Guarding Deep Learning Systems With Boosted Evasion Attack Detection and Model Update.
IEEE Internet Things J.(2024)
摘要
Deep learning systems are susceptible to evasion attacks, which represent a significant category of security vulnerabilities. These attacks entail the alteration of input data in such a way that the victim Deep Neural Network (DNN) misclassifies it. Researchers have devised detection and defense methods to counter evasion attacks; however, these techniques impose a significant computational burden and are not suitable for real-time detection on devices with limited resources. Our paper presents an infrastructure, GERALT designed to improve the efficiency of evasion attack detection for real-time execution on edge devices. It involves a partition analysis that optimizes detection methods and allows for the use of a smaller detection network. Additionally, we propose a hardware architecture that accelerates inter-network inference using intermediate data reuse techniques and enables a different pattern of model updates between cloud servers and edge devices in real-world applications. Furthermore, it is also extended to a principle of inter-network accelerator design, which is evaluated at different PE ratios. Our evaluations demonstrate that GERALT achieves more than 3x improvement in performance compared to standard accelerators like Eyeriss, without affecting detection and classification accuracy. The boosted model update system avoids the bandwidth limit between edge devices and the cloud server, saving 14 hours when updating the model for a new evasion attack.
更多查看译文
关键词
Evasion Attack Detection,Inference Accelerator,Deep Neural Network,Adversarial Training,Adversarial Example,Model Update from Cloud,Image Classification
AI 理解论文
溯源树
样例
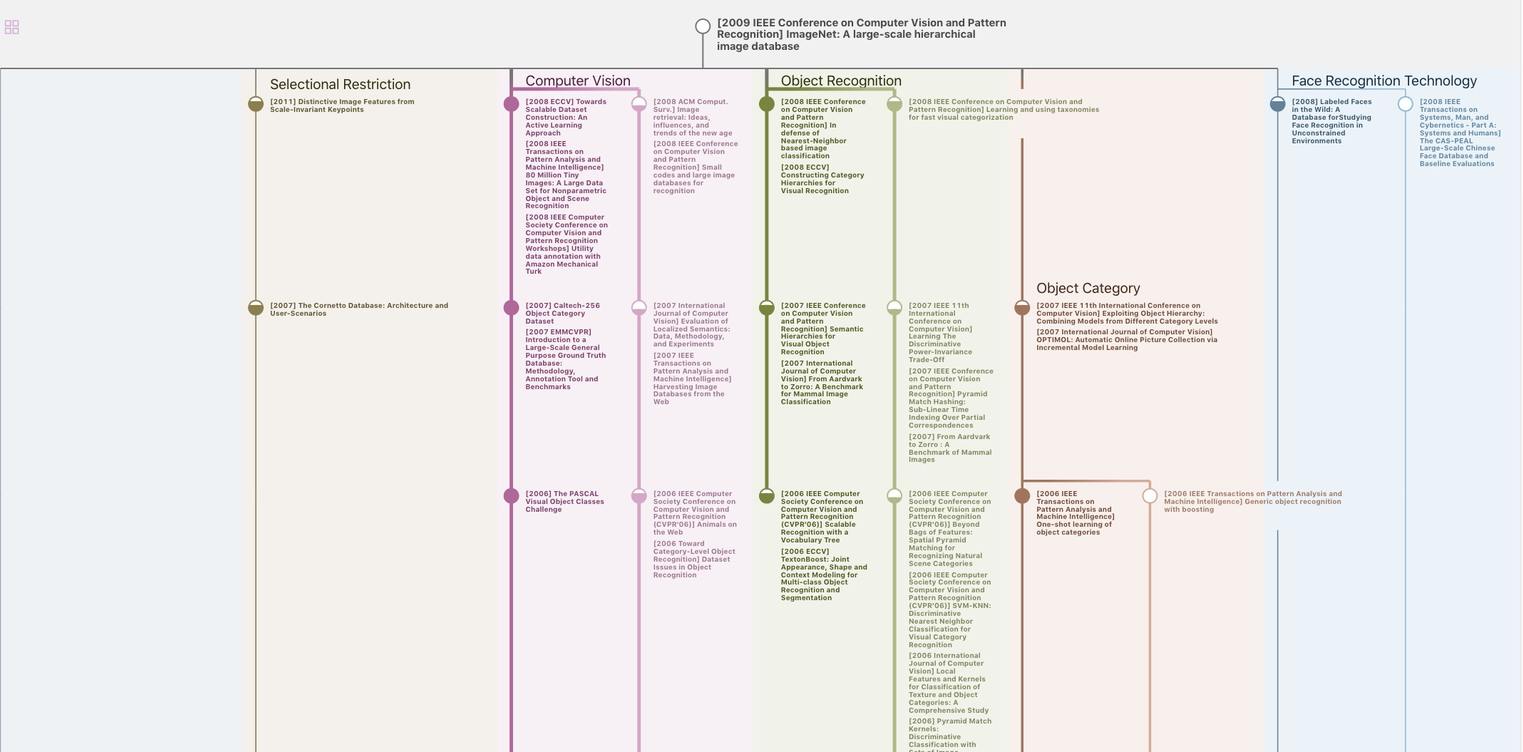
生成溯源树,研究论文发展脉络
Chat Paper
正在生成论文摘要