A Deep Learning-Based IDS for Automotive Theft Detection for In-Vehicle CAN Bus
IEEE Access(2023)
摘要
Driver behavior features extracted from the controller area network (CAN) have potential applications in improving vehicle safety. However, the development of a classifier-based intrusion detection system (IDS) for in-vehicle networks remains an open research problem. To address this challenge, we incorporate novel $n$ -fold cross-validation windowing techniques on two publicly available driving behavior datasets. A driver classification-based IDS is proposed using the LSTM-FCN model that utilizes the strengths of both fully convolutional network (FCN) and long short-term memory (LSTM) networks. These modules allow the model to learn spatial and temporal features and utilize contextual information. In addition, we combine three squeeze and excite (SnE) layers following FCN layers to incorporate adjacent spatial locations and augment a scaled dot product attention mechanism into the LSTM to improve its feature selection and extraction capabilities. Our proposed IDS uses hacking and countermeasure research lab (HCRL) and test datasets, which achieve an improvement in accuracy of 4.18% and 13.99% respectively, from the baseline LSTM-FCN model. The experimental results of our method exhibited an overall accuracy of 99.36% and 96.36% for both datasets and outperformed various state-of-the-art methods.
更多查看译文
关键词
automotive theft detection,can bus,ids,learning-based,in-vehicle
AI 理解论文
溯源树
样例
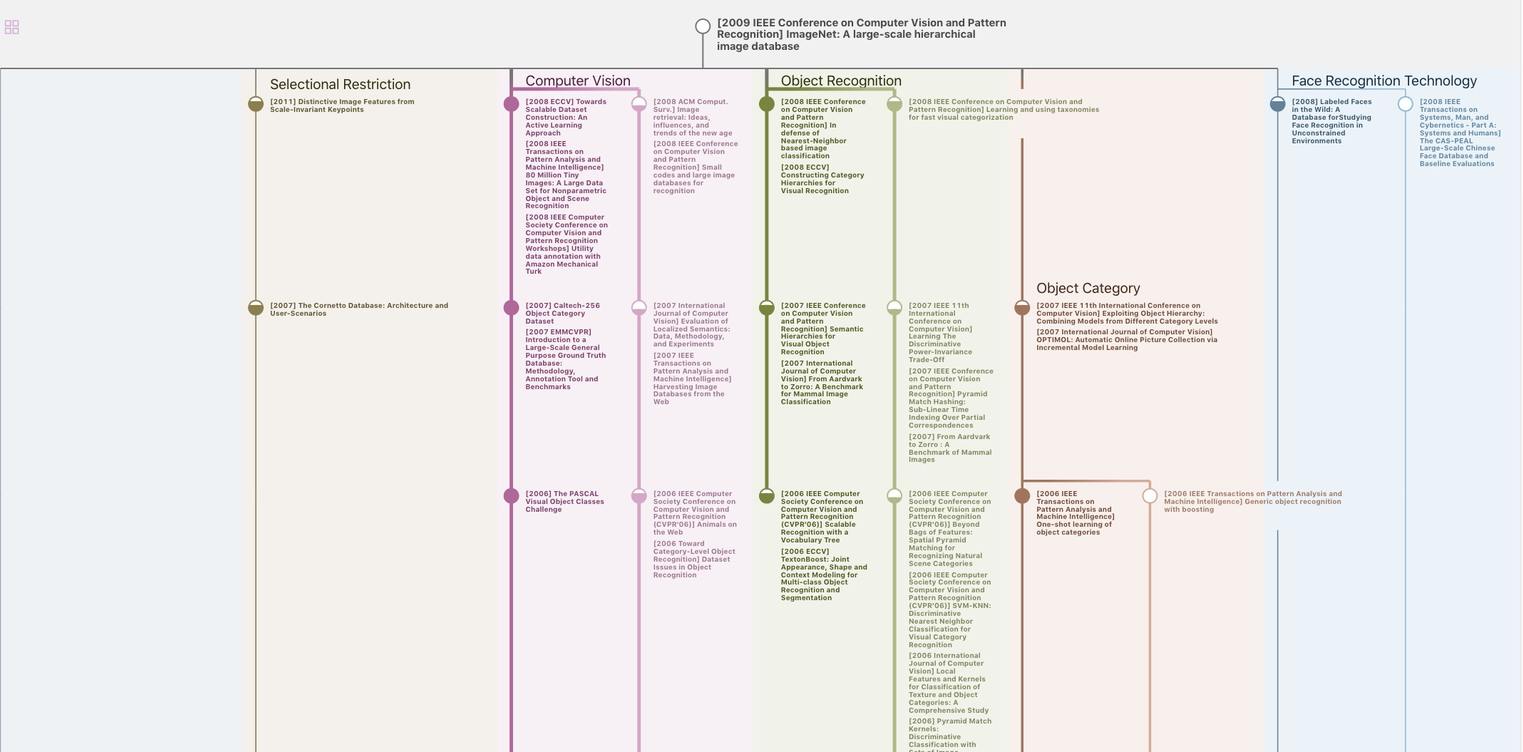
生成溯源树,研究论文发展脉络
Chat Paper
正在生成论文摘要