Predicting Room Impulse Responses Through Encoder-Decoder Convolutional Neural Networks
2023 IEEE 33rd International Workshop on Machine Learning for Signal Processing (MLSP)(2023)
摘要
This paper investigates the ability of deep neural networks (DNNs) to predict a specific room impulse response (RIR) between two given room locations. We use three end-to-end deep learning (DL) models based on the encoder-decoder structure: an auto-encoder (AE), a variational AE (VAE) and a UNet. They try to generate a new RIR given: 1) the short-time Fourier transform (STFT) of a true RIR from the same room, 2) the spatial coordinates of the true and new RIRs, and 3) several room-related parameters. On the one hand, the magnitude and phase of the STFT are computed and presented to the DNN input. On the other hand, the spatial coordinates and the room parameters form an information vector that is embedded into the DNNs through their latent space (AE, VAE) or equivalent layer (UNet). A real database of RIRs measured in five different rooms was used to train and test the models. Two experiments were carried out to study the influence of the magnitude and phase terms of the loss function on the performance of the models. An additional experiment investigated the ability of the DL models to generalize across rooms. Results show that the three DNNs are able to predict the magnitude of the STFT, but they cannot accurately predict its phase. When comparing their ability to generalize across spaces, the UNet achieves the best results.
更多查看译文
关键词
Room impulse response modeling,deep learning,neural networks
AI 理解论文
溯源树
样例
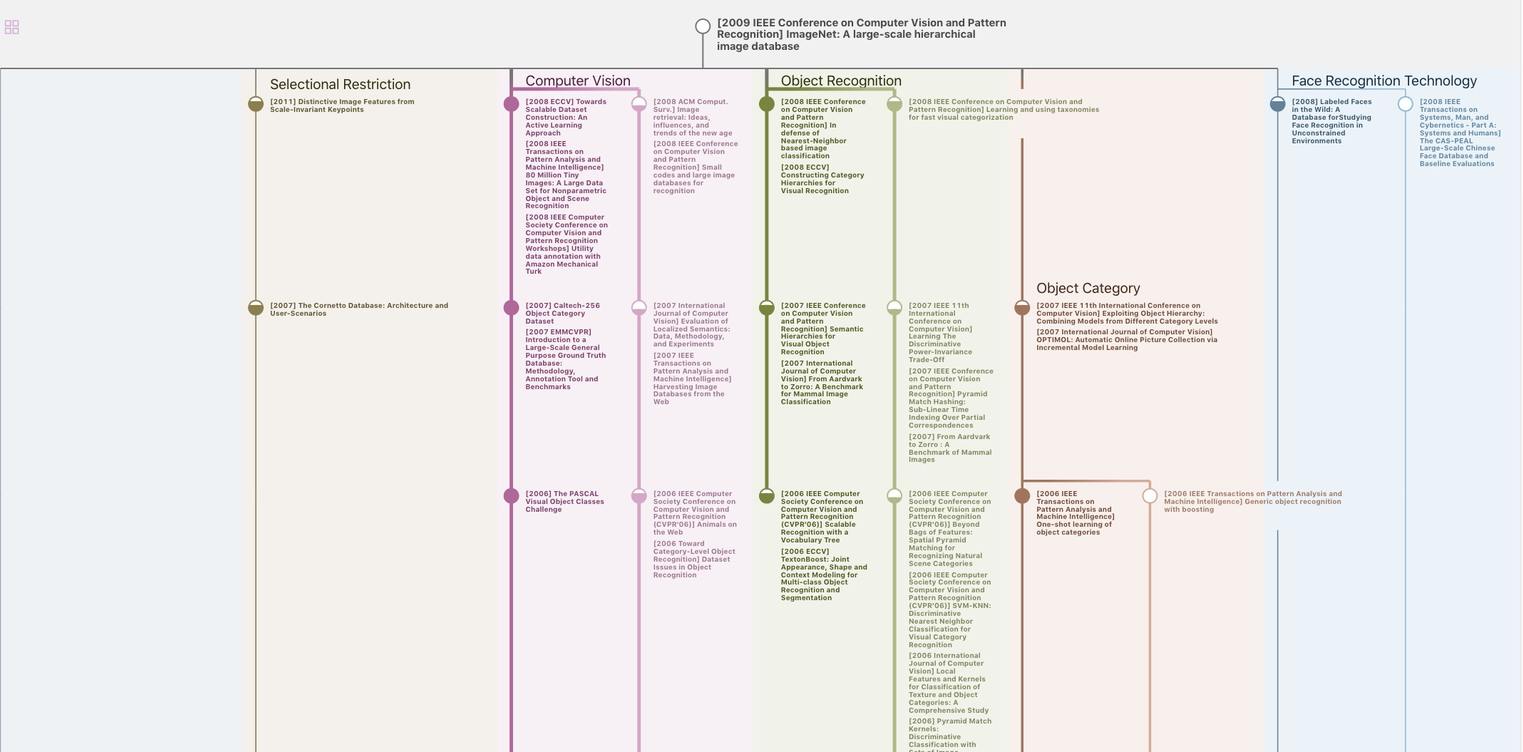
生成溯源树,研究论文发展脉络
Chat Paper
正在生成论文摘要