ASAR Dataset and Computational Model for Affective State Recognition During ARAT Assessment for Upper Extremity Stroke Survivors
ICMI '23 Companion: Companion Publication of the 25th International Conference on Multimodal Interaction(2023)
摘要
Stroke is one of the leading global causes of disability and many stroke survivors must deal with challenging movement impairment daily. Stroke rehabilitation therapy is expensive, physically, and emotionally challenging, can be difficult to access, and is time-consuming for patients and clinicians alike. As a result, there is an opportunity to develop automated or semi-automated systems to assist clinicians in efficient and accurate stroke patient movement assessment. In this work, we present the ASAR (Affective State for ARAT Rehab) dataset where we use custom label classes (neutral, engagement, and pain) to annotate video data of stroke survivors performing the standardized Action Research Arm Test (ARAT) for upper extremity assessment. 106 patients participated in this study and each patient performed, or attempted to perform, each of the 19 ARAT assessment tasks. In our preliminary analysis, we annotated the frames of 126 videos for 10 patients. Using a multimodal model, we achieved an accuracy of 0.77 ± 0.06 in per-frame state recognition on the ASAR dataset. This affective information will potentially increase the interpretability of clinician’s scores of the stroke survivor’s ARAT performance and thus could assist in the design and implementation of semi-automated systems for stroke assessment and rehabilitation.
更多查看译文
AI 理解论文
溯源树
样例
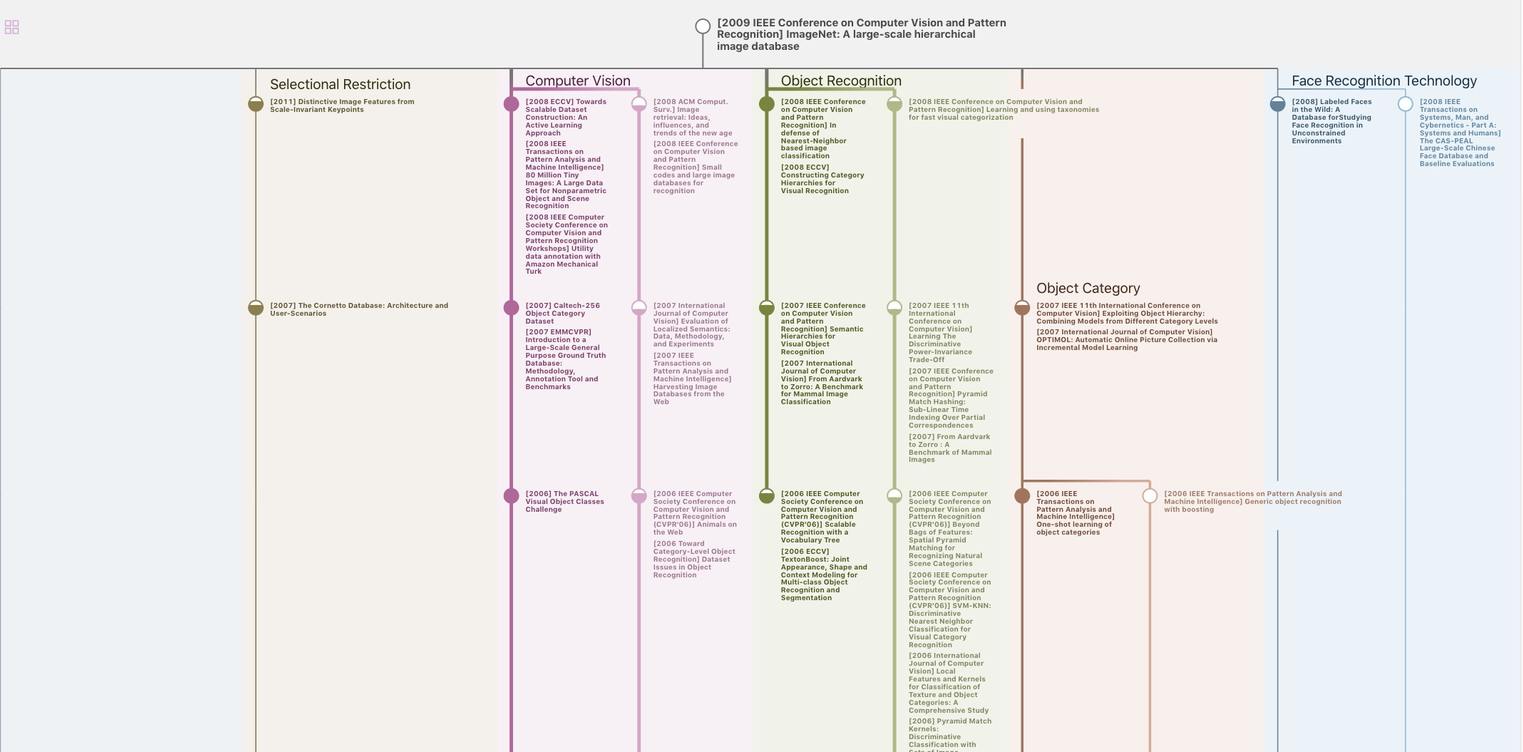
生成溯源树,研究论文发展脉络
Chat Paper
正在生成论文摘要