Adaptive modeling and inference of higher-order coordination in neuronal assemblies: a dynamic greedy estimation approach
bioRxiv : the preprint server for biology(2023)
摘要
Central in the study of population codes, coordinated ensemble spiking activity is widely observable in neural recordings with hypothesized roles in robust stimulus representation, interareal communication, and learning and memory formation. Model-free measures of synchrony characterize coherent pairwise activity but not higher-order interactions, a limitation transcended by statistical models of ensemble spiking activity. However, existing model-based analyses often impose assumptions about the relevance of higher-order interactions and require repeated trials to characterize dynamics in the correlational structure of ensemble activity. To address these shortcomings, we propose an adaptive greedy filtering algorithm based on a discretized mark point-process model of ensemble spiking and a corresponding statistical inference framework to identify significant higher-order coordination. In the course of developing a precise statistical test, we show that confidence intervals can be constructed for greedily estimated parameters. We demonstrate the utility of our proposed methods on simulated neuronal assemblies. Applied to multi-electrode recordings from human and rat cortical assemblies, our proposed methods provide new insights into the dynamics underlying localized population activity during transitions between brain states.
Author summary Simultaneous ensemble spiking is hypothesized to have important roles in neural encoding; however, neurons can also spike simultaneously by chance. In order to characterize the potentially time-varying higher-order correlational structure of ensemble spiking, we propose an adaptive greedy filtering algorithm that estimates the rate of all reliably-occurring simultaneous ensemble spiking events. Moreover, we propose an accompanying statistical inference framework to distinguish the chance occurrence of simultaneous spiking events from coordinated higher-order spiking. We demonstrate the proposed methods accurately differentiate coordinated simultaneous spiking from chance occurrences in simulated data. In application to human and rat cortical data, the proposed methods reveal time-varying dynamics in higher-order coordination that coincide with changing brain states.
### Competing Interest Statement
The authors have declared no competing interest.
更多查看译文
关键词
neuronal assemblies,coordination,dynamic greedy estimation approach,adaptive modeling,higher-order
AI 理解论文
溯源树
样例
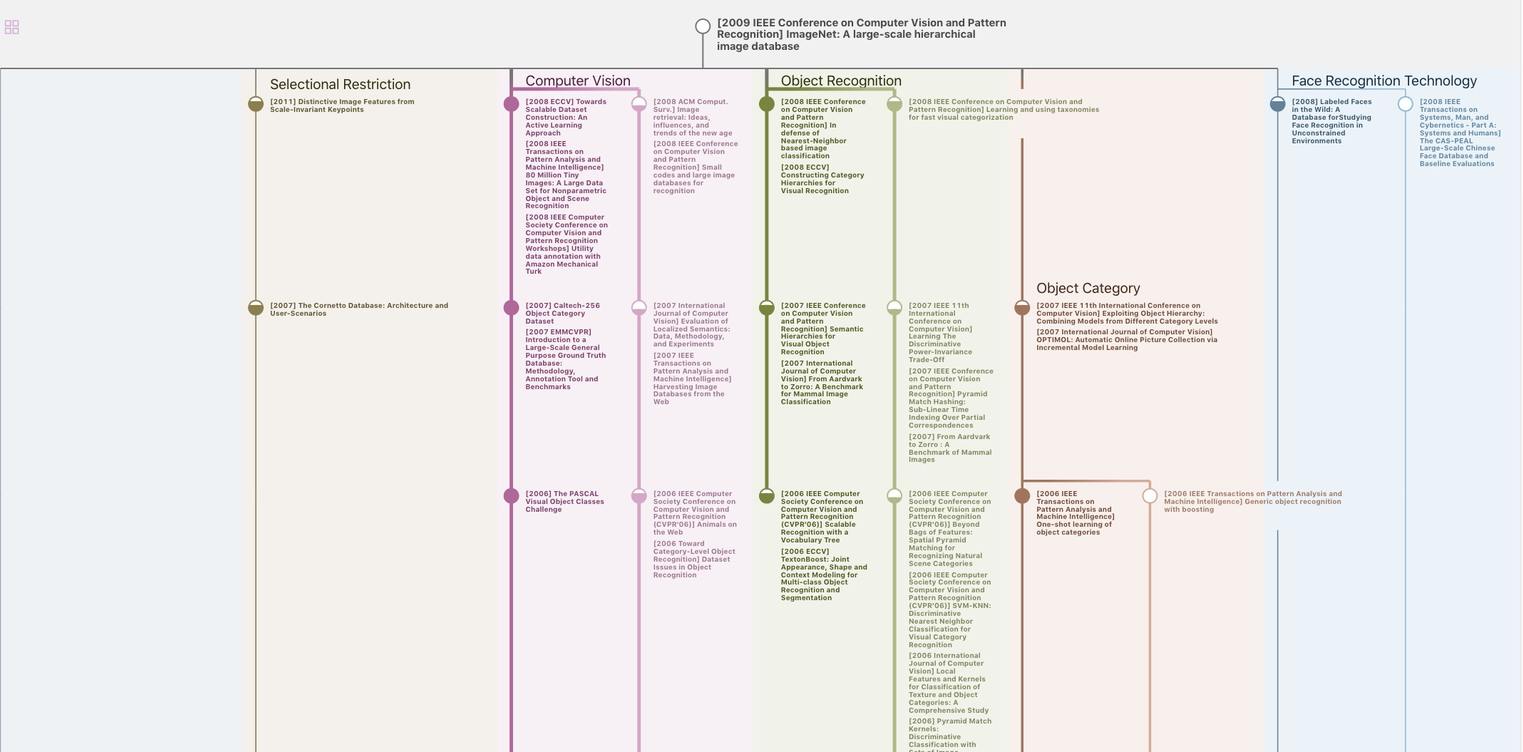
生成溯源树,研究论文发展脉络
Chat Paper
正在生成论文摘要