Investigating Multimodal Sensor Features Importance to Detect Agitation in People with Dementia
2023 IEEE Canadian Conference on Electrical and Computer Engineering (CCECE)(2023)
摘要
Neuropsychiatric Symptoms (NPS) are often manifested in People Living with Dementia (PwD), with agitation being one of the most common symptoms. Agitated behavior in PwD causes distress and raises the risk of injury to patients and caregivers. Therefore, detecting agitation events is essential for the safety of PwD and the people around them. AI-powered tools can monitor agitation behavior, alert care providers to instances of agitation, and help them respond quickly and effectively to improve the quality of life for PwD. Furthermore, research shows that selecting the proper set of features significantly affects the outcomes of a machine learning model and performance. This work investigates using a new set of features with various machine learning models to detect individual patterns of NPS. These features are extracted from sensor data collected by multimodal wearable devices from 17 PwDs admitted to a specialized Dementia Unit in Canada. Several machine learning models are trained using these features, and our findings show that Extra Trees achieves higher performance with the new feature set compared to the state-of-the-art feature set known to date. Performance evaluation shows that the new models successfully classified behavioral symptoms from personalized models with a median AUC of 0.941.
更多查看译文
关键词
Agitation,Dementia,Multi-modal sensors,Machine learning,Feature extraction,Exploratory Data Analysis
AI 理解论文
溯源树
样例
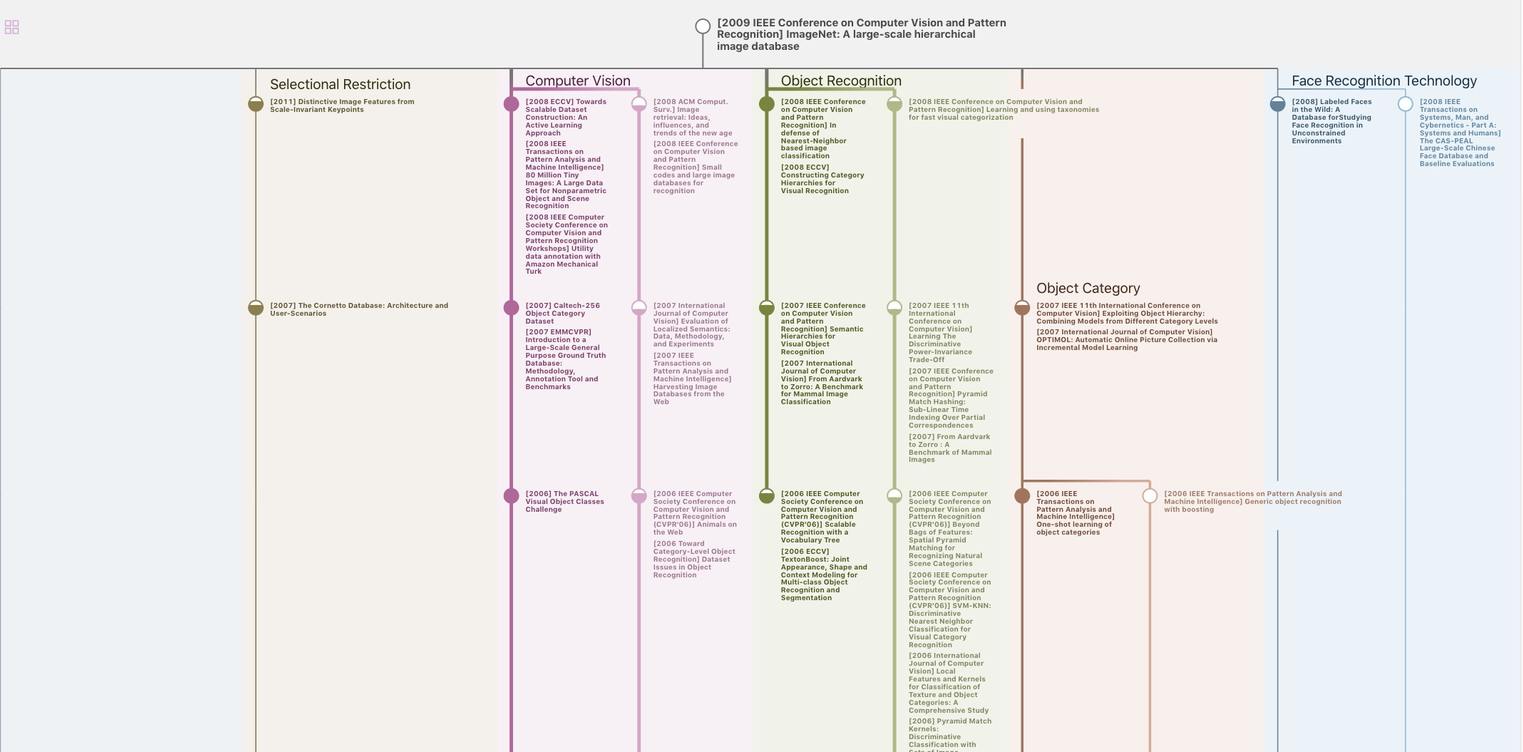
生成溯源树,研究论文发展脉络
Chat Paper
正在生成论文摘要