LEPCNet: A Lightweight End-to-End PCG Classification Neural Network Model for Wearable Devices.
IEEE Trans. Instrum. Meas.(2024)
摘要
Wearable intelligent phonocardiogram (PCG) sensors provide a non-invasive method for long-term monitoring of cardiac status, which is crucial for the early detection of cardiovascular diseases. As one of the key technologies for intelligent PCG sensors, PCG classification techniques based on computer audition have been widely leveraged in recent years, such as Convolutional Neural Networks, Generative Adversarial Nets, and Long Short-Term Memory. However, the limitation of these methods is that the models have a sizeable computational complexity, which is not suitable for wearable devices. To this end, we propose an end-to-end neural network for PCG classification with low computational complexity (52.67K parameters and 1.59M floating point operations per second (FLOPs). We utilise two public datasets to test the model, and experimental results demonstrate that the proposed model achieves an accuracy of 93.1% in the 2016 PhysioNet/CinC Challenge dataset with considerable complexity reduction compared to the state-of-the-art works. Moreover, we design an energy-efficient wearable PCG sensor and deploy the proposed algorithms on it. The experimental results show that our proposed model consumes only 245.1mW for PCG classification with an accuracy of 89.8% on test datasets. This means that the proposed model obtains excellent performance compared to previous work while consuming lower power, which is significant in practical application scenarios.
更多查看译文
关键词
Lightweight Model,Heart Sound,End-to-End,Computer Audition,Intelligent Sensor
AI 理解论文
溯源树
样例
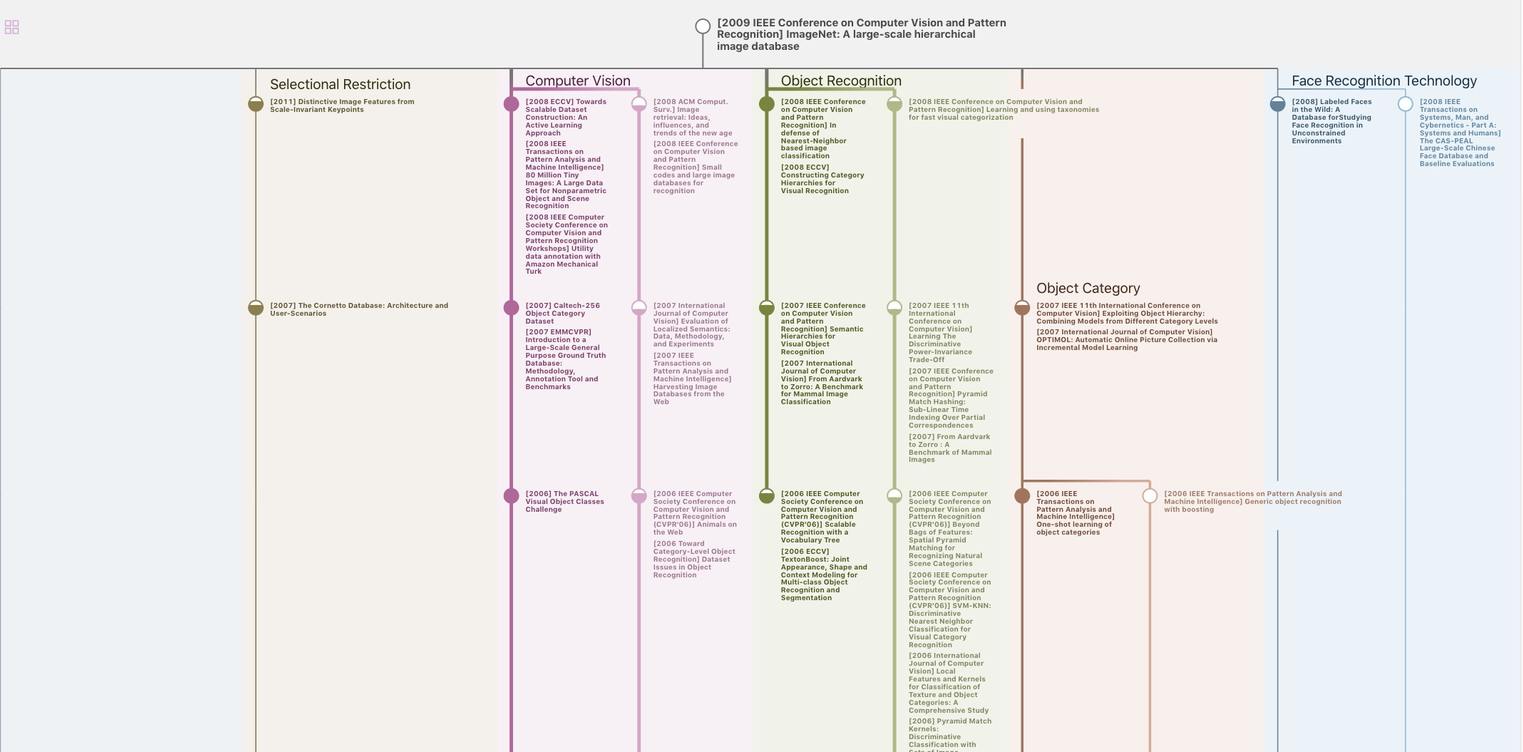
生成溯源树,研究论文发展脉络
Chat Paper
正在生成论文摘要