Predicting a Protein's Stability under a Million Mutations
NeurIPS(2023)
摘要
Stabilizing proteins is a foundational step in protein engineering. However, the evolutionary pressure of all extant proteins makes identifying the scarce number of mutations that will improve thermodynamic stability challenging. Deep learning has recently emerged as a powerful tool for identifying promising mutations. Existing approaches, however, are computationally expensive, as the number of model inferences scales with the number of mutations queried. Our main contribution is a simple, parallel decoding algorithm. Our Mutate Everything is capable of predicting the effect of all single and double mutations in one forward pass. It is even versatile enough to predict higher-order mutations with minimal computational overhead. We build Mutate Everything on top of ESM2 and AlphaFold, neither of which were trained to predict thermodynamic stability. We trained on the Mega-Scale cDNA proteolysis dataset and achieved state-of-the-art performance on single and higher-order mutations on S669, ProTherm, and ProteinGym datasets. Code is available at https://github.com/jozhang97/MutateEverything
更多查看译文
关键词
mutations,protein,stability
AI 理解论文
溯源树
样例
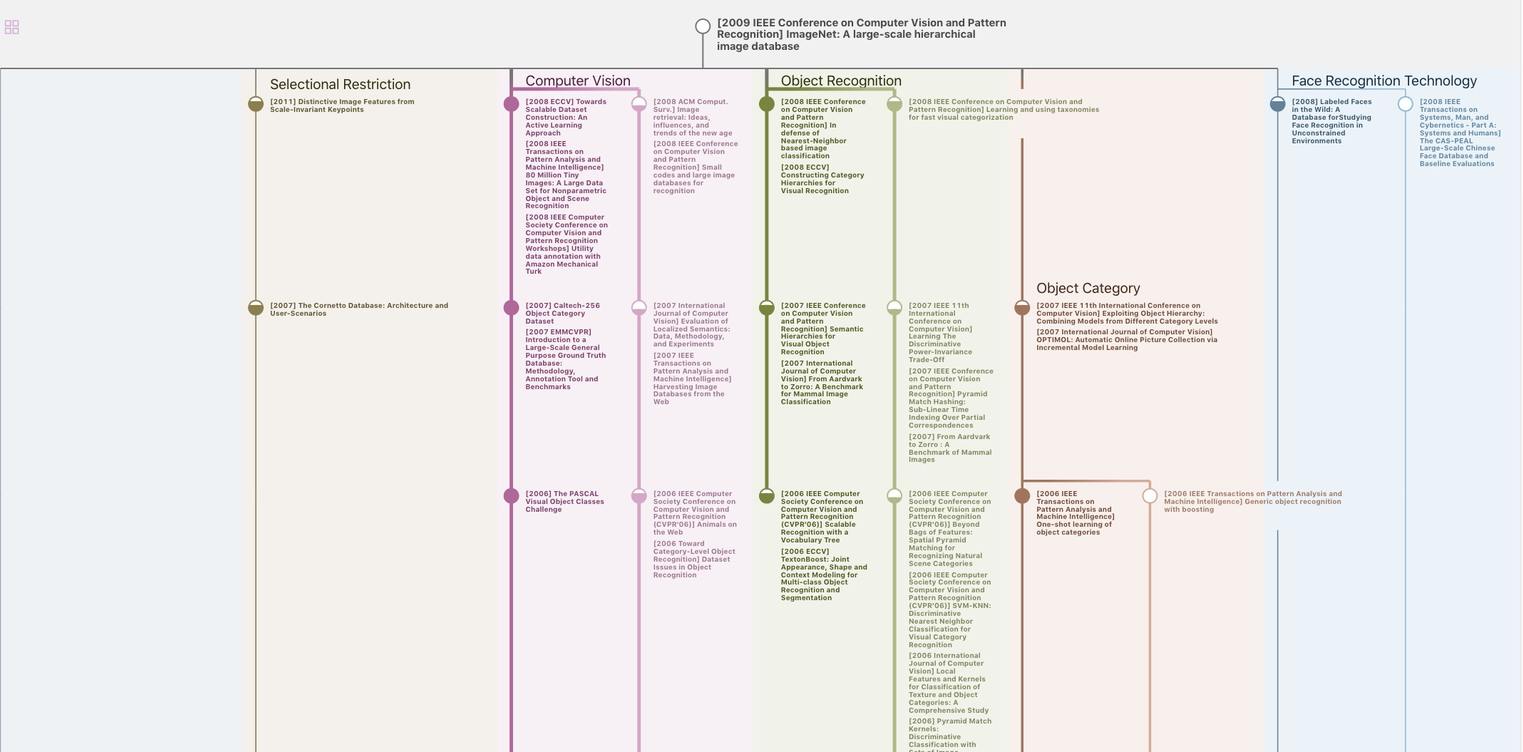
生成溯源树,研究论文发展脉络
Chat Paper
正在生成论文摘要