Human Motion Prediction Based on Space-Time-Separable Graph Convolutional Network
IEEE Access(2023)
摘要
Human motion prediction is a popular method to predict future motion sequences based on past sequences, which is widely used in human-computer interaction. Space-time-separable graph Convolutional Network (STS-GCN) is a conventional mathematical model for human motion prediction. However, the uncertainty of human movements often leads to the problem of significant prediction error in the prediction results. This paper first proposed a Multi-scale STS-GCN (MSTS-GCN) model based on the conventional STS-GCN method to find the relevant factors that affect the prediction results. In our study, the constructed Multi-scale Temporal Convolutional Network (MTCN) decoder effectively reduced the human motion prediction error at specific time nodes. To expand the transmission and utilization performance in a larger receptive field, a Gated Recurrent Unit-TCN decoder was also designed. Finally, a new STS-GCN (NSTS-GCN) human motion prediction model was proposed, which realized the transmission and utilization of motion sequence features under a larger temporal perceptual field. To verify the effectiveness of NSTS-GCN, the Human3.6M dataset, AMASS, and 3DPW dataset were tested. The experimental results show that the MPJPE error of the proposed model for human joint prediction at each time node is reduced compared with the conventional STS-GCN model, and the mean reduction was achieved by 3.0mm. All the experimental results validated the effectiveness of the proposed NSTS-GCN model, which further improved the performance of human motion prediction.
更多查看译文
关键词
human motion prediction,graph,space-time-separable
AI 理解论文
溯源树
样例
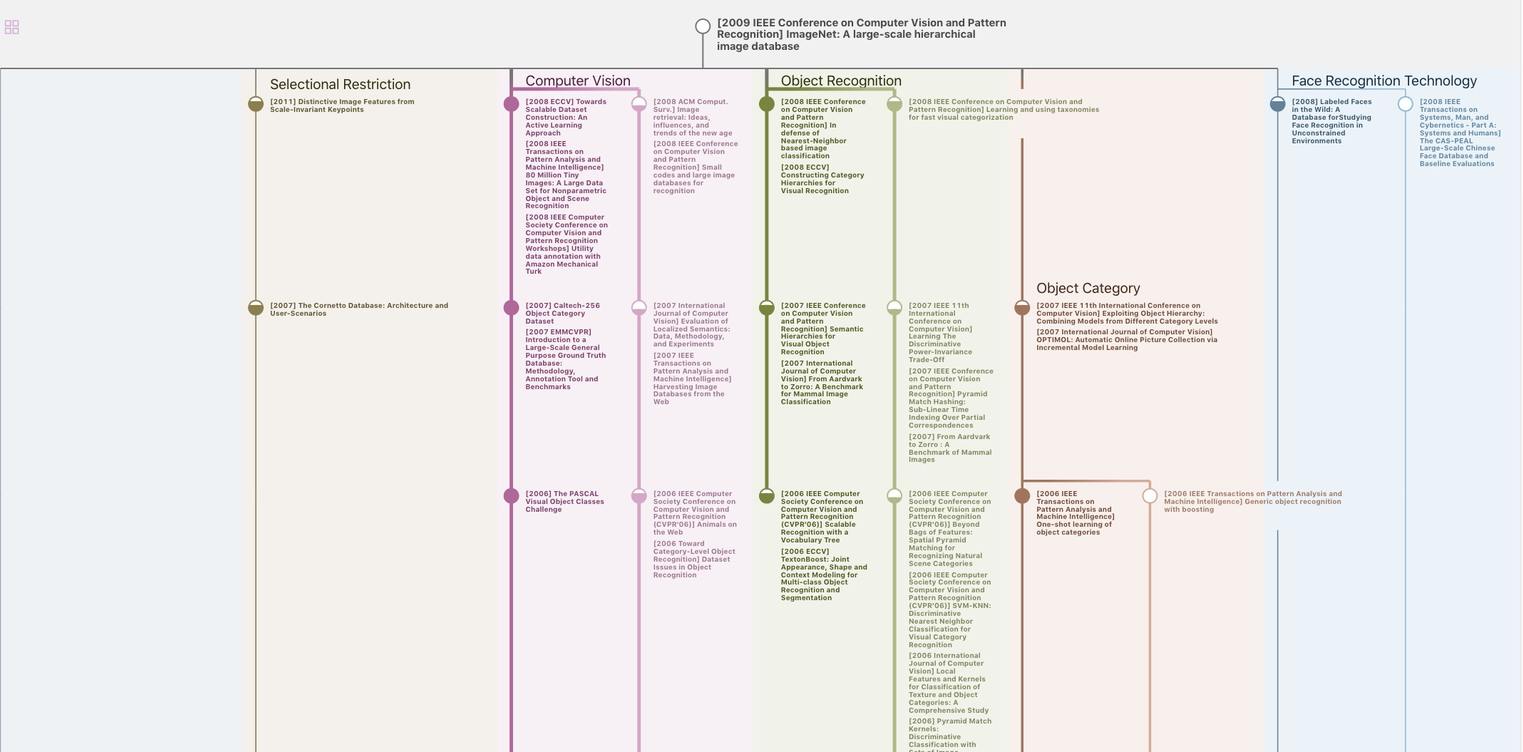
生成溯源树,研究论文发展脉络
Chat Paper
正在生成论文摘要