Thermal Degradation of Polycrystalline Ni-Rich Cathodes, New Insights from Total Scattering and Machine-Learning Assisted Tomography
Meeting abstracts(2023)
摘要
Alkali metal-based batteries (e.g., lithium batteries) are considered the most effective energy storage candidate for portable electronics, power grids, and electric vehicles due to their considerable energy density, power density, cycle life, safety, and competitive prices. The Ni-rich Co-less ternary LiNi x Mn y Co 1- x - y O 2 (NMC) (Ni>80%) is one of the candidate materials due to its high discharge capacity (>200 mAh g -1 ) and high energy density (>750 Wh kg -1 ). However, the thermal stability of charged Ni-rich NMCs is inferior, following a general negative correlation between Ni content and stability. Such severer degradation in Ni-rich NMCs might lead to dysfunctionality, thermal runaway, and eventually catastrophic failure and explosion of batteries, raising enormous safety concerns. Therefore, it is critical to understand the thermal behavior of charged Ni-rich NMC cathode from different perspectives in order to conduct material design for better thermal stability. After literature survey of typical and widely used probes by this community (e.g., diffraction, spectroscopy, imaging), we found these approaches subject to compromise to some degree in the experimental design, resulting in an inadequate description of the thermal degradation mechanism. Here we offer two novel approaches, neutron total scattering and energy-resolved 3D nano-tomography, to probe the reaction and evolution of the charged NMCs under non-ambient conditions in real-time. Such in situ measurements were only accomplished by deliberate experimental design at beamlines and deep integrations of neutron or synchrotron facilities with battery science. In situ heating neutron scattering captures the transition of crystal structure in reciprocal space (by Rietveld refinements), as well as of the local atomic framework in real space (by pair distribution function analysis). Moreover, machine learning algorithms were applied to the 3D nano-tomography datasets to reveal the detailed microstructural and oxidation state progression inside the charged particles. Thereafter, we discussed the key factors which have previously been overlooked by the community and offer constructive suggestions on particle design for thermally stable cathodes from our unique perspective. The work was supported by the National Science Foundation under Grant no. DMR-1832613 (F.L.). The machine-learning assisted tomography analysis was partially supported from NASA under 80NSSC21M0333 and NSF under NSF-2119688 (X.-D.Z)
更多查看译文
关键词
thermal degradation,ni-rich,machine-learning
AI 理解论文
溯源树
样例
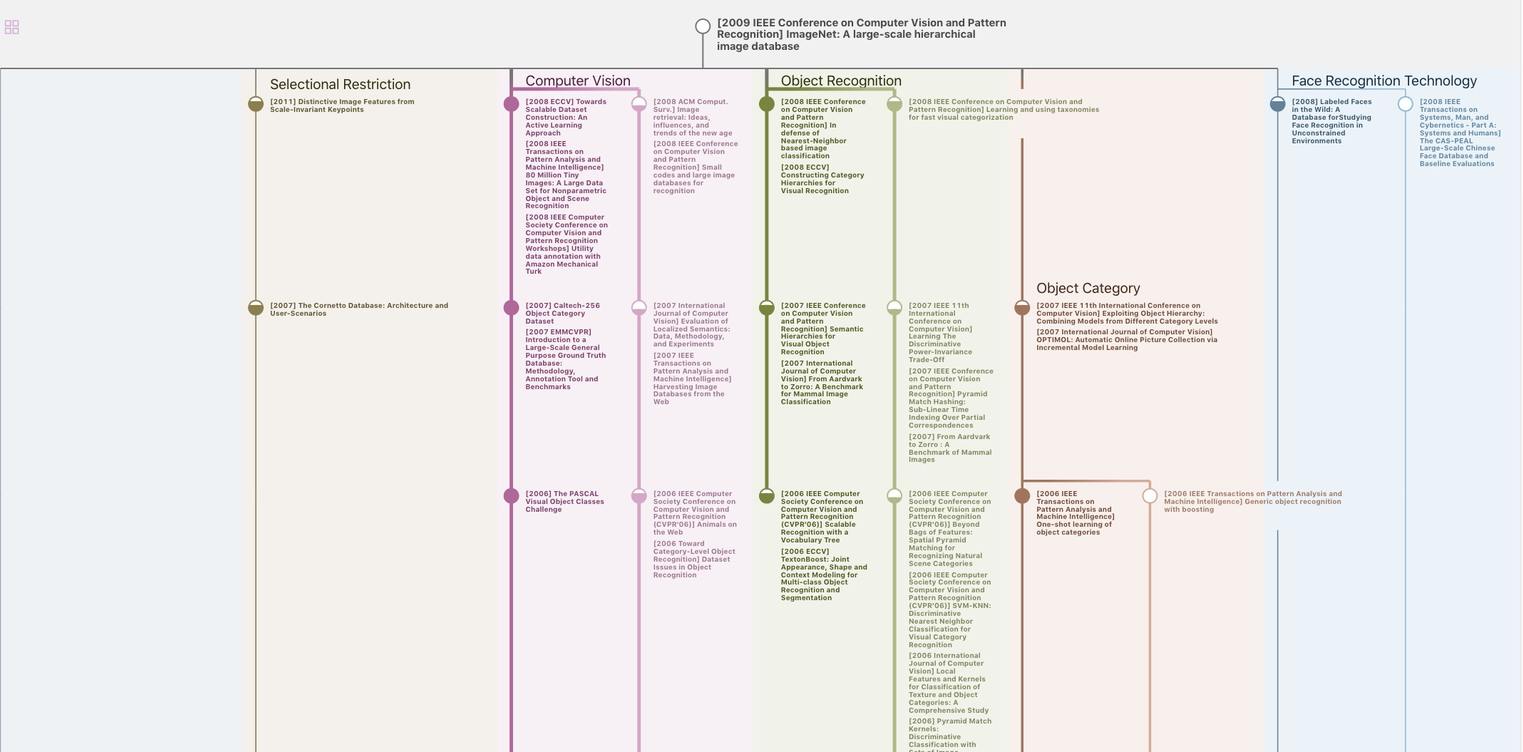
生成溯源树,研究论文发展脉络
Chat Paper
正在生成论文摘要