A Reconstruction Algorithm for Cherenkov-Excited Luminescence Scanning Imaging Based on Unrolled Iterative Optimization
CHINESE JOURNAL OF LASERS-ZHONGGUO JIGUANG(2023)
摘要
Objective Cherenkov-excited luminescence scanning imaging (CELSI) is an emerging optical imaging technology that provides a new tool for tumor diagnosis and treatment. However, CELSI image reconstruction is ill-posed and underdetermined because of lightscattering in biological tissues and limited boundary measurements. Regularization techniques have been widely adopted to alleviate the ill-posedness of the CELSI reconstruction. However, these methods typically exhibit poor image quality. To date, deep-learning-based reconstruction algorithms have attracted significant attention in optical tomography. To enhance the image quality of CELSI, we develop a reliable and effective deep learning reconstruction algorithm based on unrolled iterative optimization. Methods In this paper, a deep learning reconstruction algorithm is introduced based on unrolled iterative optimization, which takes the acquired sinogram image as network input and directly outputs the high-quality reconstructed images through end-to-end training. First, the image reconstruction of CELSI is reformulated as a l1 norm optimization problem based on sparse regularization technique. Second, the alternating direction method of multipliers (ADMM) based neural network algorithm (ADMM-Net) is adopted to minimize the optimization problem, which converts each iteration into convolution neural network (CNN) processing layer and deploys multiple processing layers cascaded into a deep network. Each processing layer consists of a reconstruction layer, a nonlinear layer, and a multiplier update layer. We linearize the reconstruction layer to avoid matrix inversion. The nonlinear transformation function in the nonlinear layer consists of five convolutional operators with three rectified linear unit (ReLU). The first convolution operator comprises 32 filters with the size of 3 x 3, and the other convolution operators consist of 32 filters with the size of 3 x 3 x 32. Note that all the parameters in the ADMM-Net are end-to-end updated through gradient backpropagation, including the step size and regularization parameter in the reconstruction layer, the shrinkage threshold in the nonlinear layer, as well as the nonlinear transformation function. To evaluate the effectiveness of ADMM-Net, numerical simulation experiments were performed, and the performance was compared with the traditional FBP algorithm, two deep learning algorithms (FBPConvNet and ISTA-Net+). Root mean square error (RMSE), peak signal-to-noise ratio (PSNR), and structural similarity (SSIM) are used as quantitative metrics. Results and Discussions First, the influence of the number of layers on the reconstruction performance is evaluated. Our results show that a better image quality can be obtained when the number of layers increases (Table 1). However, a large number of layers increases the computational cost. To balance the quality of the reconstructed images and computational cost, the number of layers is chosen empirically as five. Furthermore, experiments are performed using a single fluorescent target. Compared with the other three algorithms, reconstructed images with fewer artifacts are obtained for the proposed ADMM-Net (Fig. 5). The quantitative results show that the average values of PSNR and SSIM yielded by our algorithm are 33.75 dB and 0.86, respectively, and compared with the other three algorithms, ADMM-Net achieves the average reduction of 81.5%, 16.3%, and 25.2% in RMSE, improvement of 77.8%, 5.1%, and 8.6% in PSNR, and increases of 47.2%, 4.5%, and 2.7% in SSIM (Table 2). The ability of ADMM-Net to distinguish between two targets is also tested. When the edge-to-edge distance between the two fluorescent targets is 2 mm, the FBP, FBPConvNet, and ISTA-Net+ algorithms fail to separate the two fluorescent targets, whereas ADMM-Net successfully distinguishes the two fluorescent targets (Fig. 8). The plot of the profiles again confirmed that ADMM-Net can achieve satisfactory results in terms of the reconstructed positions, sizes, and quantum yields of the fluorescent targets (Fig. 9). Finally, the generalizability of ADMM-Net is verified by reconstructing three and four fluorescent targets with different ratios of fluorescence quantum yields. Our results reveal that the FBP, FBPConvNet, and ISTA-Net+ algorithms cannot accurately recover the distribution of fluorescent targets. In contrast, the proposed algorithm exhibits a good generalization performance and can accurately recover the distributions of three and four targets (Fig. 11). Conclusions This paper proposes a deep learning reconstruction algorithm for CELSI based on unrolled iterative optimization (ADMM-Net), which combines the structure of the iterative update steps of the ADMM algorithm with a CNN. The performance is evaluated through numerical simulation experiments. As a result, compared with the FBP, FBPConvNet, and ISTA-Net+ algorithms, the proposed algorithm can yield better results with fewer artifacts and higher quantitative accuracy when reconstructing single fluorescent targets with different contrasts. Moreover, the proposed algorithm can distinguish between two fluorescent targets with high image quality, even when the edge-to-edge distance is 2 mm. Our results also demonstrate that our algorithm has a good generalization performance and can reconstruct three and four fluorescent targets accurately, even when the model is trained on a dataset with only one or two targets.
更多查看译文
关键词
medical optics,biotechnology,Cherenkov-excited luminescence scanned imaging,image reconstruction techniques,alternating direction method of multipliers,deep learning,unrolled optimization
AI 理解论文
溯源树
样例
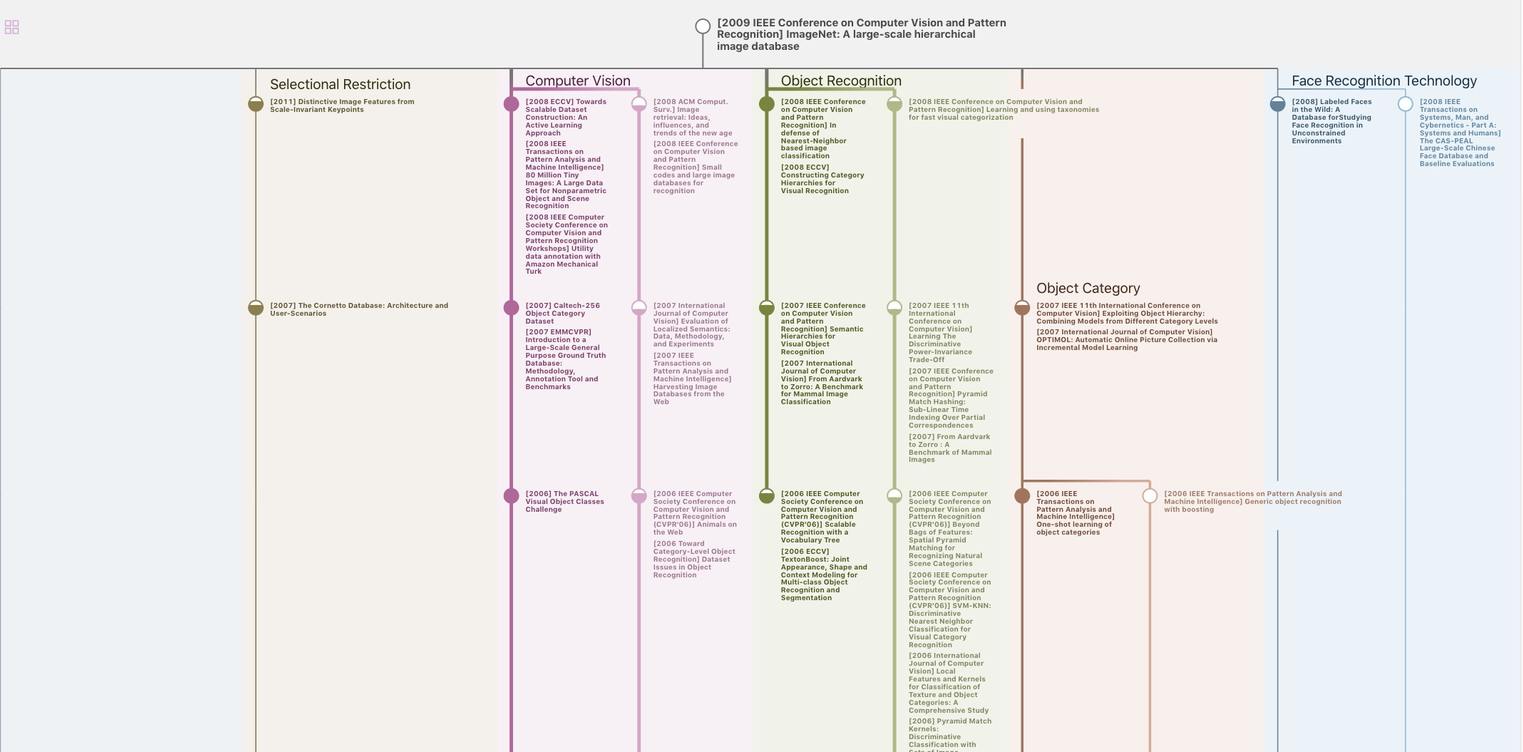
生成溯源树,研究论文发展脉络
Chat Paper
正在生成论文摘要