Personalized Differentially Private Federated Learning without Exposing Privacy Budgets
PROCEEDINGS OF THE 32ND ACM INTERNATIONAL CONFERENCE ON INFORMATION AND KNOWLEDGE MANAGEMENT, CIKM 2023(2023)
摘要
The meteoric rise of cross-silo Federated Learning (FL) is due to its ability to mitigate data breaches during collaborative training. To further provide rigorous privacy protection with consideration of the varying privacy requirements across different clients, a privacy-enhanced line of work on personalized differentially private federated learning (PDP-FL) has been proposed. However, the existing solution for PDP-FL [21] assumes the raw privacy budgets of all clients should be collected by the server. These values are then directly utilized to improve the model utility via facilitating the privacy preferences partitioning (i.e., partitioning all clients into multiple privacy groups). It is however non-realistic because the raw privacy budgets can be quite informative and sensitive. In this work, our goal is to achieve PDP-FL without exposing clients' raw privacy budgets by indirectly partitioning the privacy preferences solely based on clients' noisy model updates. The crux lies in the fact that the noisy updates could be influenced by two entangled factors of DP noises and non-IID clients' data, leaving it unknown whether it is possible to uncover privacy preferences by disentangling the two affecting factors. To overcome the hurdle, we systematically investigate the unexplored question of under what conditions can the model updates of clients be primarily influenced by noise levels rather than data distribution. Then, we propose a simple yet effective strategy based on clustering the L-2 norm of the noisy updates, which can be integrated into the vanilla PDP-FL to maintain the same performance. Experimental results demonstrate the effectiveness and feasibility of our privacy-budget-agnostic PDP-FL method.
更多查看译文
关键词
Differential Privacy,Federated Learning,Personalized Privacy
AI 理解论文
溯源树
样例
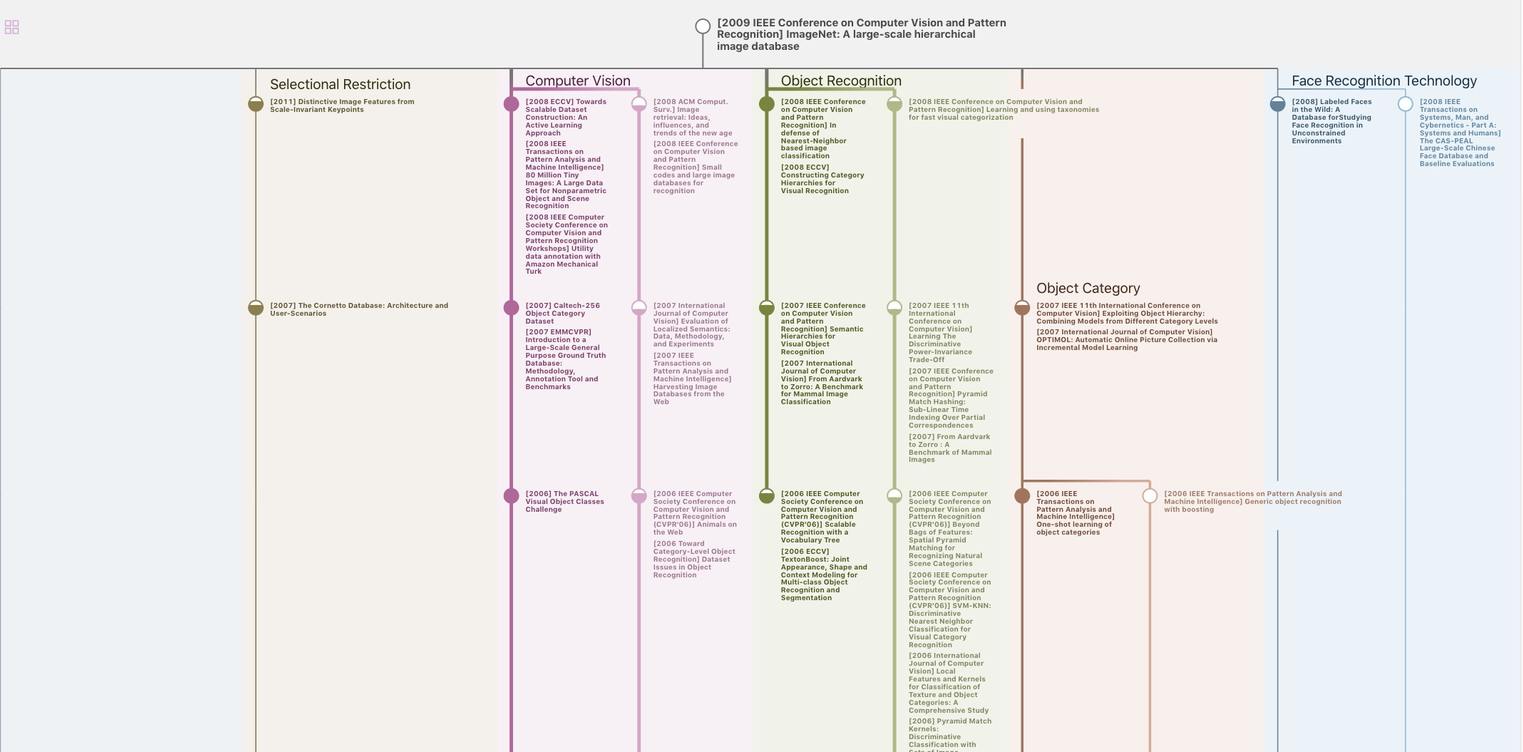
生成溯源树,研究论文发展脉络
Chat Paper
正在生成论文摘要