Mu-Net a Light Architecture for Small Dataset Segmentation of Brain Organoid Bright-Field Images
Biomedicines(2023)
摘要
To characterize the growth of brain organoids (BOs), cultures that replicate some early physiological or pathological developments of the human brain are usually manually extracted. Due to their novelty, only small datasets of these images are available, but segmenting the organoid shape automatically with deep learning (DL) tools requires a larger number of images. Light U-Net segmentation architectures, which reduce the training time while increasing the sensitivity under small input datasets, have recently emerged. We further reduce the U-Net architecture and compare the proposed architecture (MU-Net) with U-Net and UNet-Mini on bright-field images of BOs using several data augmentation strategies. In each case, we perform leave-one-out cross-validation on 40 original and 40 synthesized images with an optimized adversarial autoencoder (AAE) or on 40 transformed images. The best results are achieved with U-Net segmentation trained on optimized augmentation. However, our novel method, MU-Net, is more robust: it achieves nearly as accurate segmentation results regardless of the dataset used for training (various AAEs or a transformation augmentation). In this study, we confirm that small datasets of BOs can be segmented with a light U-Net method almost as accurately as with the original method.
更多查看译文
关键词
brain organoid,segmentation,U-Net,Mini-UNet,MU-Net,bright-field
AI 理解论文
溯源树
样例
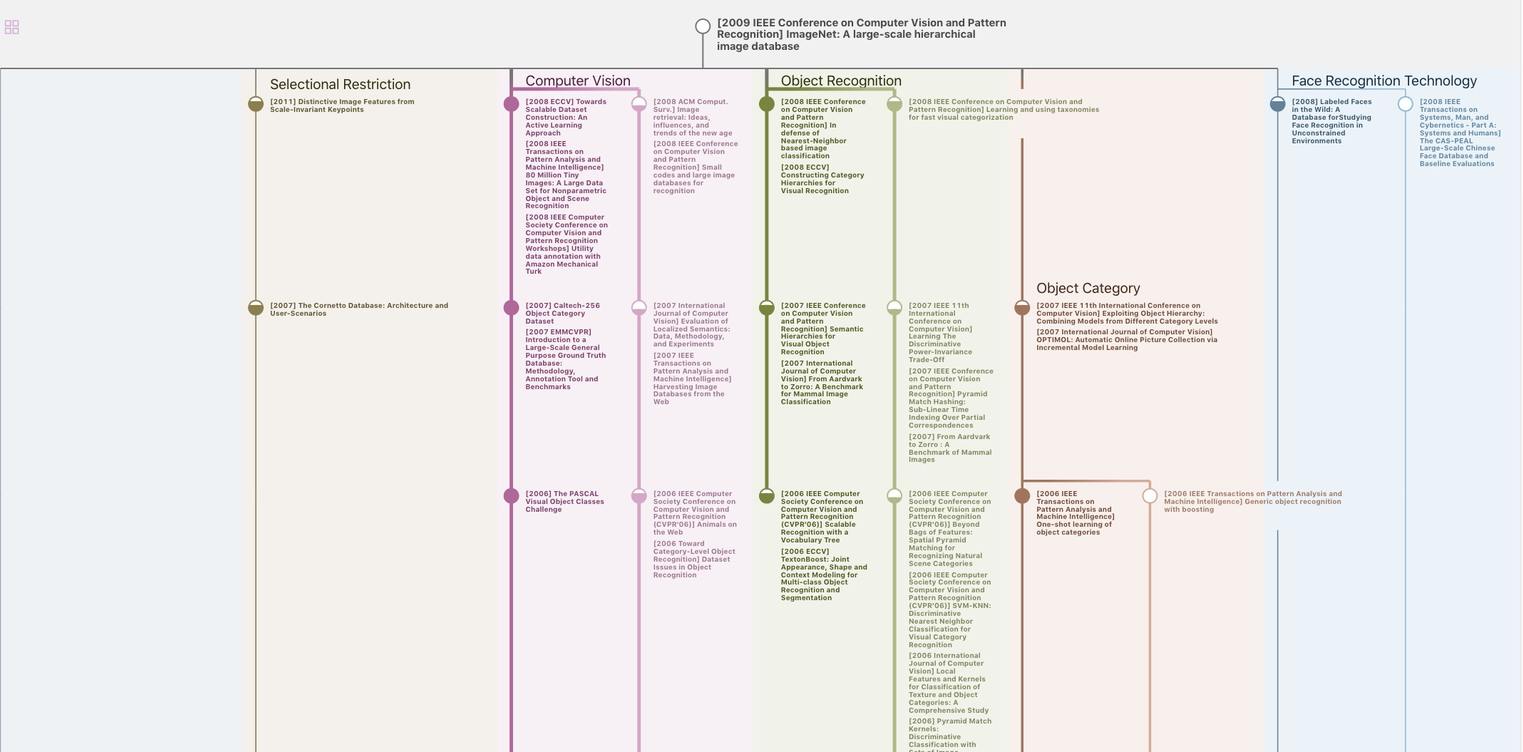
生成溯源树,研究论文发展脉络
Chat Paper
正在生成论文摘要