Aggregated-attention deformable convolutional network for few-shot SAR jamming recognition
PATTERN RECOGNITION(2024)
摘要
This work simultaneously addresses the challenges of unseen classes and low-data problems on synthetic aperture radar jamming recognition (SAR-JR). Currently, very few studies have tackled both challenges. Inspired by the success of few-shot learning, which learns a robust model from a few instances, we formulate SAR-JR as a few-shot task in a metric-learning framework to alleviate the above challenges. Against the jamming features with significant dispersion and complex geometric transformations, as well as feature obscuration in time-frequency images (TF), we propose an aggregated-attention deformable convolutional network (A2-DCNet) framework consisting of an aggregated-attention deformable convolutional module (A2 -DC-Module) and a prototype classification module based on polynomial loss (PolyLoss-PC-Module). The former learns informative and refined embeddings from the TF images, while the latter performs the SAR-JR in an embedding space by calculating distances to prototypes of each class. Specifically, the modulated deformable convolution of the A2-DC-Module can capture long-range spatial contextual information from a global perspective, while the aggregated attention is designed to refine the representations of obscured features in the TF images. To further optimize the framework, we introduce a novel PolyLoss and customize the optimal form for our model to learn an embedding space with robust inter-class separability. Finally, to realize few-shot SAR-JR tasks, we simulate a novel dataset called JamSet. Extensive experimental results on our dataset have demonstrated substantial improvement of our proposed A2-DCNet method over the benchmarks.
更多查看译文
关键词
Few-shot learning,Modulated deformable convolution,Aggregated attention,SAR jamming recognition
AI 理解论文
溯源树
样例
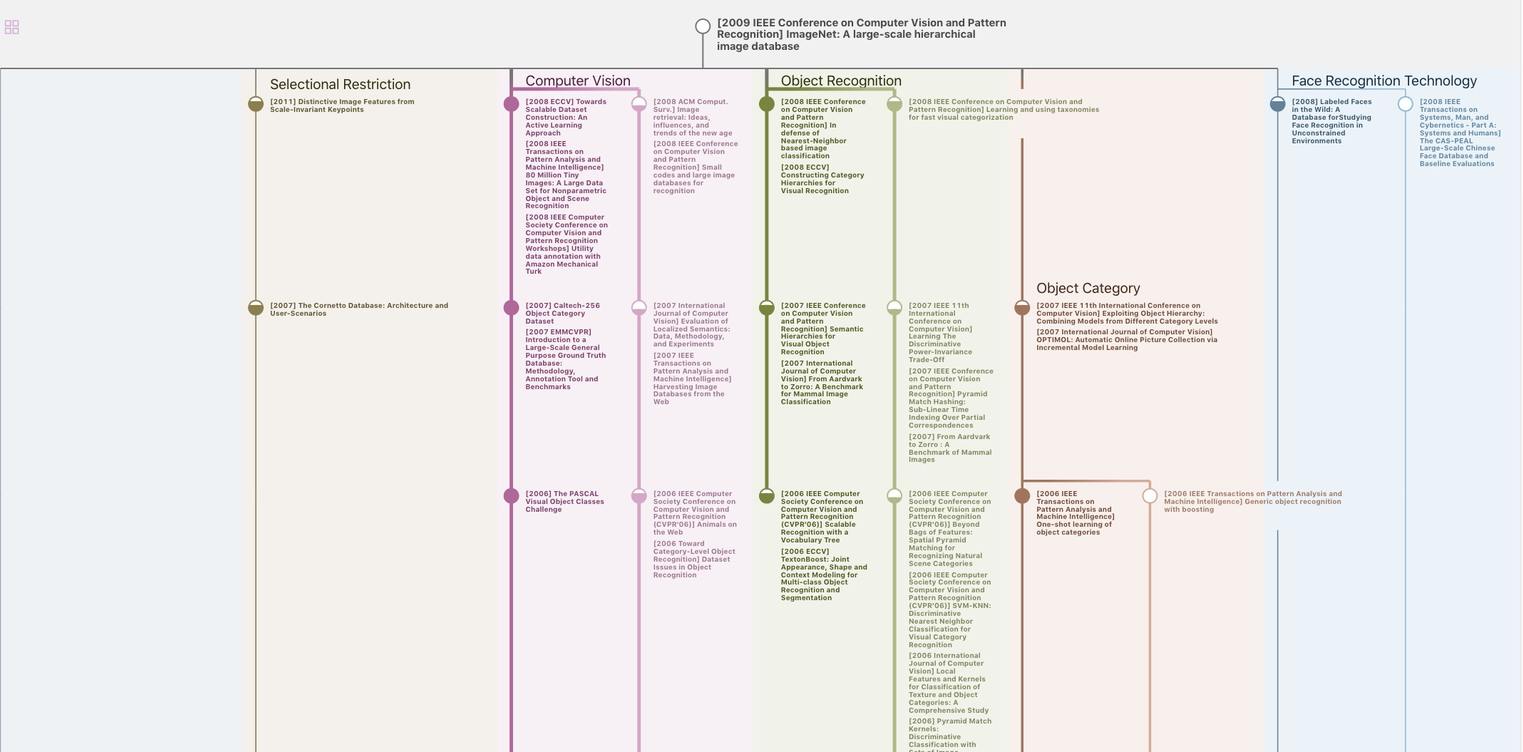
生成溯源树,研究论文发展脉络
Chat Paper
正在生成论文摘要