Correcting phenomenological quantum noise via belief propagation
CoRR(2023)
摘要
Quantum stabilizer codes often face the challenge of syndrome errors due to error-prone measurements. To address this issue, multiple rounds of syndrome extraction are typically employed to obtain reliable error syndromes. In this paper, we consider phenomenological decoding problems, where data qubit errors may occur between two syndrome extractions, and each syndrome measurement can be faulty. To handle these diverse error sources, we define a generalized check matrix over mixed quaternary and binary alphabets to characterize their error syndromes. This generalized check matrix leads to the creation of a Tanner graph comprising quaternary and binary variable nodes, which facilitates the development of belief propagation (BP) decoding algorithms to tackle phenomenological errors. Importantly, our BP decoders are applicable to general sparse quantum codes. Through simulations of quantum memory protected by rotated toric codes, we demonstrates an error threshold of 3.3% in the phenomenological noise model. Additionally, we propose a method to construct effective redundant stabilizer checks for single-shot error correction. Simulations show that BP decoding performs exceptionally well, even when the syndrome error rate greatly exceeds the data error rate.
更多查看译文
关键词
phenomenological quantum noise,quantum noise,belief
AI 理解论文
溯源树
样例
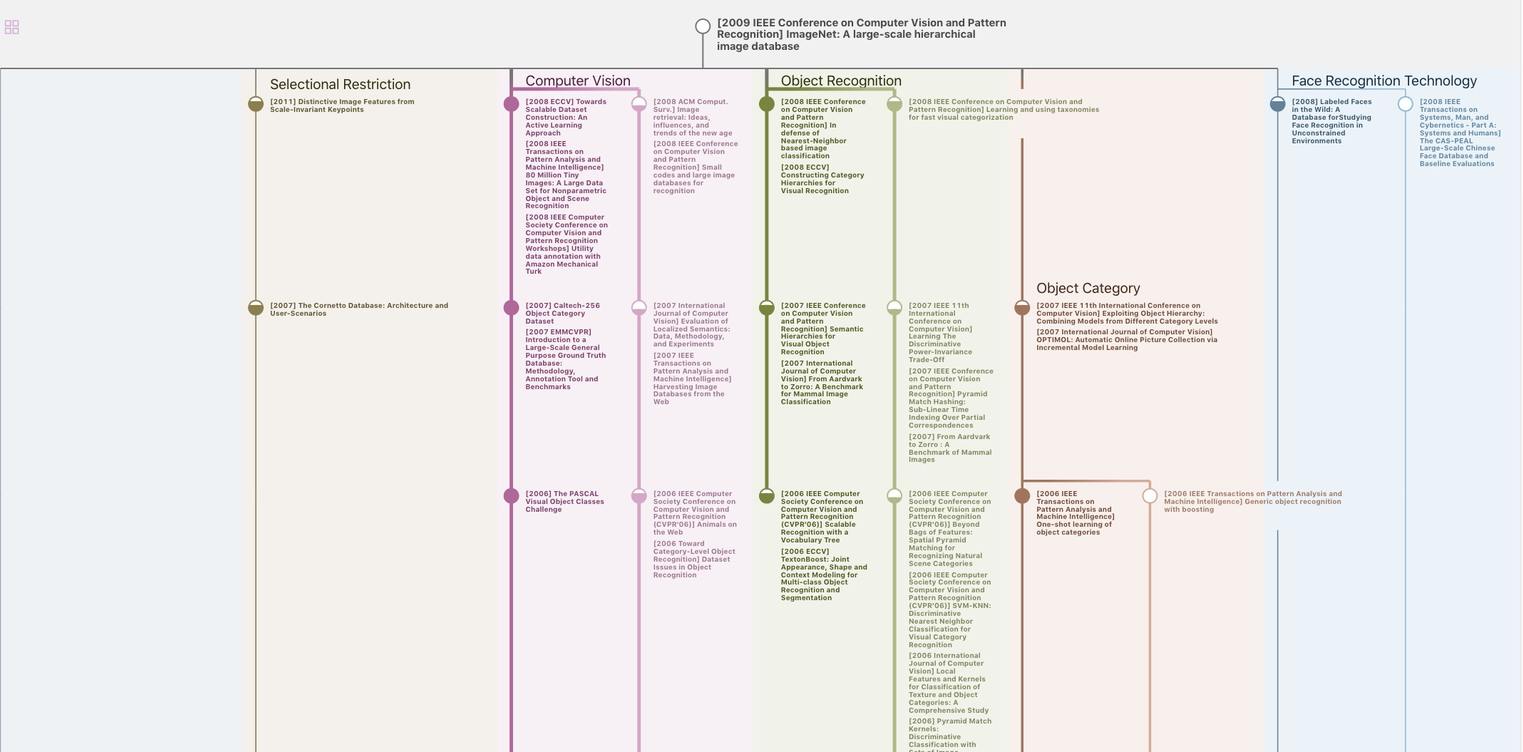
生成溯源树,研究论文发展脉络
Chat Paper
正在生成论文摘要