Transfer Learning Allows Accurate RBP Target Site Prediction with Limited Sample Sizes
Biology(2023)
摘要
Simple Summary RNA-binding proteins play crucial roles in essential biological processes, and disruptions in their functionality can lead to various diseases, including cancer. Despite the significant progress that computational deep learning methods have made in identifying their binding sites, obtaining high-quality data in sufficient amounts remains a major challenge, impeding development of accurate predictive models for many proteins. In this work, we present a novel approach to address the limited availability of training samples by leveraging transfer learning for predicting RBP binding sites. Using three input features and a sophisticated network architecture, we demonstrate the substantial advantages of employing transfer learning in a reusable and interpretable manner, as showcased on two prominent benchmark datasets for RNA-binding proteins.Abstract RNA-binding proteins are vital regulators in numerous biological processes. Their disfunction can result in diverse diseases, such as cancer or neurodegenerative disorders, making the prediction of their binding sites of high importance. Deep learning (DL) has brought about a revolution in various biological domains, including the field of protein-RNA interactions. Nonetheless, several challenges persist, such as the limited availability of experimentally validated binding sites to train well-performing DL models for the majority of proteins. Here, we present a novel training approach based on transfer learning (TL) to address the issue of limited data. Employing a sophisticated and interpretable architecture, we compare the performance of our method trained using two distinct approaches: training from scratch (SCR) and utilizing TL. Additionally, we benchmark our results against the current state-of-the-art methods. Furthermore, we tackle the challenges associated with selecting appropriate input features and determining optimal interval sizes. Our results show that TL enhances model performance, particularly in datasets with minimal training data, where satisfactory results can be achieved with just a few hundred RNA binding sites. Moreover, we demonstrate that integrating both sequence and evolutionary conservation information leads to superior performance. Additionally, we showcase how incorporating an attention layer into the model facilitates the interpretation of predictions within a biologically relevant context.
更多查看译文
关键词
transfer,prediction,learning
AI 理解论文
溯源树
样例
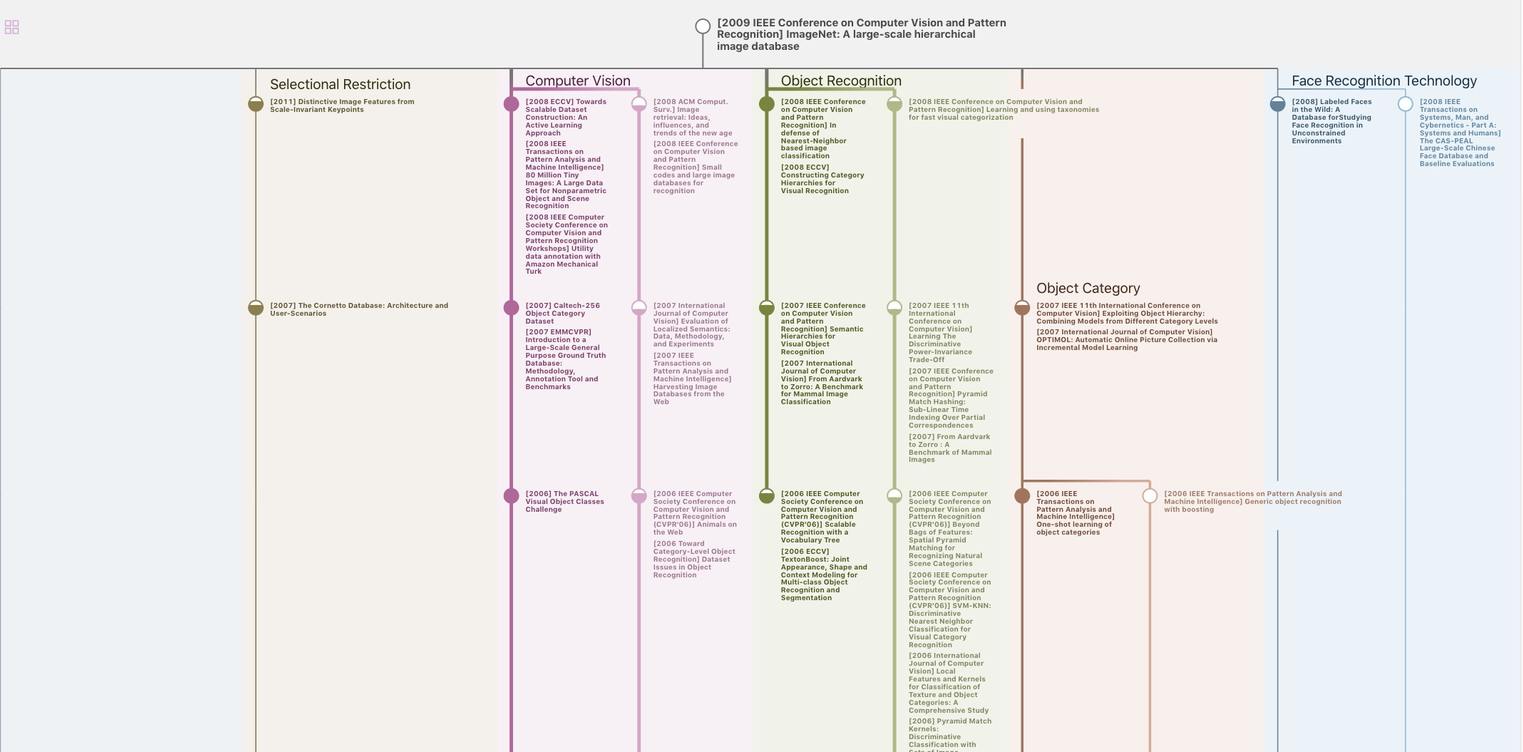
生成溯源树,研究论文发展脉络
Chat Paper
正在生成论文摘要