Multisensor data fusion and machine learning to classify wood products and predict workpiece characteristics during milling
CIRP Journal of Manufacturing Science and Technology(2023)
摘要
The wood industry demands advanced methods for material classification and workpiece characteristic modelling to enhance process monitoring and adaptive process control. This paper presents a sensor fusion approach that integrates data from acoustic emissions, airborne sound, and power consumption during the milling of solid wood and wood-based composites. The aims are to achieve accurate material classification and to model workpiece characteristics such as surface roughness or density. A design matrix was generated by extracting relevant features from the multimodal signals to serve as an input for the classification and regression algorithms. The tested classification approaches to differentiate between workpiece type demonstrated high precision with an average validation accuracy of 92.16 %. Regression models for predicting the surface roughness showed R2 values between 0.79 and 0.97. The density could be predicted with R2 values between 0.84 and 0.98. As a conclusion, workpiece types could be classified and important workpiece properties during machining, such as surface roughness and density, could be well described by using information from multiple sensors during machining.
更多查看译文
关键词
Machining, Process monitoring, Sensor fusion, Machine learning, Predictive models
AI 理解论文
溯源树
样例
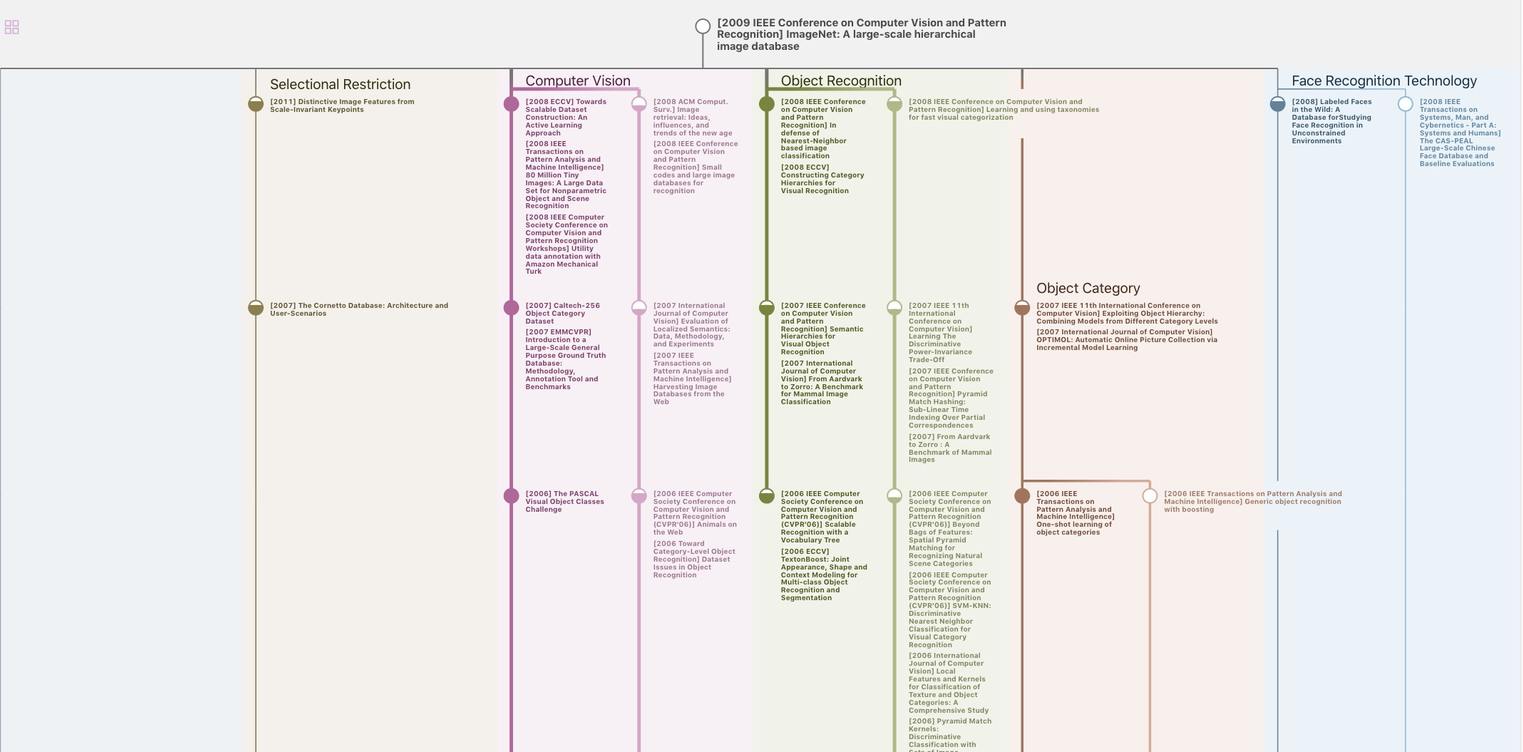
生成溯源树,研究论文发展脉络
Chat Paper
正在生成论文摘要