Machine learning attacks resistant strong PUF design utilizing response obfuscates challenge with lower hardware overhead
MICROELECTRONICS JOURNAL(2023)
摘要
Physical unclonable function (PUF) is a hardware security primitive with significant application potential. However, strong PUF is vulnerable to machine learning attacks based on modeling attacks. Although various resistance techniques have been proposed, strong PUF suffers from deficiencies in its resistance to machine learning attacks, hardware overhead, and reliability. This study proposes a highly reliable and secure lightweight PUF that complicates the original challenge using an internal response. Specifically, the internal response is used to determine the odd/even bit flip or left/right cyclic shift of the original challenge to achieve preliminary obfuscation, and byte substitution that exploits an S-box is performed on the preliminary obfuscated challenge to achieve deep nonlinear obfuscation. Experimental results on a Xilinx Artix-7 FPGA show that the proposed PUF is not only highly resistant to machine learning (ML) attacks but also has a low hardware overhead. An arbiter PUF (APUF), which uses the proposed obfuscated scheme with a 64-bit challenge length, was evaluated by three advanced machine learning algorithms with up to 600,000 challenge-response-pairs (CRPs) in the dataset, and the observed prediction accuracy was less than 56 %. Moreover, the PUF is unique, reliable, and has a significantly lower hardware overhead than other prior anti-attack PUFs.
更多查看译文
关键词
Strong PUF,Machine learning attacks,Obfuscation,FPGA
AI 理解论文
溯源树
样例
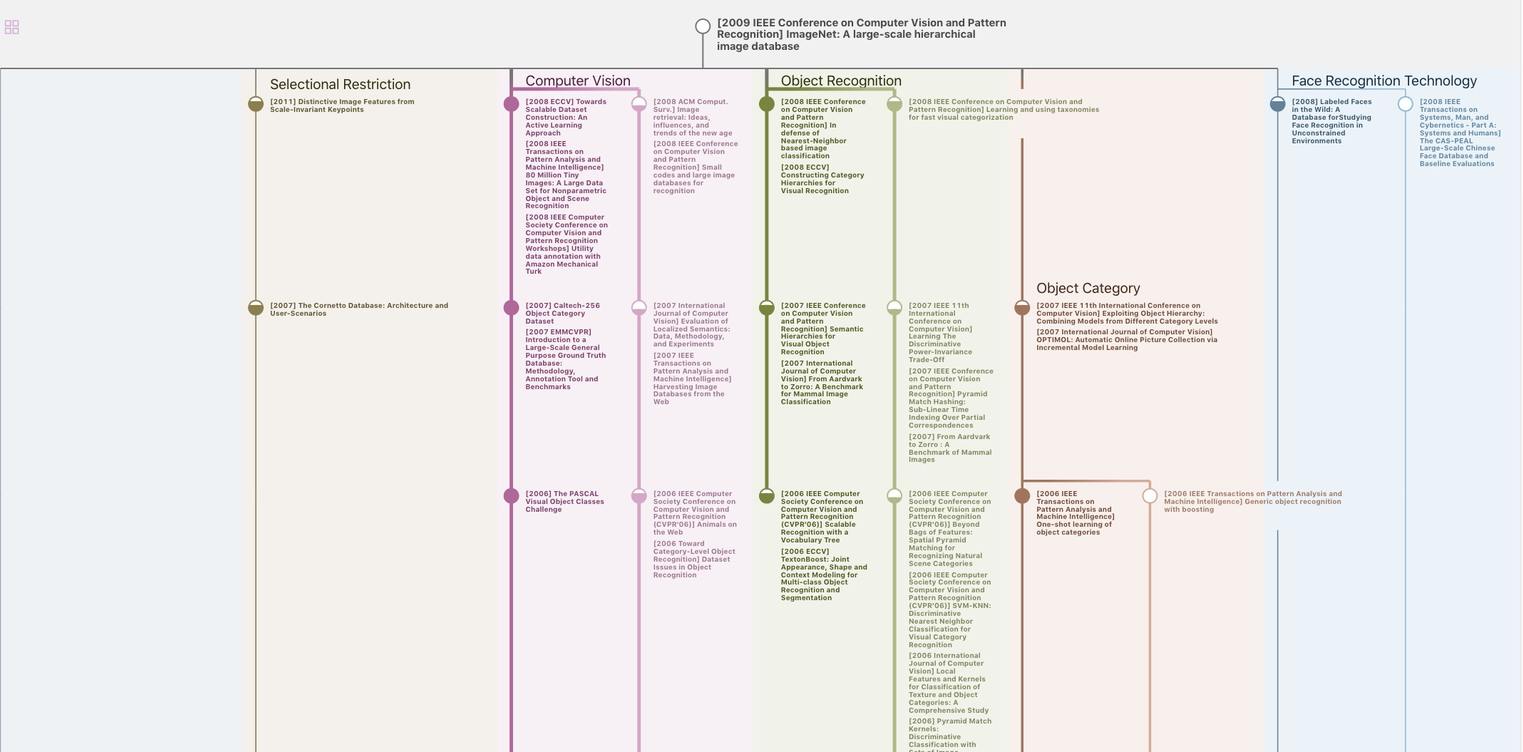
生成溯源树,研究论文发展脉络
Chat Paper
正在生成论文摘要