Analysing the drivers of ecological footprint in Africa with machine learning algorithm
ENVIRONMENTAL IMPACT ASSESSMENT REVIEW(2024)
摘要
Africa is largely confronted with varying issue of socioeconomic and insecurity, which is now increasingly linked with the mounting pressure on the continent's natural capital as glaring in the Sahel and Norther regions. As such, this study further probed the drivers of ecological footprint (EFP) by utilizing panel dataset of 39 African countries over the 1996 to 2018. Considering that several suspected drivers or covariates (which include the economy sectoral aspects, globalization aspects, governance and institutional aspects, energy types, and other socioeconomic aspects) of EFP were considered in the model, the feasibility of multicollinearity and other econometric drawbacks were accounted for by employing a two-step strategy. First, we used the least absolute shrinkage and selection operators (LASSOs) machine learning algorithms to predict the most important drivers of EFP. Second, we employed the Partialing-out LASSO instrumental variable regression (POIVLR) and Bayesian Model Averaging (BMA) techniques to obtain the variables marginal effects. The result found a more parsimo-nious regularization within the Adaptive LASSO (lambda = 0.0012), thus selecting 19 regressors out of the 26 cova-riates. Furthermore, the study found that Adaptive LASSO selection should be employed for inference since it performed better than the rest of the LASSOs and Elasticnet. As for the marginal effect of EFP, the partialing-out LASSO instrumental-variables regression (POIVLR) technique largely established expected impact of the selected covariates on EFP in Africa except for the impact of GDP and its square that shows negative and positive inference respectively. Combining these results and the sensitivity analysis, this study is poised to offer useful policy guide to environmentalist and decision-makers across Africa.
更多查看译文
关键词
Ecological footprint,Environmental sustainability,Bayesian moving average,Machine learning,Africa
AI 理解论文
溯源树
样例
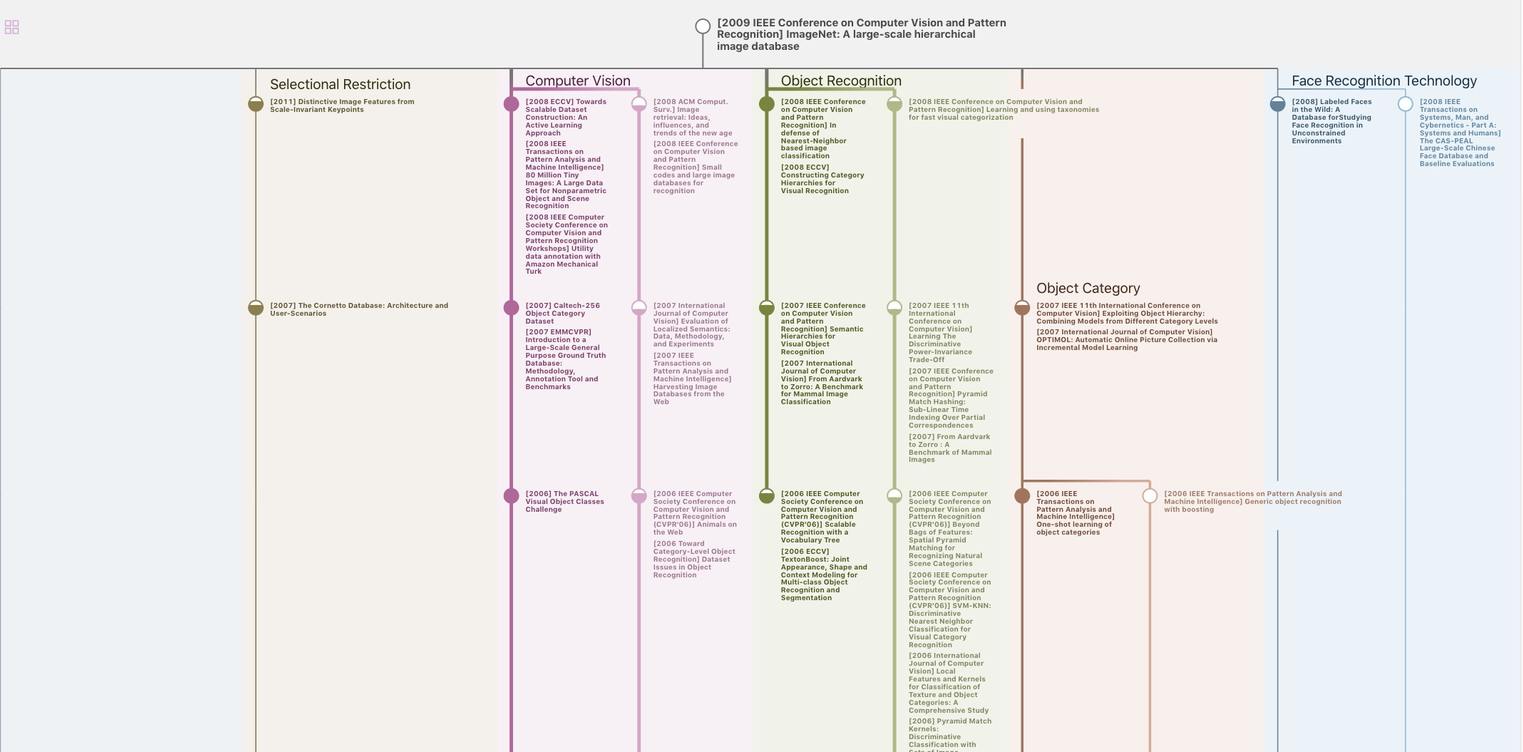
生成溯源树,研究论文发展脉络
Chat Paper
正在生成论文摘要