Texture and CNN Based Doppler Signal Classification
2023 8th International Conference on Computer Science and Engineering (UBMK)(2023)
摘要
Background understanding and extraction from images becomes easy when different image descriptors capture the location and movement of different sub-elements in a given image. One main type of artificial neural network called Convolutional neural network (CNN) has attracted lot of interest in bio-medical sub-domain. Depending on the type and amount of data, its different architectures lead to its heterogeneous learning capability. Cerebral emboli detection is considered as one of the main problems in understanding the nature of normal and abnormal blood flow. Embolus detection using transcranial Doppler ultrasound is a useful method for the identification of active embolic sources in cerebrovascular diseases. Texture features represent the lower space of image data and encode the relationship of different pixel values. Local Derivative pattern (LDP) calculates the n-th order derivative directions of each pixel in an image. In this paper, we make use of labelled Doppler signal dataset collected from patients with carotid stenosis and make use of combination of CNN layers and LDP operators for Doppler signal classification. We convert Doppler signals into image data and add LDP feature extraction as a layer in CNN. In a single mode CNN-LDP, network on our Doppler image data achieved validation accuracy of 90% with a loss of 21.91% (Batch size of 64, epochs 10). In multi-mode CNN-LDP, network achieved validation accuracy of 91% with a loss of 20.64% (Batch-size 64 epochs 8).
更多查看译文
关键词
Convolutional Neural Networks,Doppler signal,Local Binary Pattern,Local Derivative pattern
AI 理解论文
溯源树
样例
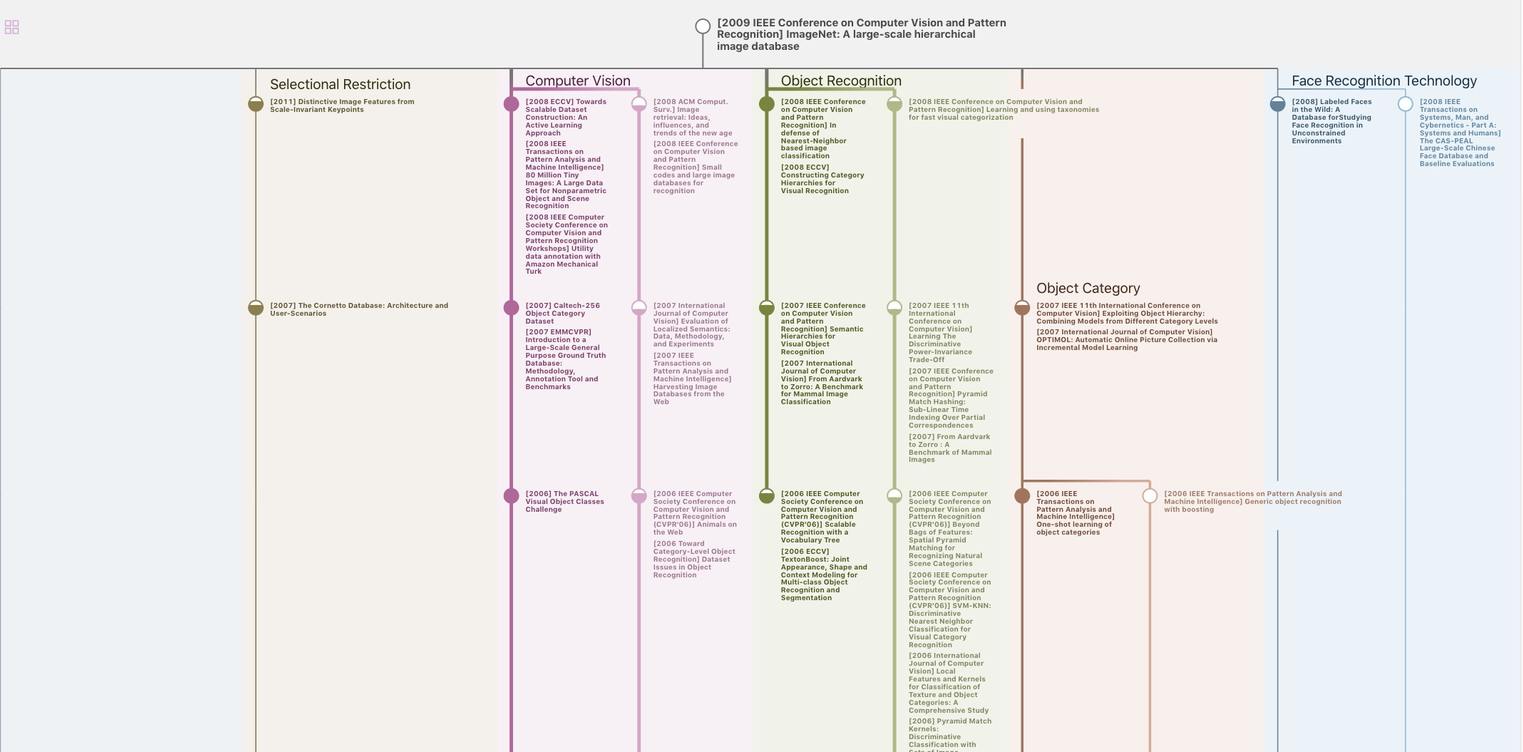
生成溯源树,研究论文发展脉络
Chat Paper
正在生成论文摘要