Low-Complexity Streaming Speech Super-Resolution
2023 IEEE 33rd International Workshop on Machine Learning for Signal Processing (MLSP)(2023)
摘要
Speech super-resolution is the process of estimating the missing frequency content of a speech signal from its existing band-limited frequency content. The loss of frequency components is a common occurrence that can be because of a low sampling rate, low-quality microphones, or various transmission factors, and it is an increasingly common problem as bandwidth for high-quality communications is generally available, but many end devices are still using older standards and protocols. Although a number of solutions exist for this problem, we note that most are not amenable to real-world use, due to computational or algorithmic constraints. In this paper we present a compact, efficient, and minimal-latency solution to speech super-resolution that is suitable for use with real-time streaming data. We propose a novel causal architecture that can be easily deployed for real-world use. We additionally propose a novel adversarial training process and an initialization procedure that speeds up convergence and results in improved outputs. Objective and subjective results show that our proposed model outperforms the latest solutions in this space, despite being significantly smaller and faster.
更多查看译文
关键词
speech super-resolution,bandwidth extension,speech synthesis
AI 理解论文
溯源树
样例
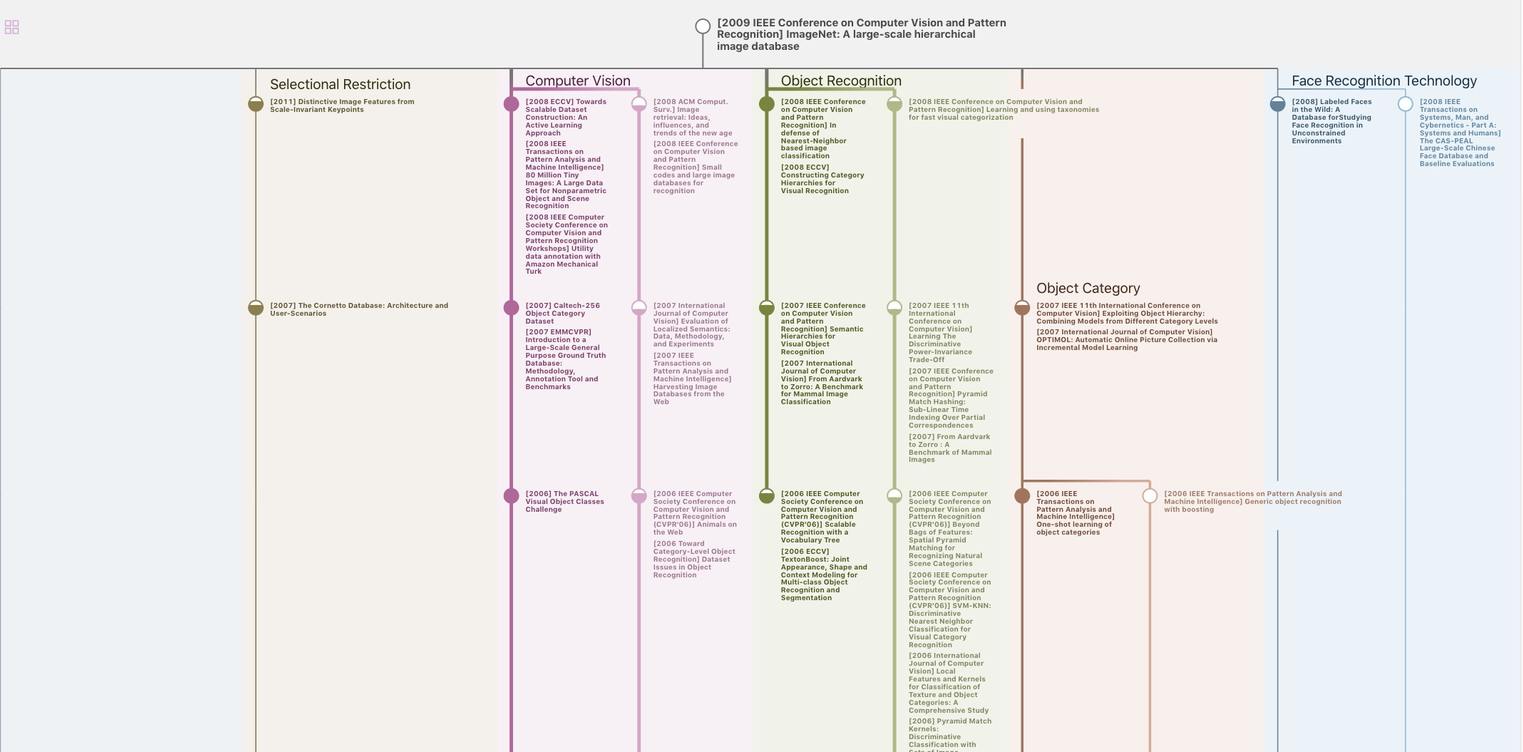
生成溯源树,研究论文发展脉络
Chat Paper
正在生成论文摘要