Research on dynamic decision-making for product assembly sequence based on Connector-Linked Model and deep reinforcement learning
Journal of Manufacturing Systems(2023)
摘要
Assembly sequence planning (ASP) is a critical challenge in the manufacturing industry, involving the arrangement of components to maximize production efficiency and reduce costs. Solving the ASP problem is not only hindered by its NP-hard nature, making it difficult to obtain optimal solutions, but also requires making rapid decisions in the face of dynamic changes in resource availability during the actual assembly process. This paper proposes a Deep reinforcement learning (DRL) approach to tackle the ASP problem. DRL learns optimal assembly strategies through interactions between an intelligent agent and its environment, using a reward function as a long-term objective to guide decision-making, ultimately enabling the acquisition of near-optimal solutions. DRL exhibits high scalability and reusability, leveraging previously acquired knowledge to adapt to dynamic changes in the assembly environment. First, a connector-based graph structure is introduced to decompose assembly products into a series of connector-based assembly elements. These elements are then transformed into a matrix format for use as input to the DRL method. Second, an encoder-decoder architecture tailored to the ASP problem is developed. This architecture utilizes mask mechanism to ensure that the generated assembly sequences adhere to assembly priority constraints while adapting to dynamic changes in resource availability during assembly. Finally, policy gradient methods are employed to train the DRL model, enabling it to generate assembly sequences with lower costs and higher efficiency. Experimental results demonstrate the effectiveness of the proposed approach. Furthermore, compared to heuristic algorithms, DRL exhibits faster decision-making capabilities and better adaptability to dynamic resource changes during the assembly process.
更多查看译文
关键词
Assembly sequence planning, Deep reinforcement learning, Combinatorial optimization, Connector-Linked Model
AI 理解论文
溯源树
样例
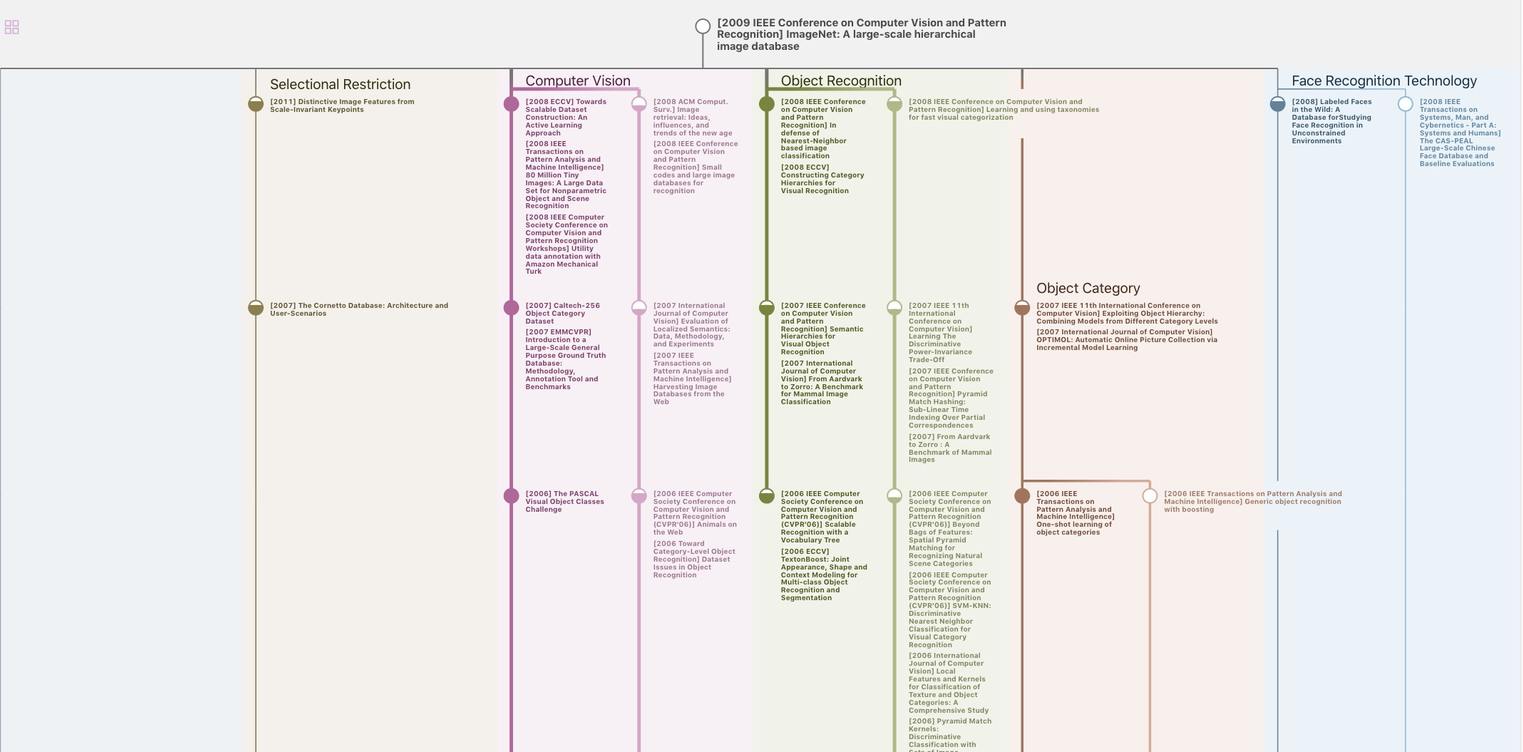
生成溯源树,研究论文发展脉络
Chat Paper
正在生成论文摘要