Nonlinear learning method for local causal structures
INFORMATION SCIENCES(2024)
摘要
Recent years have witnessed the proliferation of causal learning techniques, aimed at extracting the abundant causal relationships embedded within observational data. In many scenarios, our primary focus lies in predicting a single target variable. In such cases, it becomes both inefficient and unnecessary to learn an entire causal network through advanced global learning methods. To address this challenge, the concept of local causal learning has been introduced to identify the direct causes and effects of a target variable of interest. However, current algorithms exhibit limitations stemming from their reliance on conditional independence tests, which only consider the linear and pairwise relationships but ignore the ubiquitous nonlinear and multivariate causality, making them lose efficacy in practical scenarios. This paper takes significant strides toward facilitating the real-world applications for local causal learning. To identify the nonlinear relations, this paper discovers the Markov boundary (MB) through calculating the minimal conditional covariance operator in reproducing kernel Hilbert space. This approach establishes a theoretical equivalence between the solution and MB. Subsequently, a nonlinear scoring mechanism for structure learning is employed based on the selected subset, yielding the optimal local causal structure. A series of extensive experiments serves to underscore the superiority of the proposed method.
更多查看译文
关键词
Causal learning,Causal discovery,Local causal structure,Bayesian network,Markov boundary,Markov blanket
AI 理解论文
溯源树
样例
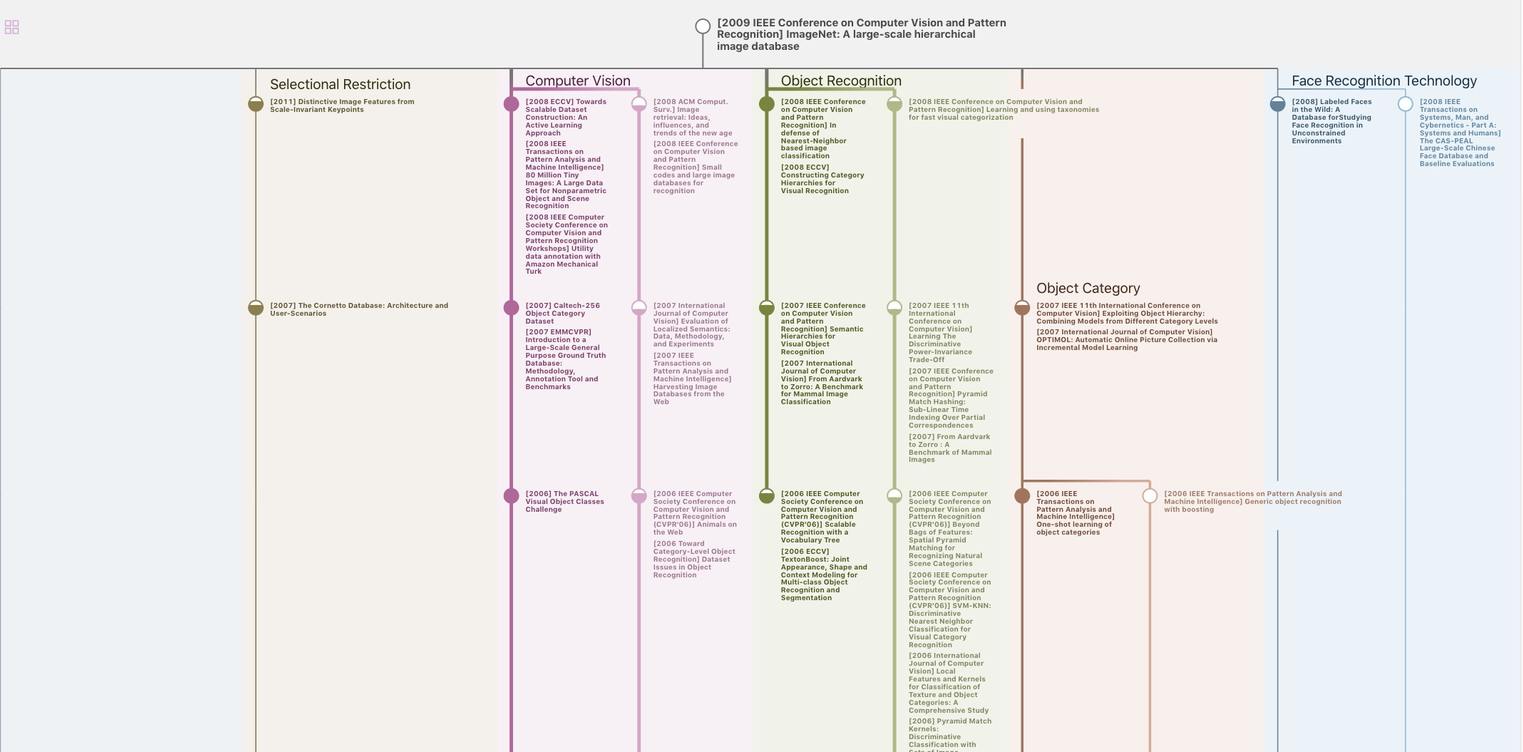
生成溯源树,研究论文发展脉络
Chat Paper
正在生成论文摘要