Privacy-Preserving Federated Learning Based On Partial Low-Quality Data
Research Square (Research Square)(2023)
摘要
Abstract Traditional machine learning requires collecting data from participants for training, which may result in malicious acquisition of privacy in participants' data. Federated learning offers a method to protect participants' data privacy by transferring the training process from a centralized server to terminal devices. However, the server may still obtain participants' privacy information through inference attacks, among other methods. Additionally, the data provided by participants varies in quality, and excessive involvement of low-quality data in the training process can render the model unusable, which is an important issue in current mainstream Federated learning. To address the aforementioned issues, this paper presents a Privacy Preserving Federated learning Scheme with Partial Low-Quality Data (PPFL-LQDP). It achieves good training results while allowing participants to utilize partial low-quality data, thereby enhancing the privacy and ronutness of the Federated learning scheme. Specifically, we use a modified distributed Paillier cryptographic mechanism to protect the privacy and security of participants' data during the Federated training process. Simultaneously, we construct composite evaluation values for the data held by participants to reduce the involvement of low-quality data, thereby minimizing the negative impact of such data on the model. Through experiments on the MNIST dataset, we demonstrate that this scheme can complete the model training of Federated learning with the participation of partial low-quality data, while effectively protecting the security and privacy of participants' data. Comparisons with related schemes also show that our scheme has good overall performance.
更多查看译文
关键词
privacy-preserving,low-quality
AI 理解论文
溯源树
样例
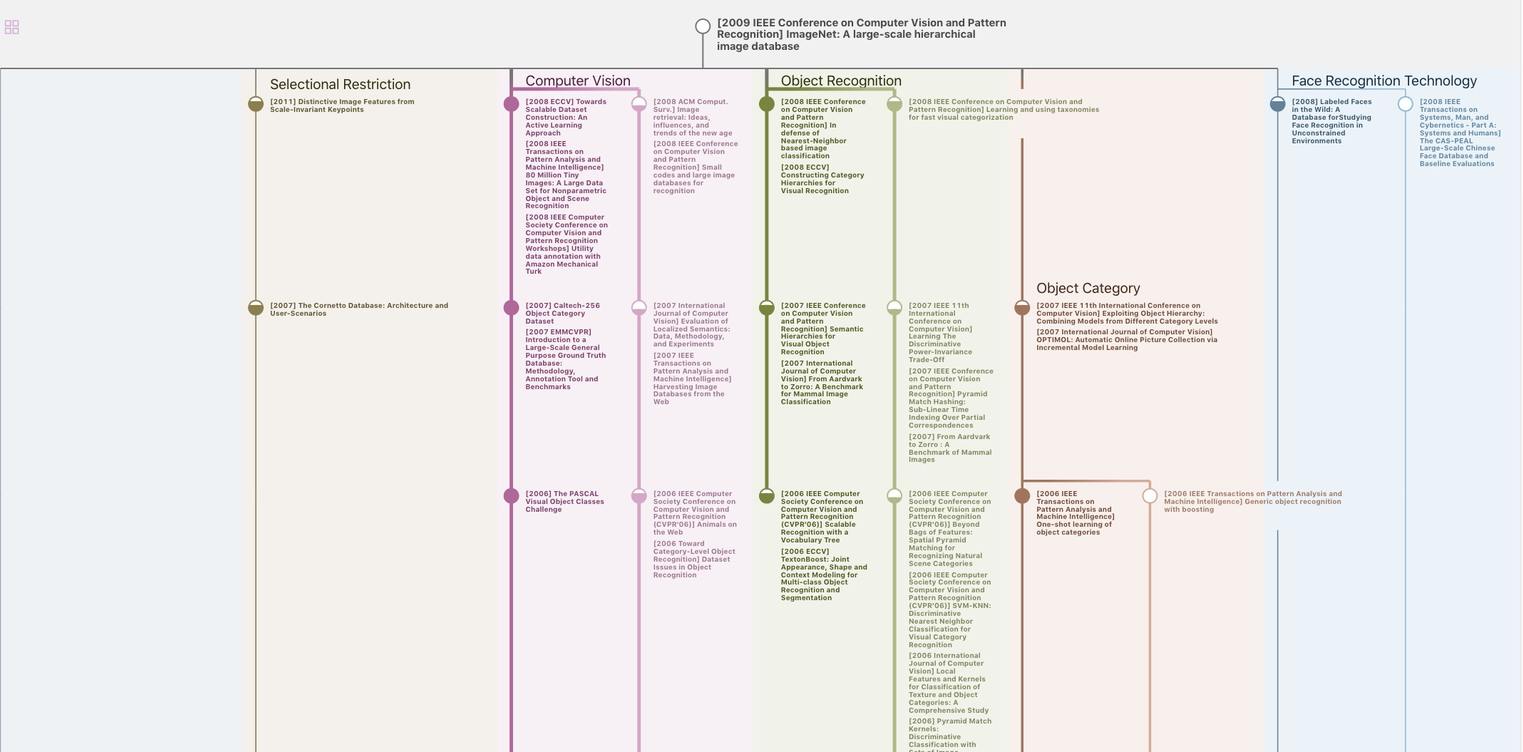
生成溯源树,研究论文发展脉络
Chat Paper
正在生成论文摘要