Locswinunet: A Neural Network for Urban Wireless Localization Using TOA and RSS Radio Maps
2023 IEEE 33rd International Workshop on Machine Learning for Signal Processing (MLSP)(2023)
摘要
In outdoor environments, Global Navigation Satellite Systems (GNSS) is usually the preferred mode for localization of a user equipment (UE). The accuracy of GNSS localization depends on the line-of-sight (LoS) conditions between the ground UE and the GNSS satellites, and hence it often does not perform well in urban environment with building blockages. In such situations, user would have to rely on the widely deployed mobile network and use signal parameters such as received signal strength (RSS) and time of arrival (ToA) measured from several base stations (BS) for localization. However, the localization accuracy is still limited by the dense multipath and non-line-of-sight (NLoS) propagations. Radio maps which provide extensive location specific RF signatures can be a promising solution to overcome these limitations. In this paper, we present a novel neural network, LocSwinUNet, which utilizes the RSS and ToA measurements made by the UE and the corresponding radio maps of the BSs to perform the localization task. The proposed network achieves RMSE localization errors of 4.398 meters with clean measurements and 10.140 meters when the RSS and ToA measurements are distorted by Gaussian noises with σ
p
= 10 dB and σ
t
= 10 meters, respectively. Our method shows robustness across different realistic scenarios modelled by various Gaussian noises. The code is available at https: //github.com/mhh0318/WirelessLocation.
更多查看译文
关键词
Received signal strength (RSS),time-of arrival (ToA),radio map,urban localization
AI 理解论文
溯源树
样例
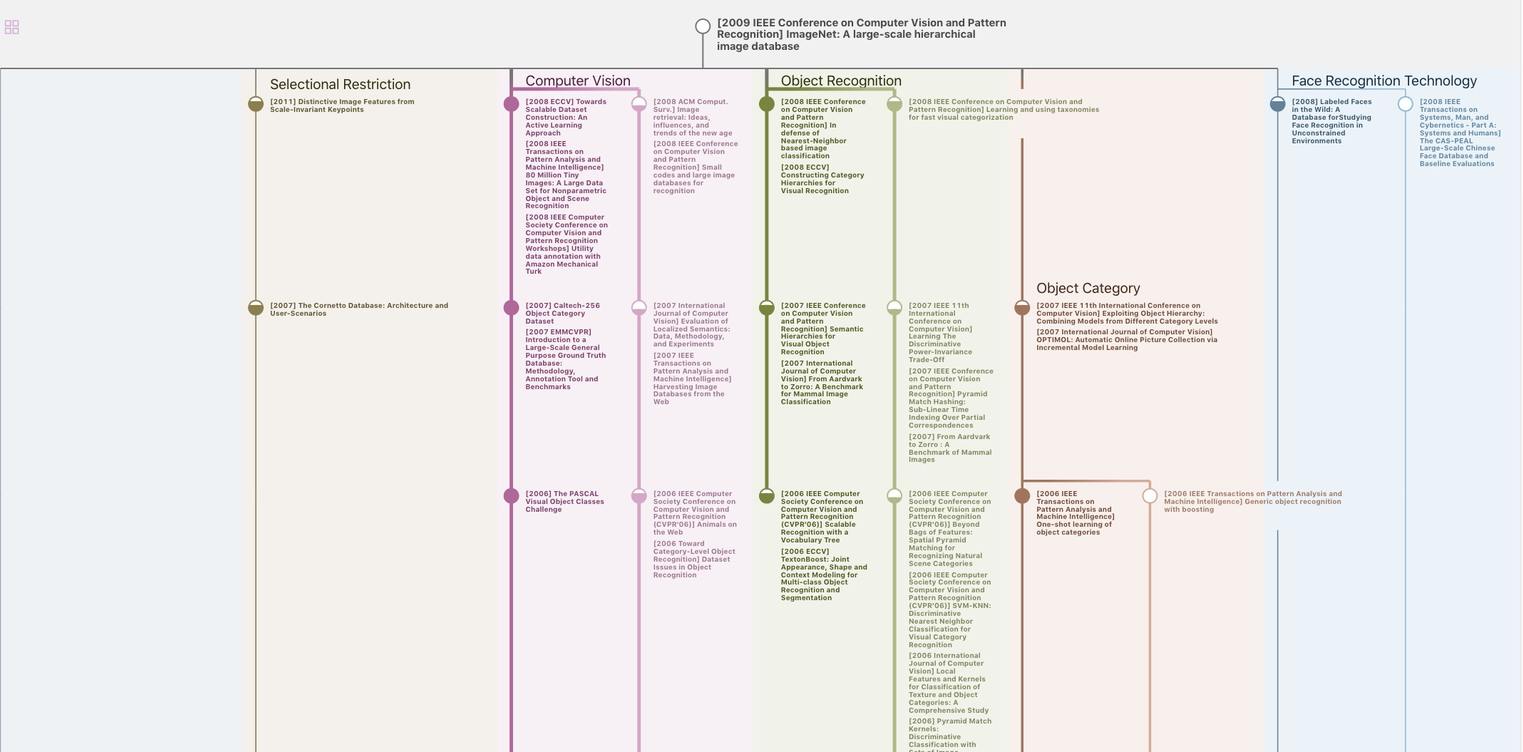
生成溯源树,研究论文发展脉络
Chat Paper
正在生成论文摘要