Prompt-based event relation identification with Constrained Prefix ATTention mechanism
Knowledge-Based Systems(2023)
摘要
Event Relation Identification (ERI) aims at mining the inter-event dependencies expressed in event-mentioned sentences. The main challenge of this task lies in recognizing the implicit clue for utterances without context words indicating the relation definitely. When confronting a lack of training samples, mainstream techniques fail to efficiently capture the subtle relations between events because the parameters of neural networks cannot be adequately fitted. Although there is a rising trend of using prompt learning to alleviate such issues, existing methods lack optimization of the prompt and prompts-tuning process. These deficiencies lead to two weaknesses: co-occurrence interference and amphibolous prompting. To this end, this paper proposes a Constrained Prefix ATTention mechanism (CPATT) and incorporates it into the traditional prompt-tuning process. In this fashion, our approach integrates context semantic features into dynamic prompts to mitigate co-occurrence interference. Moreover, CPATT supervises the guide effect of prompts via incorporating mutual exclusivity between categories into the loss function. The experimental results on two widely used datasets demonstrate that our method outperforms all state-of-the-art baselines, including GPT3.5-turbo, in terms of intra- and inter-sentence event relation identification tasks.
更多查看译文
关键词
Event relation identification,Prompt tuning,Pre-trained language model,Template generation,Information extraction
AI 理解论文
溯源树
样例
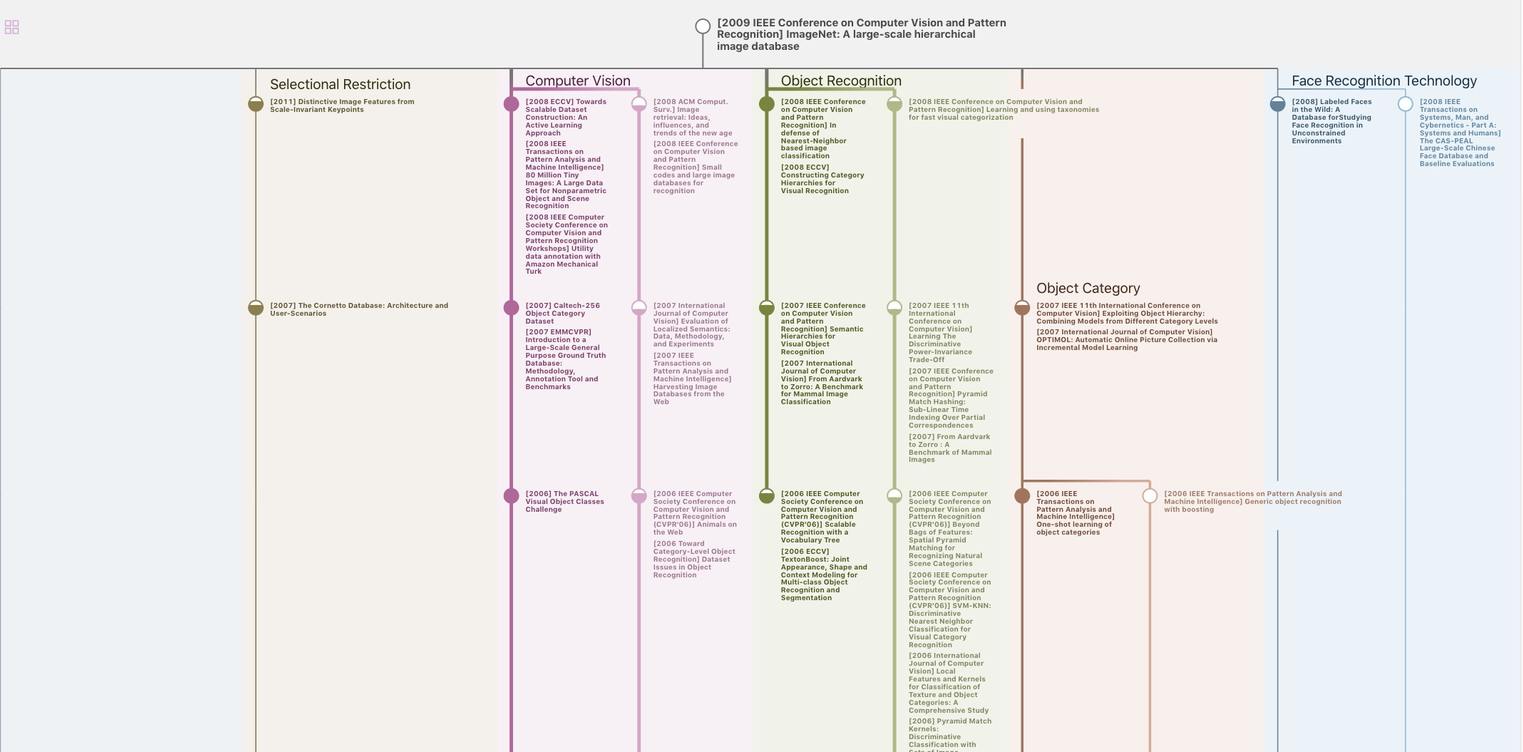
生成溯源树,研究论文发展脉络
Chat Paper
正在生成论文摘要