A frequency domain-based machine learning architecture for short-term wave height forecasting
Ocean Engineering(2023)
摘要
Accurate and reliable short-term waves forecasting plays a crucial role in ensuring the safety of offshore operations. However, the non-stationary and high-noise characteristics of wave height time series in real-world environments present significant challenges for prediction. This paper introduces a novel lightweight machine learning forecasting architecture, named FreMixer, that leverages frequency domain information. Specifically designed for sequences with a low signal-to-noise ratio (SNR), FreMixer utilizes a Gaussian filter block for noise reduction and a Fourier block for frequency domain feature extraction. The Mixer block, which incorporates skip-connections, serves as the key component for feature learning. Towing tank experiments demonstrate the superior performance of FreMixer compared to benchmark models such as the autoregressive moving average (ARMA), long short-term memory (LSTM), and extreme gradient boosting (XGBoost) models. Due to its simple structure, FreMixer offers low deployment costs and high training efficiency, making it an attractive option for practical applications. Furthermore, the impact of training set size and historical window size on the performance is discussed.
更多查看译文
关键词
Wave height, Short-term forecasting, Machine learning, Frequency domain, Multi-layer perceptron
AI 理解论文
溯源树
样例
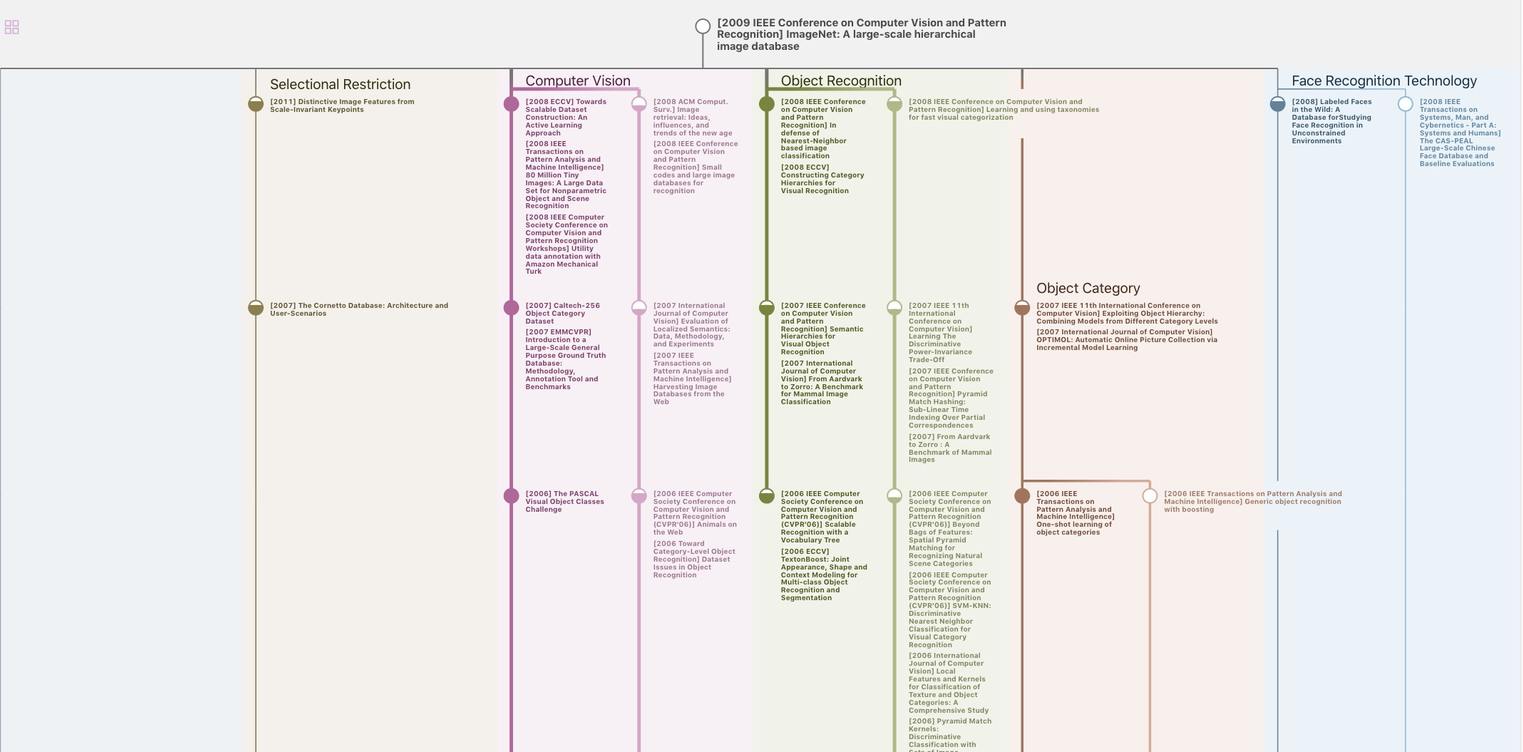
生成溯源树,研究论文发展脉络
Chat Paper
正在生成论文摘要