A Bayesian model calibration framework for stochastic compartmental models with both time-varying and time-invariant parameters
CoRR(2023)
摘要
We consider state and parameter estimation for compartmental models having both time-varying and time-invariant parameters. Though the described Bayesian computational framework is general, we look at a specific application to the susceptible-infectious-removed (SIR) model which describes a basic mechanism for the spread of infectious diseases through a system of coupled nonlinear differential equations. The SIR model consists of three states, namely, the three compartments, and two parameters which control the coupling among the states. The deterministic SIR model with time-invariant parameters has shown to be overly simplistic for modelling the complex long-term dynamics of diseases transmission. Recognizing that certain model parameters will naturally vary in time due to seasonal trends, non-pharmaceutical interventions, and other random effects, the estimation procedure must systematically permit these time-varying effects to be captured, without unduly introducing artificial dynamics into the system. To this end, we leverage the robustness of the Markov Chain Monte Carlo (MCMC) algorithm for the estimation of time-invariant parameters alongside nonlinear filters for the joint estimation of the system state and time-varying parameters. We demonstrate performance of the framework by first considering a series of examples using synthetic data, followed by an exposition on public health data collected in the province of Ontario.
更多查看译文
关键词
stochastic compartmental,bayesian model calibration framework,models,time-varying,time-invariant
AI 理解论文
溯源树
样例
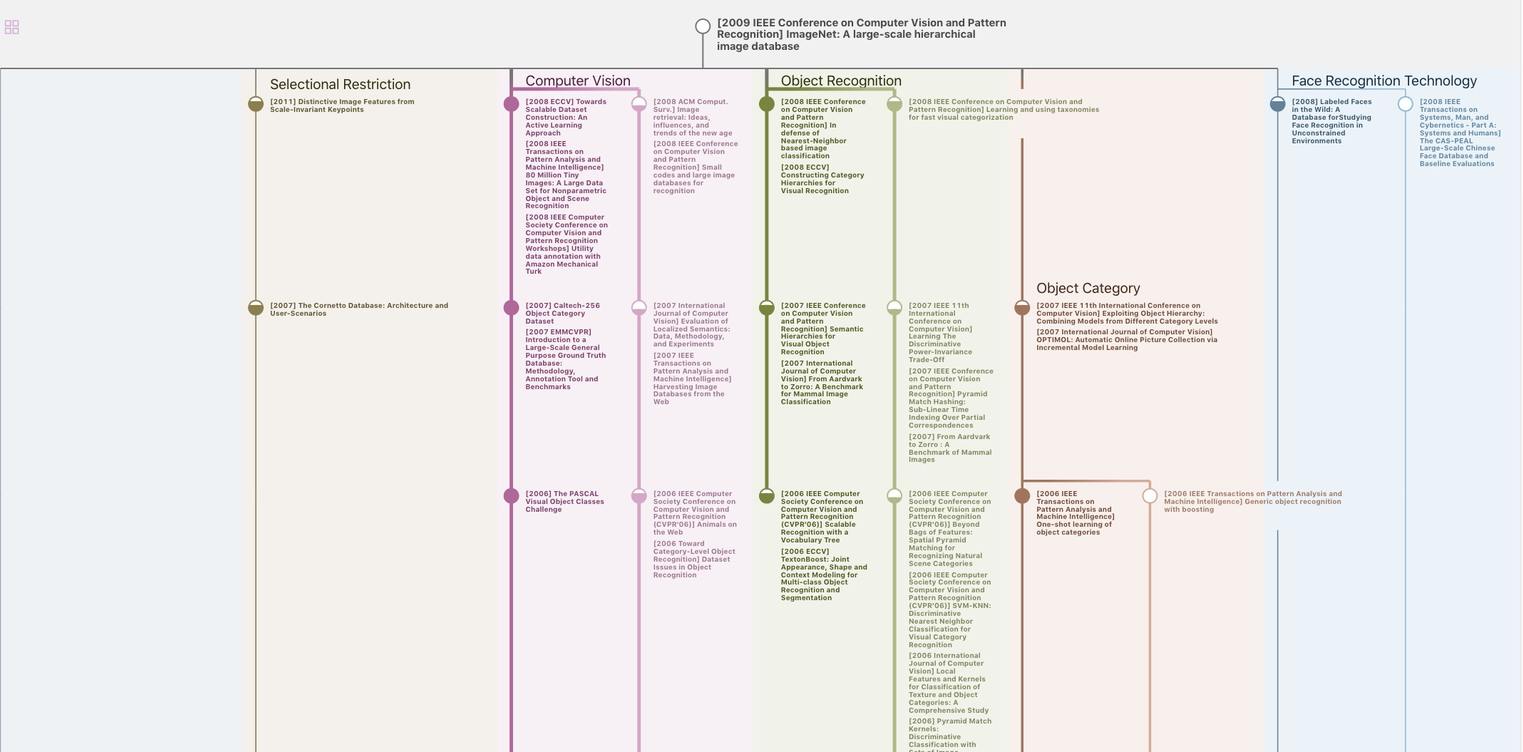
生成溯源树,研究论文发展脉络
Chat Paper
正在生成论文摘要