Explanatory Object Part Aggregation for Zero-Shot Learning
IEEE TRANSACTIONS ON PATTERN ANALYSIS AND MACHINE INTELLIGENCE(2024)
摘要
Zero-shot learning (ZSL) aims to recognize objects from unseen classes only based on labeled images from seen classes. Most existing ZSL methods focus on optimizing feature spaces or generating visual features of unseen classes, both in conventional ZSL and generalized zero-shot learning (GZSL). However, since the learned feature spaces are suboptimal, there exists many virtual connections where visual features and semantic attributes are not corresponding to each other. To reduce virtual connections, in this paper, we propose to discover comprehensive and fine-grained object parts by building explanatory graphs based on convolutional feature maps, then aggregate object parts to train a part-net to obtain prediction results. Since the aggregated object parts contain comprehensive visual features for activating semantic attributes, the virtual connections can be reduced by a large extent. Since part-net aims to extract local fine-grained visual features, some attributes related to global structures are ignored. To take advantage of both local and global visual features, we design a feature distiller to distill local features into a master-net which aims to extract global features. The experimental results on AWA2, CUB, FLO, and SUN dataset demonstrate that our proposed method obviously outperforms the state-of-the-arts in both conventional ZSL and GZSL tasks.
更多查看译文
关键词
Zero-shot learning,generalized zero-shot learning,virtual connections,object part discovery,explanatory graph,part aggregation,feature distillation
AI 理解论文
溯源树
样例
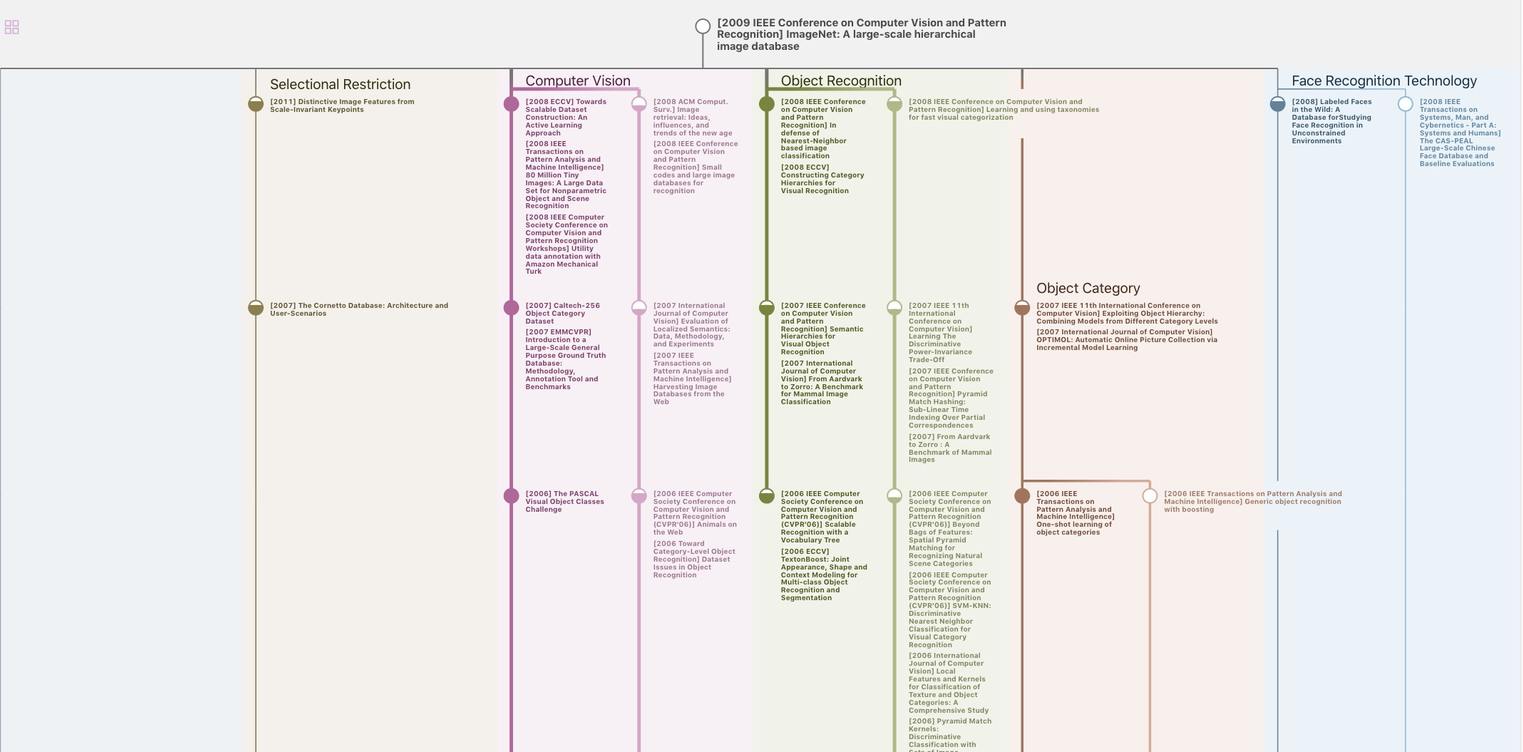
生成溯源树,研究论文发展脉络
Chat Paper
正在生成论文摘要