Digital Twin for Optimization of Slicing-Enabled Communication Networks: A Federated Graph Learning Approach
IEEE Communications Magazine(2023)
摘要
Slicing-enabled communication networks refer to a network architecture that enables the definition of multiple virtual networks or “slices” over a shared physical network. Each slice operates independently with its own dedicated resources, configuration, and management. However, this poses a major challenge in guaranteeing optimal resource allocation among those slices while preserving the obligatory Quality of Service (QoS) levels for each one. This study presents a federated learning-driven digital twin (DT) framework named FED-DT for creating a digital replica of the physical slicing-supported network to mimic its complicated infrastructure and forecast the network's dynamic performance. In FED-DT, the DT of network slicing is designated as non-Euclidean graph representations. A novel lightweight Graph Lineformer Network (GLN) is introduced to collaboratively learn and estimate QoS metrics from the topological structures of the underlying network slices. The FED-DT is empowered with an intelligent self-supervision method to improve generalizability on a large network, while Gaussian Differential Privacy (DP) is applied to guarantee the preservation of model privacy during training. Proof-of-concept simulations on different network topologies demonstrate the effectiveness of FED-DT in fulfilling rigid QoS requirements and achieving ideal performance.
更多查看译文
关键词
federated graph learning approach,communication networks,digital,slicing-enabled
AI 理解论文
溯源树
样例
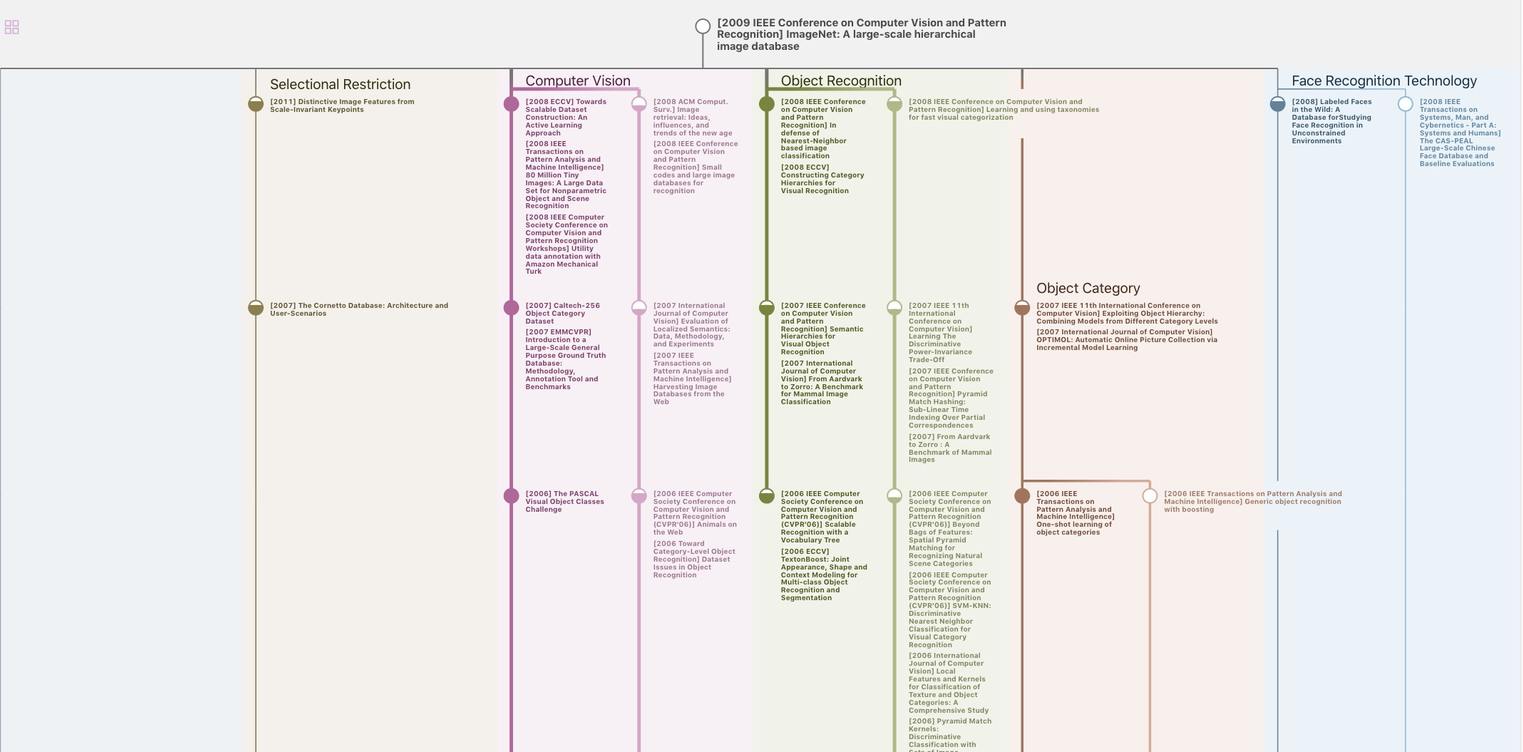
生成溯源树,研究论文发展脉络
Chat Paper
正在生成论文摘要