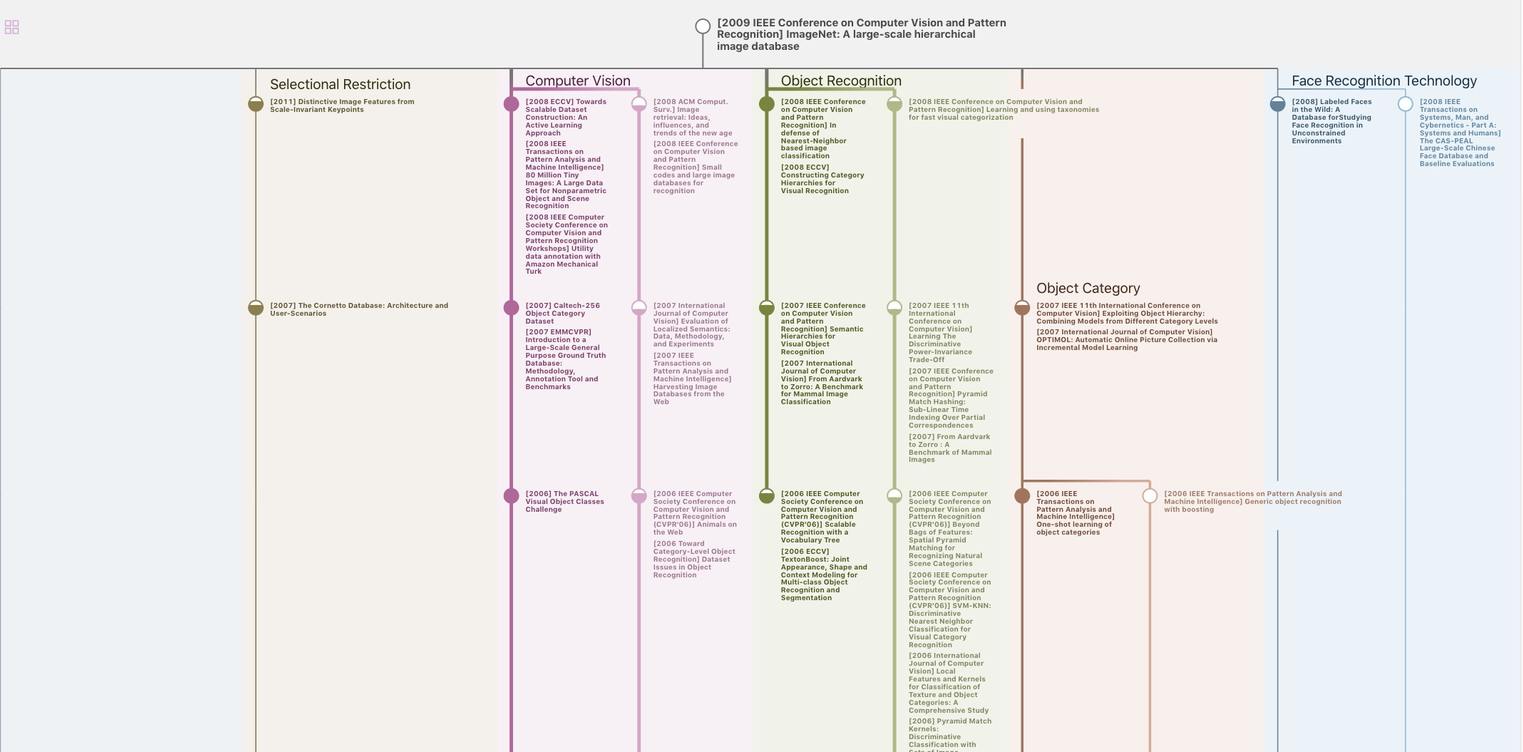
生成溯源树,研究论文发展脉络
Chat Paper
正在生成论文摘要