Efficient daily electricity demand prediction with hybrid deep-learning multi-algorithm approach
Energy Conversion and Management(2023)
摘要
Predicting electricity demand (G) is crucial for electricity grid operation and management. In order to make reliable predictions, model inputs must be analyzed for predictive features before they can be incorporated into a forecast model. In this study, a hybrid multi-algorithm framework is developed by incorporating Artificial Neural Networks (ANN), Encoder-Decoder Based Long Short-Term Memory (EDLSTM) and Improved Complete Ensemble Empirical Mode Decomposition with Adaptive Noise (ICMD). Following the partitioning of data, the G time-series are decomposed into multiple time-series using the ICEEMDAN algorithm, with partial autocorrelation applied to training sets to determine lagged features. We combine lagged inputs into a predictive framework where G components with the highest frequency are predicted with an ANN model, while remaining components are predicted with an EDLSTM model. To generate the results, all IMF components' predictions are merged using ICMD-ANN-EDLSTM hybrid models. A comparison is made between this objective model and standalone models (ANN, RFR, LSTM), hybrid models (CLSTM), and three decomposition-based hybrid models. Based on the results, the Relative Mean Absolute Error at Duffield Road substation was approximate to 2.82%, approximate to 4.15%, approximate to 3.17%, approximate to 6.41%, approximate to 6.60%, approximate to 6.49%, and approximate to 6.602%, compared to ICMD-RFR-LSTM, ICMD-RFR-CLSTM, LSTM, CLSTM, RFR, and ANN. According to statistical score metrics, the hybrid ICMD-ANN-EDLSTM model performed better than other benchmark models. Further, the results show that the hybrid ICMD-ANN-EDLSTM model can not only detect seasonality in G data, but also predict and analyze electricity market demand to add valuable insight to market analysis.
更多查看译文
关键词
Electricity demand prediction,Sustainable energy,Artificial intelligence,Deep learning,Encode-decoder architectures,Kernel density estimation
AI 理解论文
溯源树
样例
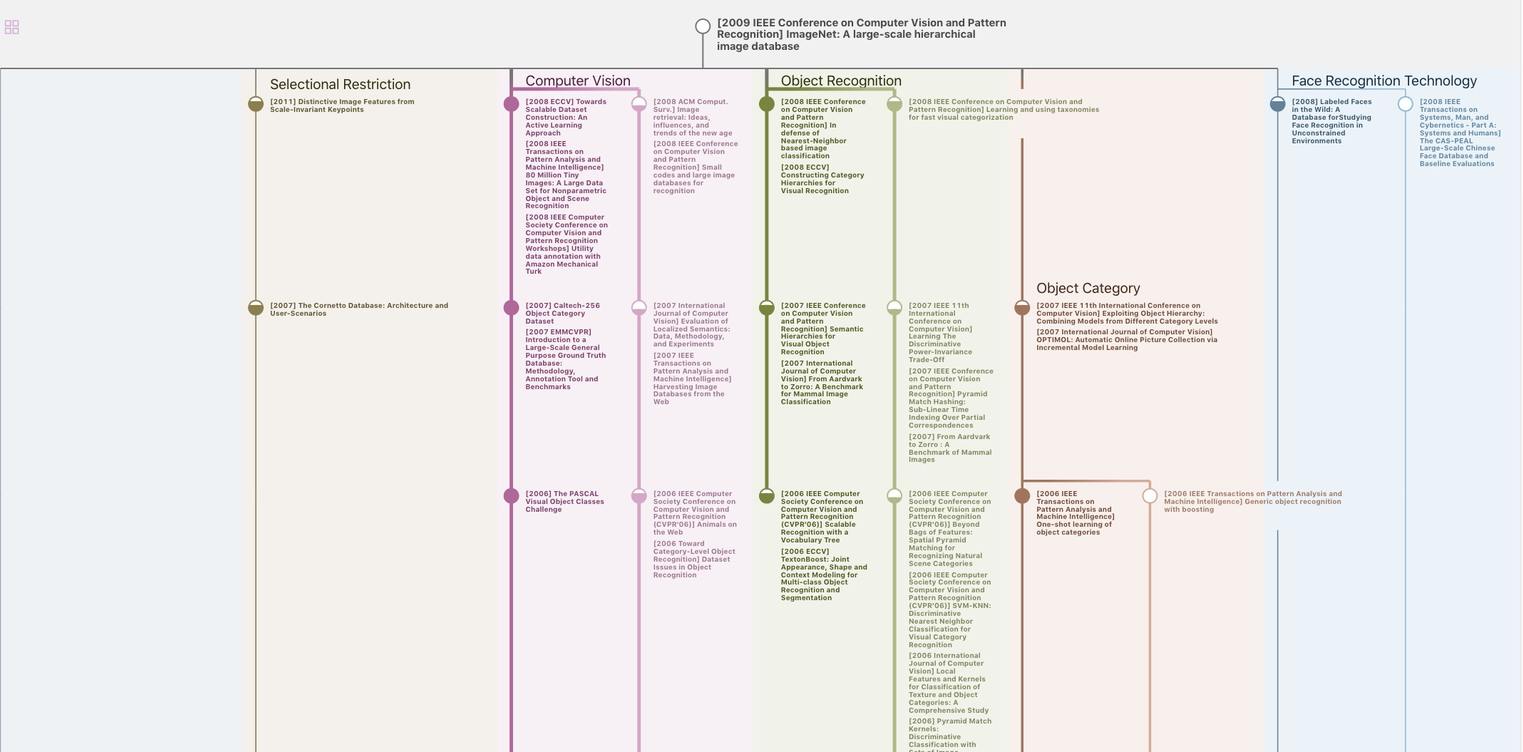
生成溯源树,研究论文发展脉络
Chat Paper
正在生成论文摘要