Deep Feature Registration for Unsupervised Domain Adaptation
CoRR(2023)
Abstract
While unsupervised domain adaptation has been explored to leverage the knowledge from a labeled source domain to an unlabeled target domain, existing methods focus on the distribution alignment between two domains. However, how to better align source and target features is not well addressed. In this paper, we propose a deep feature registration (DFR) model to generate registered features that maintain domain invariant features and simultaneously minimize the domain-dissimilarity of registered features and target features via histogram matching. We further employ a pseudo label refinement process, which considers both probabilistic soft selection and center-based hard selection to improve the quality of pseudo labels in the target domain. Extensive experiments on multiple UDA benchmarks demonstrate the effectiveness of our DFR model, resulting in new state-of-the-art performance.
MoreTranslated text
Key words
n/a
AI Read Science
Must-Reading Tree
Example
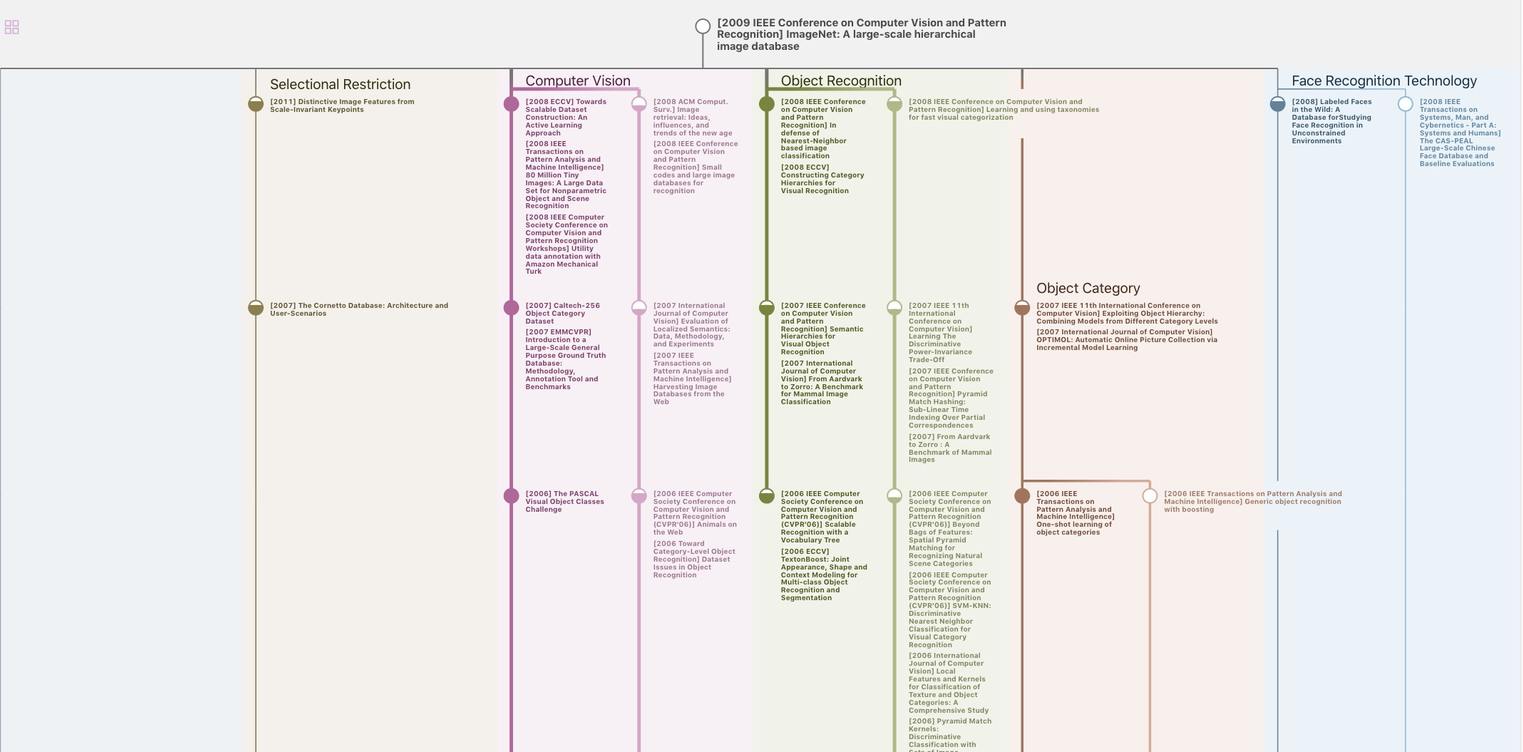
Generate MRT to find the research sequence of this paper
Chat Paper
Summary is being generated by the instructions you defined