A non-local grouping tensor train decomposition model for travel demand analysis concerning categorical independent variables
Transportation Research Part C: Emerging Technologies(2023)
摘要
With the booming of transportation big data, plenty of spatial and temporal features become available for developing travel demand analysis models. However, existing machine learning based travel demand fitting models could face significant model complexity issues once there are multiple discrete/categorical independent variables. This paper embeds a non-local grouping (clustering) process into the tensor train decomposition (NLG-TTD) to characterize the higherorder and nonlinear relationship between categorical variables and travel demand. That is, the transportation dataset with multiple discrete/categorical independent variables and travel demand is treated as a multi-mode tensor, wherein levels of a discrete/categorical variable are regarded as indexes of a mode in the tensor, leading to a "regression on index". The proposed NLG-TTD model divides the full tensor into non-local sub-tensors, clusters sub-tensors with similarity, conducts tensor train decomposition (TTD) within each cluster, and aggregates clusterspecific learning results. Compared with a conventional TTD that generally works on image completions, NLG-TTD can reveal non-Euclidean and non-linear patterns between categorical/ discrete levels in independent variables. It is expected to discover similar travel demand patterns among categorical levels and provide high-level goodness-of-fit. For numerical studies, we use bike-sharing usage data and metro demand data in New York City (NYC), in which several widelyused machine learning models are trained for comparison. The results demonstrate the good performance of the proposed model. Moreover, we apply the NLG-TTD model to analyze and predict the impact of land use shifts on travel demand.
更多查看译文
关键词
travel demand analysis,tensor train decomposition model,categorical independent variables,non-local
AI 理解论文
溯源树
样例
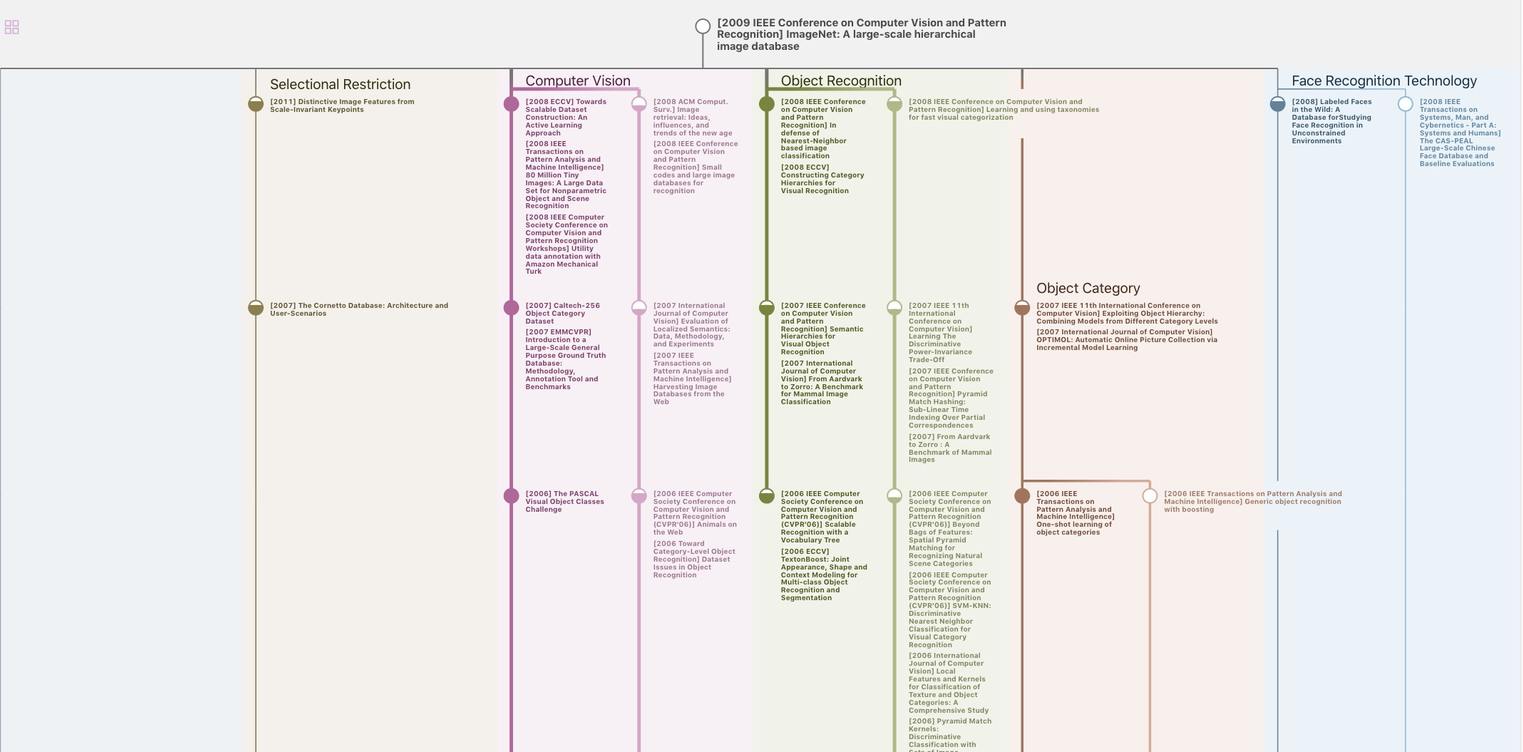
生成溯源树,研究论文发展脉络
Chat Paper
正在生成论文摘要